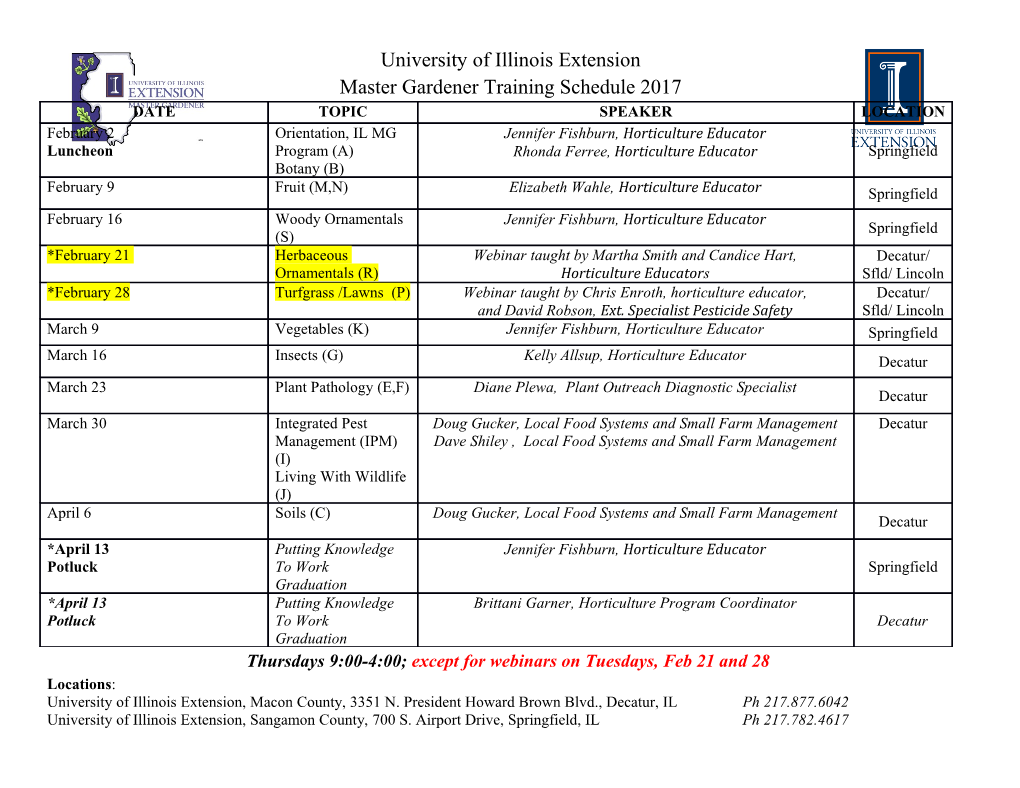
CHAPTER MARKOV RENEWAL THEORY APPLIED TO PERFORMABILITY EVALUATION y Ricardo Fricks Miklos Telek Center for Adv Comp and Comm Dept of Telecommunications Dept of Electrical Engineering Technical University of Budap est Duke University Budap est Hungary Durham NC y Antonio Puliato Kishor Trivedi Ist di Informatica e Telecom Center for Adv Comp and Comm Universita di Catania Dept of Electrical Engineering Catania Italy Duke University Durham NC Abstract Signicant advances have been made in performability modeling and analysis since the early s In this chapter we present two special classes of continuous time stochastic processes with embedded Markov renewal sequences that can besuccessful ly employed for performability analysis Detailed examples il lustrate the solution techniques surveyed in the introductory sections of the chapter y This work was supp orted in part by an NSF grant EEC Brazils Na tional Council of Research and Development and a CACC core pro ject funded by NASA Lewis Research Center FRICKS TELEK PULIAFITO TRIVEDI INTRODUCTION Computer and communication systems are designed to meet a certain sp ecied b ehavior The pro curement of metrics to establish howwell the system b ehaves that is how closely it follows the sp ecied b ehav ior is the ob jective of quantitative analysis Traditionally p erformance and dep endabilityevaluation are used as separate approaches to pro vide quantitative gures of system b ehavior Performance evaluates the quality of service assuming that the system is failurefree De p endability fo cuses on determining deviation of the actual b ehavior from the sp ecied b ehavior in the presence of comp onent or subsystem failures Beaudry prop osed the aggregated measure computation before failure while Meyer prop osed the term performabilitywhich has b een used since then Performability analysis aims to capture the interaction between the failurerepair b ehavior and the p erformance delivered by the system Its results are fundamental to the analysis of realtime system p erformance in the presence of failure Performability measures provide b etter insightinto the b ehavior of faulttolerant systems Basic metrics used to evaluate faulttolerant designs are reliabilityandavailability The conditional probabilitythat a system survives until some time tgiven it is fully op erational at t is called the reliability Rt of the system Reliability is used to describ e systems which are not allowed to fail in which the system is serving a critical function and cannot b e down Note that comp onents or subsystems can fail so long as the system do es not The instanta neous availability At of a system is the probability that the system is prop erly functioning at time t Availabilityistypically used as a basis for evaluating systems in which functionality can b e delayed or denied for short p erio ds without serious consequences Reliability and availability do not consider dierentlevels of system functionality Performability analysis of real systems with nondeterministic comp onents andor environmental characteristics results in sto chastic mo deling problems Several techniques for solving them for transient and steadystate measures have b een prop osed and later combined un der the framework of Markov reward mo dels The traditional frame work allows the solution of sto chastic problems enjoying the Markov prop erty the probability of any particular futurebehavior of the pro cess when its current state is known exactly is not altered by additional MARKOVRENEWAL THEORY know ledge concerning its past behavior If the past history of the pro cess is completely summarized in the current state and is indep endent of the current time then the pro cess is said to b e time homoge neous Otherwise the exact characterization of the present state needs the asso ciated time information and the pro cess is said to b e non homogeneous A wide range of real problems fall in the class of Markov mo dels b oth homogeneous and nonhomogeneous but problems in p erformability analysis havebeenidentied that cannot b e adequately describ ed in this traditional framework The common characteristic these problems share is that the Markovproperty is not valid if valid at all at all time instants This category of problems is jointly re ferred to as nonMarkovian mo dels and can b e analyzed using several approaches Phasetypeexpansions when the past history of the sto chas tic pro cess can b e describ ed by a discrete variable an expanded continuoustime homogeneous Markovchain can b e used to cap ture the sto chastic b ehavior of the original system Supplementary variables when the past history is describ ed by one or more continuous variables the approach of the supple mentary variables can b e applied and a set of ordinary or partial dierential equations can b e dened together with b oundary con ditions and analyzed Embeddedpointprocesses when the temp oral b ehavior of the system can b e studied by means of some appropriately chosen embedded epochs where the Markov prop erty applies Several wellknown classes of sto chastic pro cesses such as regenerative semiMarkov and Markov regenerative pro cesses are based on the concept of emb edded p oints Theobjectofthischapter is to present a theory based on the concept of emb edded p ointpro cesses that encompass semiMarkov and Markov regenerative pro cesses This theory named Markov renewal theoryis reviewed in the rst three sections of this chapter and later applied to several nonMarkovian p erformability mo dels Our purp ose is to provide an uptodate treatment of the basic analytic mo dels to study nonMarkovian systems by means of Markov renewal theory and an accurate description of the solution algorithms In particular we develop a general framework which allows us to deal FRICKS TELEK PULIAFITO TRIVEDI with renewal pro cesses and sp ecically with semiMarkovandMarkov regenerative pro cesses Wehopethatthischapter will serve as a refer ence for practicing engineers researchers and students in p erformance and reliability mo deling Other surveys on Markov renewal theory ap plied to reliability analysis have app eared in the literature but none of them as complete or as didactic as the presentone The rest of this chapter is organized as follows Section intro duces the basic terminology asso ciated with the theory including the concepts and distinction b etween semiMarkov pro cesses and Markov regenerative pro cesses Section presents basic solution techniques for sto chastic pro cesses with emb edded Markov renewal sequences Markov regenerativePetri nets useful as a highlevel description language of these kind of sto chastic mo dels are reviewed in Section and employed in the analyses of three examples presented in Section Examples are selected to illustrate the metho dology asso ciated with semiMarkov and Markov regenerative pro cesses Section concludes the chapter MARKOV RENEWAL THEORY Assume we wish to quantitatively study the b ehavior of a given non deterministic system One p ossible solution would b e to asso ciate a random variable Z taking values in a countable set F to describ e t the state of the system at any time instant t The family of random variables Z constitutes a sto chastic pro cess Z fZ t R g t t successive occurrences of a recurrent phenomenon time S = 0 S S 0 1 S2 3 θ θ θ 1 2 3 start of observation Figure A sample realization of a renewal pro cess Supp ose we are interested in a single event related with the system eg when system comp onents fail Additionally assume MARKOVRENEWAL THEORY the times b etween successive o ccurrences of this typeofeventare independent and identical ly distributed iid random variables Let S S S b e the time instants of successiveevents to o c cur as shown in Figure The sequence of nonnegative iid random variables S fS S n N g is a renewal process n n Otherwise if we do not start observing the system at the exact moment an event has o ccurred ie S the sto chastic pro cess S is a delayed renewal process Contexts in which renewal pro cesses arise ab ound in applied probabilityFor instance the times b etween successive electrical im pulses or signals impinging on a recording device are often assumed to form a renewal pro cess Another classical example of renewal pro cess is the item replacement problem explored in where S S S S represent the lifetimes of items light bulbs machines etc that are successively placed in service immediately following the failure of the previous one However supp ose instead of a single event we observe that cer tain transitions b etween identiable system states j of a subset E of F EF also resemble the b ehavior just describ ed when considered in isolation Successive times S at which a xed state j j Eisentered n form a p ossibly delayed renewal pro cess In the sample pro cess real ization depicted in Figure we see that the sequence of time instants fS S g forms a renewal pro cess while fS S g and fS S g form delayed renewal pro cesses Additionally when studying the system evolution weobserve that at these particular times the sto chastic pro cess Z exhibits the Markov prop erty ie at anygiven moment S n Nwe can for n get the past history of the pro cess In this scenario we are dealing with a countable collection of renewal pro cesses progressing simultane ously such that successive renewals form a discretetime Markovchain DTMC The sup erp osition of all the identied renewal pro cesses gives the p oints fS n Ngknown as Markov renewal moments and to n gether
Details
-
File Typepdf
-
Upload Time-
-
Content LanguagesEnglish
-
Upload UserAnonymous/Not logged-in
-
File Pages44 Page
-
File Size-