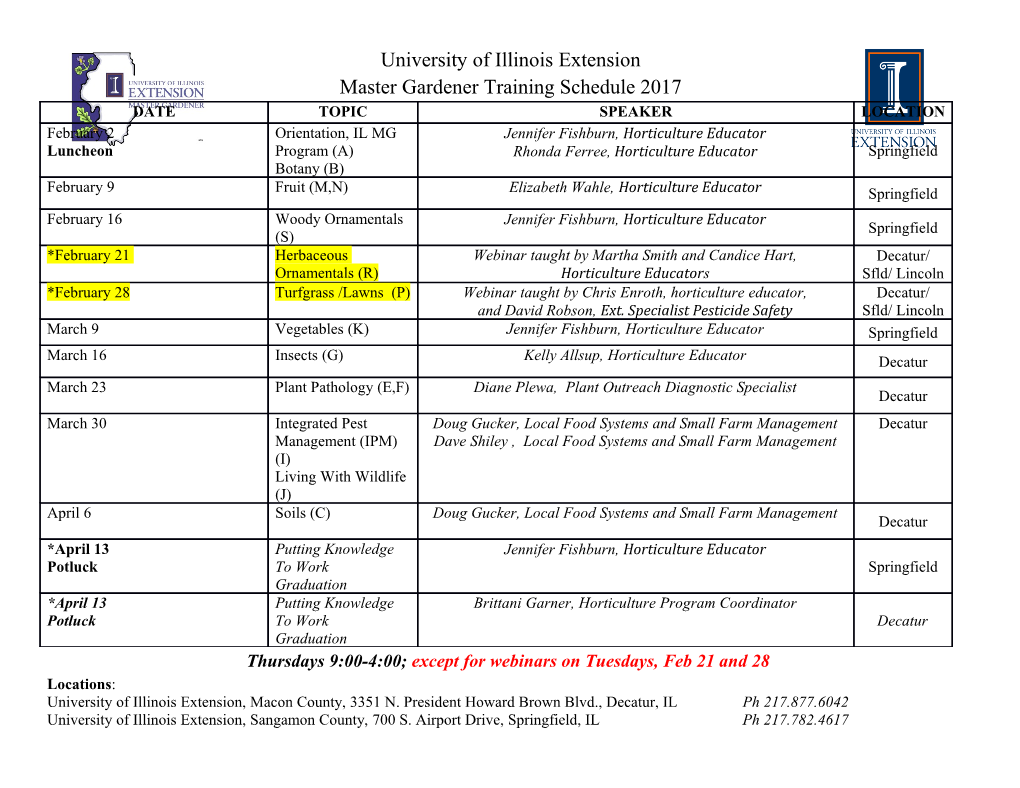
Named Entity Recognition in Tweets: An Experimental Study Alan Ritter, Sam Clark, Mausam and Oren Etzioni Computer Science and Engineering University of Washington Seattle, WA 98125, USA aritter,ssclark,mausam,etzioni @cs.washington.edu { } Abstract the size of the Library of Congress (Hachman, 2011) and is growing far more rapidly. Due to the vol- People tweet more than 100 Million times ume of tweets, it is natural to consider named-entity daily, yielding a noisy, informal, but some- recognition, information extraction, and text mining times informative corpus of 140-character over tweets. Not surprisingly, the performance of messages that mirrors the zeitgeist in an un- precedented manner. The performance of “off the shelf” NLP tools, which were trained on standard NLP tools is severely degraded on news corpora, is weak on tweet corpora. tweets. This paper addresses this issue by In response, we report on a re-trained “NLP re-building the NLP pipeline beginning with pipeline” that leverages previously-tagged out-of- part-of-speech tagging, through chunking, to domain text, 2 tagged tweets, and unlabeled tweets named-entity recognition. Our novel T-NER to achieve more effective part-of-speech tagging, system doubles F1 score compared with the chunking, and named-entity recognition. Stanford NER system. T-NER leverages the redundancy inherent in tweets to achieve this 1 The Hobbit has FINALLY started filming! I performance, using LabeledLDA to exploit cannot wait! Freebase dictionaries as a source of distant 2 Yess! Yess! Its official Nintendo announced supervision. LabeledLDA outperforms co- today that they Will release the Nintendo 3DS training, increasing F1 by 25% over ten com- in north America march 27 for $250 mon entity types. 3 Government confirms blast n nuclear plants n Our NLP tools are available at: http:// japan...don’t knw wht s gona happen nw... github.com/aritter/twitter_nlp Table 1: Examples of noisy text in tweets. 1 Introduction We find that classifying named entities in tweets is a difficult task for two reasons. First, tweets contain Status Messages posted on Social Media websites a plethora of distinctive named entity types (Compa- such as Facebook and Twitter present a new and nies, Products, Bands, Movies, and more). Almost challenging style of text for language technology all these types (except for People and Locations) are due to their noisy and informal nature. Like SMS relatively infrequent, so even a large sample of man- (Kobus et al., 2008), tweets are particularly terse ually annotated tweets will contain few training ex- and difficult (See Table 1). Yet tweets provide a amples. Secondly, due to Twitter’s 140 character unique compilation of information that is more up- limit, tweets often lack sufficient context to deter- to-date and inclusive than news articles, due to the mine an entity’s type without the aid of background low-barrier to tweeting, and the proliferation of mo- 2 bile devices.1 The corpus of tweets already exceeds Although tweets can be written on any subject, following convention we use the term “domain” to include text styles or 1See the “trending topics” displayed on twitter.com genres such as Twitter, News or IRC Chat. 1524 Proceedings of the 2011 Conference on Empirical Methods in Natural Language Processing, pages 1524–1534, Edinburgh, Scotland, UK, July 27–31, 2011. c 2011 Association for Computational Linguistics knowledge. Accuracy Error To address these issues we propose a distantly su- Reduction pervised approach which applies LabeledLDA (Ra- Majority Baseline (NN) 0.189 - Word’s Most Frequent Tag 0.760 - mage et al., 2009) to leverage large amounts of unla- Stanford POS Tagger 0.801 - beled data in addition to large dictionaries of entities T-POS(PTB) 0.813 6% gathered from Freebase, and combines information T-POS(Twitter) 0.853 26% about an entity’s context across its mentions. T-POS(IRC + PTB) 0.869 34% We make the following contributions: T-POS(IRC + Twitter) 0.870 35% T-POS(PTB + Twitter) 0.873 36% 1. We experimentally evaluate the performance of T-POS(PTB + IRC + Twitter) 0.883 41% off-the-shelf news trained NLP tools when ap- plied to Twitter. For example POS tagging Table 2: POS tagging performance on tweets. By training on in-domain labeled data, in addition to annotated IRC accuracy drops from about 0.97 on news to chat data, we obtain a 41% reduction in error over the 0.80 on tweets. By utilizing in-domain, out- Stanford POS tagger. of-domain, and unlabeled data we are able to substantially boost performance, for example obtaining a 52% increase in F1 score on seg- 2, 4 and 5) represent 4-fold cross-validation experi- menting named entities. ments on the respective tasks.3 2. We introduce a novel approach to distant super- 2.1 Part of Speech Tagging vision (Mintz et al., 2009) using Topic Models. Part of speech tagging is applicable to a wide range LabeledLDA is applied, utilizing constraints of NLP tasks including named entity segmentation based on an open-domain database (Freebase) and information extraction. as a source of supervision. This approach in- Prior experiments have suggested that POS tag- creases F score by 25% relative to co-training 1 ging has a very strong baseline: assign each word (Blum and Mitchell, 1998; Yarowsky, 1995) on to its most frequent tag and assign each Out of Vo- the task of classifying named entities in Tweets. cabulary (OOV) word the most common POS tag. This baseline obtained a 0.9 accuracy on the Brown The rest of the paper is organized as follows. corpus (Charniak et al., 1993). However, the appli- We successively build the NLP pipeline for Twitter cation of a similar baseline on tweets (see Table 2) feeds in Sections 2 and 3. We first present our ap- obtains a much weaker 0.76, exposing the challeng- proaches to shallow syntax – part of speech tagging ing nature of Twitter data. ( 2.1), and shallow parsing ( 2.2). 2.3 describes a § § § novel classifier that predicts the informativeness of A key reason for this drop in accuracy is that Twit- capitalization in a tweet. All tools in 2 are used ter contains far more OOV words than grammatical § as features for named entity segmentation in 3.1. text. Many of these OOV words come from spelling § Next, we present our algorithms and evaluation for variation, e.g., the use of the word “n” for “in” in Ta- entity classification ( 3.2). We describe related work ble 1 example 3. Although NNP is the most frequent § in 4 and conclude in 5. tag for OOV words, only about 1/3 are NNPs. § § The performance of off-the-shelf news-trained 2 Shallow Syntax in Tweets POS taggers also suffers on Twitter data. The state- of-the-art Stanford POS tagger (Toutanova et al., We first study two fundamental NLP tasks – POS 2003) improves on the baseline, obtaining an accu- tagging and noun-phrase chunking. We also discuss racy of 0.8. This performance is impressive given a novel capitalization classifier in 2.3. The outputs § that its training data, the Penn Treebank WSJ (PTB), of all these classifiers are used in feature generation is so different in style from Twitter, however it is a for named entity recognition in the next section. huge drop from the 97% accuracy reported on the For all experiments in this section we use a dataset of 800 randomly sampled tweets. All results (Tables 3We used Brendan O’Connor’s Twitter tokenizer 1525 Gold Predicted Stanford T-POS Error Error ample, following are lexical variations on the word Error Reduction “tomorrow” from one cluster after filtering out other NN NNP 0.102 0.072 29% words (most of which refer to days): UH NN 0.387 0.047 88% VB NN 0.071 0.032 55% ‘2m’, ‘2ma’, ‘2mar’, ‘2mara’, ‘2maro’, NNP NN 0.130 0.125 4% ‘2marrow’, ‘2mor’, ‘2mora’, ‘2moro’, ‘2mo- UH NNP 0.200 0.036 82% row’, ‘2morr’, ‘2morro’, ‘2morrow’, ‘2moz’, ‘2mr’, ‘2mro’, ‘2mrrw’, ‘2mrw’, ‘2mw’, Table 3: Most common errors made by the Stanford POS ‘tmmrw’, ‘tmo’, ‘tmoro’, ‘tmorrow’, ‘tmoz’, Tagger on tweets. For each case we list the fraction of ‘tmr’, ‘tmro’, ‘tmrow’, ‘tmrrow’, ‘tm- times the gold tag is misclassified as the predicted for rrw’, ‘tmrw’, ‘tmrww’, ‘tmw’, ‘tomaro’, both our system and the Stanford POS tagger. All verbs ‘tomarow’, ‘tomarro’, ‘tomarrow’, ‘tomm’, are collapsed into VB for compactness. ‘tommarow’, ‘tommarrow’, ‘tommoro’, ‘tom- morow’, ‘tommorrow’, ‘tommorw’, ‘tomm- row’, ‘tomo’, ‘tomolo’, ‘tomoro’, ‘tomorow’, PTB. There are several reasons for this drop in per- ‘tomorro’, ‘tomorrw’, ‘tomoz’, ‘tomrw’, formance. Table 3 lists common errors made by ‘tomz’ the Stanford tagger. First, due to unreliable capi- 5 talization, common nouns are often misclassified as T-POS uses Conditional Random Fields (Laf- proper nouns, and vice versa. Also, interjections ferty et al., 2001), both because of their ability to and verbs are frequently misclassified as nouns. In model strong dependencies between adjacent POS addition to differences in vocabulary, the grammar tags, and also to make use of highly correlated fea- of tweets is quite different from edited news text. tures (for example a word’s identity in addition to For instance, tweets often start with a verb (where prefixes and suffixes). Besides employing the Brown the subject ‘I’ is implied), as in: “watchng american clusters computed above, we use a fairly standard set dad.” of features that include POS dictionaries, spelling and contextual features. To overcome these differences in style and vocab- On a 4-fold cross validation over 800 tweets, ulary, we manually annotated a set of 800 tweets T-POS outperforms the Stanford tagger, obtaining a (16K tokens) with tags from the Penn TreeBank tag 26% reduction in error.
Details
-
File Typepdf
-
Upload Time-
-
Content LanguagesEnglish
-
Upload UserAnonymous/Not logged-in
-
File Pages11 Page
-
File Size-