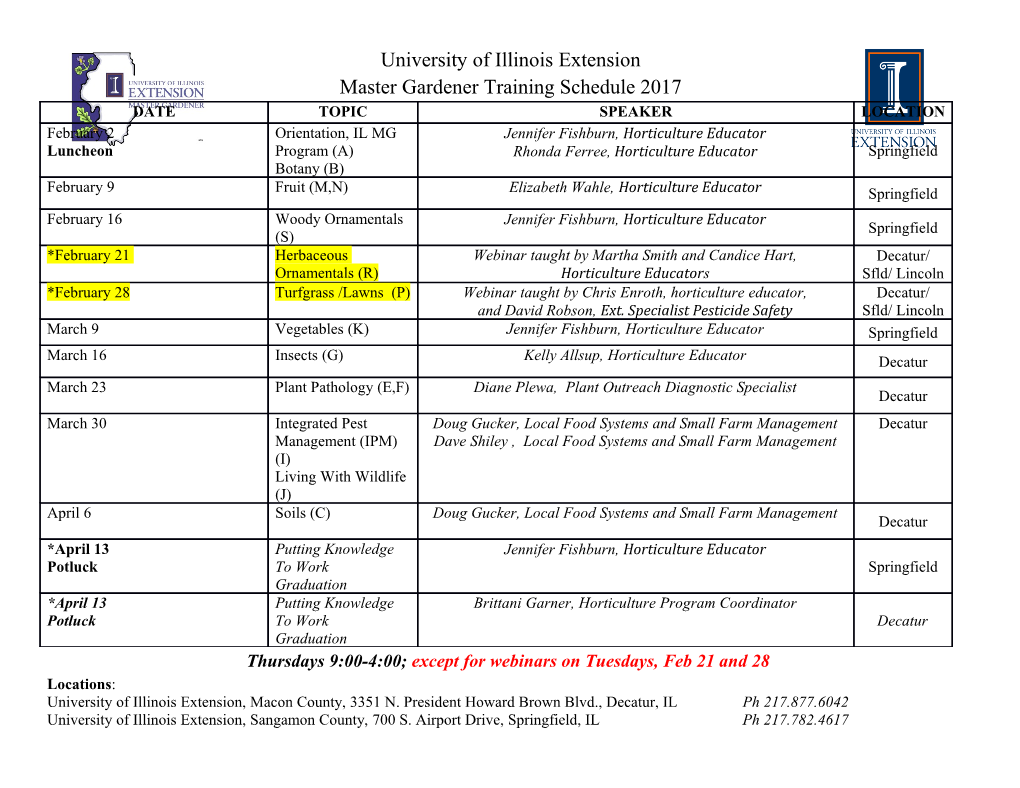
Classical Planning in Latent Space: From Unlabeled Images to PDDL (and back) Masataro Asai, Alex Fukunaga Graduate School of Arts and Sciences University of Tokyo Abstract Current domain-independent, classical planners re- ? quire symbolic models of the problem domain and Initial state Goal state image Original instance as input, resulting in a knowledge acquisi- image (black/white) Mandrill image tion bottleneck. Meanwhile, although recent work in deep learning has achieved impressive results in Figure 1: An image-based 8-puzzle. many fields, the knowledge is encoded in a subsym- bolic representation which cannot be directly used [ ] by symbolic systems such as planners. We propose Mnih et al., 2015; Graves et al., 2016 . However, the current LatPlan, an integrated architecture combining deep state-of-the-art in pure NN-based systems do not yet provide learning and a classical planner. Given a set of un- guarantees provided by symbolic planning systems, such as labeled training image pairs showing allowed ac- deterministic completeness and solution optimality. tions in the problem domain, and a pair of images Using a NN-based perceptual system to automatically pro- representing the start and goal states, LatPlan uses vide input models for domain-independent planners could a Variational Autoencoder to generate a discrete greatly expand the applicability of planning technology and latent vector from the images, based on which a offer the benefits of both paradigms. We consider the problem PDDL model can be constructed and then solved of robustly, automatically bridging the gap between such sub- by an off-the-shelf planner. We evaluate LatPlan us- symbolic representations and the symbolic representations ing image-based versions of 3 planning domains: required by domain-independent planners. 8-puzzle, LightsOut, and Towers of Hanoi. Fig. 1 (left) shows a scrambled, 3x3 tiled version of the the photograph on the right, i.e., an image-based instance of the 8-puzzle. Even for humans, this photograph-based task is ar- 1 Introduction guably more difficult to solve than the standard 8-puzzle be- Recent advances in domain-independent planning have cause of the distracting visual aspects. We seek a domain- greatly enhanced their capabilities. However, planning prob- independent system which, given only a set of unlabeled lems need to be provided to the planner in a structured, sym- images showing the valid moves for this image-based puz- bolic representation such as PDDL [McDermott, 2000], and zle, finds an optimal solution to the puzzle. Although the 8- in general, such symbolic models need to be provided by a hu- puzzle is trivial for symbolic planners, solving this image- man, either directly in a modeling language such as PDDL, or based problem with a domain-independent system which (1) via a compiler which transforms some other symbolic prob- has no prior assumptions/knowledge (e.g., “sliding objects”, lem representation into PDDL. This results in the knowledge- “tile arrangement”), and (2) must acquire all knowledge from acquisition bottleneck, where the modeling step is sometimes the images, is nontrivial. Such a system should not make as- the bottleneck in the problem solving cycle. In addition, the sumptions about the image (e.g., “a grid-like structure”). The requirement for symbolic input poses a significant obstacle to only assumption allowed about the nature of the task is that it applying planning in new, unforeseen situations where no hu- can be modeled and solved as a classical planning problem. man is available to create such a model or a generator, e.g., We propose Latent-space Planner (LatPlan), an integrated autonomous spacecraft exploration. In particular this first re- architecture which uses NN-based image processing to com- quires generating symbols from raw sensor input, i.e., the pletely automatically generate a propositional, symbolic symbol grounding problem [Steels, 2008]. problem representation. LatPlan consists of 3 components: Recently, significant advances have been made in neural (1) a NN-based State Autoencoder (SAE), which provides network (NN) deep learning approaches for perceptually- a bidirectional mapping between the raw input of the world based cognitive tasks including image classification [Deng et states and its symbolic/categorical representation, (2) an ac- al., 2009] and object recognition [Ren et al., 2015], as well tion model generator which generates a PDDL model of the as NN-based problem-solving systems for problem solving problem domain using the symbolic representation acquired model generation method of of Sec. 2.2 could be applied to such “symbols”. However, such a trivial SAE lacks the cru- The latent layer The output converges cial properties of generalization – ability to encode/decode converges to the categorical distrib. to the input unforeseen world states to symbols – and robustness – two similar images that represent “the same world state” should map to the same symbolic representation. Thus, we need a mapping where the symbolic representation captures the Figure 2: Step 1: Train the State Autoencoder by minimiz- “essence” of the image, not merely the raw pixel vector. The ing the sum of the reconstruction loss (binary cross-entropy main technical contribution of this paper is the proposal of between the input and the output) and the variational loss a SAE which is implemented as a Variational Autoencoder of Gumbel-Softmax (KL divergence between the actual la- [Kingma et al., 2014] with a Gumbel-Softmax (GS) activa- tent distribution and the random categorical distribution as the tion function [Jang et al., 2017]. target). As the training continues, the output of the network An AutoEncoder (AE) is a type of Feed-Forward Network converges to the input images. Also, as the Gumbel-Softmax (FFN) that uses unsupervised learning to produce an image temperature τ decreases during training, the latent values ap- that matches the input [Hinton and Salakhutdinov, 2006]. proaches the discrete categorical values of 0 and 1. The intermediate layer is said to have a Latent Represen- tation of the input and is considered to be performing data compression. AEs are commonly used for pretraining a neu- by the SAE, and (3) a symbolic planner. Given only a set of ral network. A Variational AutoEncoder (VAE) [Kingma and unlabeled images from the domain as input, we train (unsu- Welling, 2013] is a type of AE that forces the latent layer (the D pervised) the SAE and use it to generate , a PDDL repre- most compressed layer in the AE) to follow a certain distri- sentation of the image-based domain. Then, given a planning bution (e.g., Gaussian) for given input images. Since the tar- problem instance as a pair of initial and goal images such get random distribution prevents backpropagating the gradi- as Fig. 1, LatPlan uses the SAE to map the problem to a sym- ent, most VAE implementations use reparametrization tricks, D bolic planning instance in , and uses the planner to solve the which decompose the target distribution into a differentiable problem. We evaluate LatPlan using image-based versions of distribution and a purely random distribution that does not the 8-puzzle, LightsOut, and Towers of Hanoi domains. require the gradient. For example, the Gaussian distribution N(σ; µ) can be decomposed into µ + σN(1; 0). 2 LatPlan: System Architecture Gumbel-Softmax (GS) reparametrization is a technique for This section describe the LatPlan architecture and the cur- enforcing a categorical distribution on a particular layer of the rent implementation, LatPlanα. LatPlan works in 3 phases. In neural network. [Jang et al., 2017]. A “temperature” param- Phase 1 (symbol-grounding, Sec. 2.1), a State AutoEncoder eter τ, which controls the magnitude of approximation to the providing a bidirectional mapping between raw data (e.g., im- categorical distribution, is decreased by an annealing sched- ages)1 and symbols is learned (unsupervised) from a set of ule τ max(0:1; exp(−rt)) where t is the current training unlabeled images of representative states. In Phase 2 (action epoch and r is an annealing ratio. Using a GS layer in the net- model generation, Sec. 2.2), the operators available in the do- work forces the layer to converge to a discrete one-hot vector main is generated from a set of pairs of unlabeled images, when the temperature approaches near 0. and a PDDL domain model is generated. In Phase 3 (plan- The SAE is comprised of multilayer perceptrons combined ning, Sec. 2.3), a planning problem instance is input as a pair with Dropouts and Batch Normalization in both the encoder of images (i; g) where i shows an initial state and g shows and the decoder networks, with a GS layer in between. The a goal state. These are converted to symbolic form using the input to the GS layer is the flat, last layer of the encoder net- SAE, and the problem is solved by the symbolic planner. For work. The output is an (N; M) matrix where N is the number example, an 8-puzzle problem instance in our system consists of categorical variables and M is the number of categories. of an image of the start (scrambled) configuration of the puz- The input is fed to a fully connected layer of size N × M, zle (i), and an image of the solved state (g). Finally, the sym- which is reshaped to a (N; M) matrix and processed by the bolic, latent-space plan is converted to a sequence of human- GS activation function. comprehensible images visualizing the plan (Sec. 2.4). Our key observation is that these categorical variables can be used directly as propositional symbols by a symbolic rea- 2.1 Symbol Grounding with a State Autoencoder soning system, i.e., this provides a solution to the symbol grounding problem in our architecture The State Autoencoder (SAE) provides a bidirectional map- .
Details
-
File Typepdf
-
Upload Time-
-
Content LanguagesEnglish
-
Upload UserAnonymous/Not logged-in
-
File Pages7 Page
-
File Size-