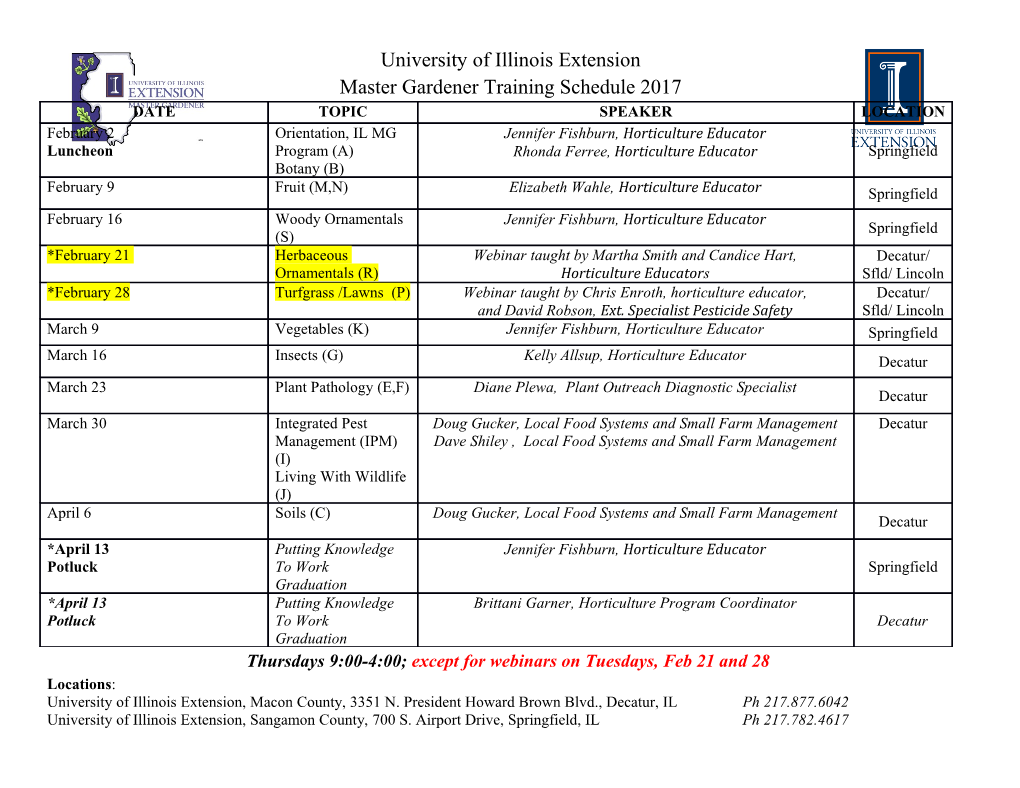
10707 Deep Learning Russ Salakhutdinov Machine Learning Department [email protected] http://www.cs.cmu.edu/~rsalakhu/10707/ Neural Networks II Neural Networks Online Course • Disclaimer: Much of the material and slides for this lecture were borrowed from Hugo Larochelle’s class on Neural Networks: https://sites.google.com/site/deeplearningsummerschool2016/ • Hugo’s class covers many other topics: convolutional networks, neural language model, Boltzmann machines, autoencoders, sparse coding, etc. • We will use his material for some of the other lectures. 2 g0(a)=g(a)(1 g(ag))0(a)=g(a)(1 g(a)) • − • − 2 2g0(a)=g0(ag)=1(a)(1 gg((aa))) g0(a)=1 g(a•) • −− • − 2 g0(a)=1 Initializationg(a) 2 2 (k) (k) 2 • ⌦(✓)=(k−) W = W k i j 2 (i,jk) 2 k F ⌦(✓)=• Initializek i biases•j W toi,j 0 = k(k)W F (k) 2|| || • ⌦(✓)= k i j Wi,j||⇣ = ||⌘k W F g0(a)= g(a)(1 • g(a)) P P P (k) || P|| • • For weights− ⇣ W(k) ⌦⌘(✓)=2⇣ W ⌘ P P P•(kr) P P P (Pk) P (k) ⌦ (✓)=2W2 W(k) ⌦(✓)=2W • rWg0(a)=1- Cang( nota•) rinitialize weights to 0 with tanh (activationk) • − ⌦(✓)= k i (k)j Wi,j Ø All ⌦gradients•(✓)=(k would)2 be zeroW (saddle| point)| ⌦(✓)= • W(k) k i j | i,j(k|) 2 ⌦(✓)= kk i jj Wi,ji,j =P Pk WP F (k) • • - Can not initialize| WP all(k) |weights⌦P(✓P) = ||to sign( the(k) sameW|| value) •W⇣r(k) ⌦(✓⌘) = sign(W ) P PØ All•P rhidden(k) units in aP layer will always behave the same (k) ⌦P(✓)=2P WP (k) (k) (Wk) ⌦(✓) = sign(Wsign((k)W) ) =1 (k) 1 (k) • Wr sign(W )i,j =1i,j (k) W 1 >0(k) W <0 • r Ø Need• to break symmetryWi,j >0 i,jWi,j−<0 i,j • (k) − ⌦(✓)=(k) k i j Wi,j sign(• W - )Samplei,j =1 W | ( k ( ) k from)U(k|[) b, b 1 ] b =( k ) , wherep6 pH6 Wi,jWi,j >i,j0 U [ Wb,i,j b] <b0 = k Hk • • −− pHk+Hpk H1 k+Hk 1 P P P • (k) − − − W(k) ⌦(✓) = sign(W ) • r(k) p6 Sample around 0 and W U [(k) b, b] b = H sign(i,j W ) =1 (k) 1 (k) k i,j W >p0 Hk+WHk <10 break symmetry • • − i,j − i,j − (k) p6 (k) Wi,j U [ b, b] b = Size of Hk h (x) 3 pHk+Hk 1 • − − 4 4 4 4 µ = 1 x(t) • T t 1 (t) σ2 = P1 (x(t) µ)2 µ = T t x T 1 t • • b − − 1 (t) σ2 = P1 (x(t) µµ1 )=2 PT (ttx) (t) T 1 t ⌃b =• T 1 t(x µb)(x µ)> • b − • − − − − 2 1 (t) 2 1 σ = P (x µ) ⌃ = P(x(t) µ)(xP(t)T 12µ) t 2 b T 1 t E[b µ•]=bbµ E[−σ ]=> σb E− ⌃ =b ⌃ • − • − − P ⌃ = 1 P(x(t) hµ)(ix(t) µ) E[b µ]=µ E[σ2]=µ µσb2 E T⌃ 1 = ⌃t b > b−• b −b b − b − • • pσ2/T h i P 2 2 µ µ b E[b µ]=µ E[σ ]=σ E ⌃ = ⌃ b− b b b b • pσ2/T µ b•µ 1.96 σ2/T h i b • 2 ±µ −µ b 2 b− p b b µ µ 1.96 σ• /Tpσ2/T • 2 ± − • b b (1) (T ) b ✓ = arg max p(x ,...,x ) p µ b µ 1.96 σ2/T ✓ • b b• 2 ± − (1) (T ) ✓ = arg max p(xb ,...,x ) ✓ p • p(x(1),...,x(T ))= p(x(t)) • b b b (1) (T ) ✓ = arg max p(xt ,...,x ) • p(x(1),...,x(T ))= p(x(t)) ✓ Y T 1 1 (t) (tt) T− ⌃ = T t(x µ)(xYb µ)> • • − −(1) (T ) (t) T 1 1 (t) (t) p(x ,...,x )= p(x ) − ⌃ = (x µ)(xP µ)> • T T t b− − Machine learning t b b Y P Model Selectionb Supervised T 1 Machine learning1 example:learning(t) (x,y()t) x y −b ⌃ = b (x µ)(x µ)> • • T T t − − • Training Protocol: Supervised learningTraining example: set: (xtrain,y) =x y(x(t),y(t)) • • b D P { Machine} learning train (t) (t) b b - Train your model on theTraining Training set:Set f(x;=✓) (x ,y ) • •D Supervised{ learning} example: (x,y) x y f(x; ✓) valid• test - For model selection,• use Validation Set •D TrainingD set: train = (x(t),y(t)) Ø Hyper-parameter search: hidden layer size,• learning rate, D { } number of iterations/epochs, etc. f(x; ✓) • - Estimate generalization performance using the Testvalid Set test •D D • Remember: Generalization is the behavior of the model on unseen examples. 4 5 5 5 Early Stopping • To select the number of epochs, stop training when validation set error increases (with some look ahead). 5 Tricks of the Trade: • Normalizing your (real-valued) data: Ø for each dimension xi subtract its training set mean Ø divide each dimension xi by its training set standard deviation Ø this can speed up training • Decreasing the learning rate: As we get closer to the optimum, take smaller update steps: i. start with large learning rate (e.g. 0.1) ii. maintain until validation error stops improving iii. divide learning rate by 2 and go back to (ii) 6 g0(a)=g(a)(1 g(a)) • − 2 g0(a)=1 g(a) • − 2 ⌦(✓)= W (k) = W(k) 2 • k i j i,j k || ||F ⇣ ⌘ P P P (k) P (k) ⌦(✓)=2W • rW ⌦(✓)= W (k) • k i j | i,j | (k) (k) ⌦P(✓)P = sign(P W ) • rW (k) sign(W )i,j =1W(k)>0 1W(k)<0 • i,j − i,j (k) p6 (k) Wi,j U [ b, b] b = Hk h (x) pHk+Hk 1 • − − a(3)(x)=b(3) + W(3)h(2) • a(2)(x)=b(2) + W(2)h(1) • a(1)(x)=b(1) + W(1)x • h(3)(x)=o(a(3)(x)) • h(2)(x)=g(a(2)(x)) • h(1)(x)=g(a(1)(x)) • b(3) b(2) b(1) • W(3) W(2) W(1) xf(x) • @f(x) f(x+✏) f(x ✏) − − • Mini-batch,@x ⇡ 2✏ Momentum f(x) x ✏ • • Make updatesf(x based+ ✏) onf( xa mini-batch✏) of examples (instead of a single example):• − 1 ↵ = Ø the• gradientt=1 is tthe average1 regularized loss for that mini-batch Ø can give a more2 accurate estimate of the gradient Pt1=1 ↵t < ↵t Ø can• leverage matrix/matrix1 operations, which are more efficient ↵P = ↵ • t 1+δt • Momentum: Can ↵use an exponential average of previous ↵t = tδ 0.5 < δ 1 δ gradients:• (t) (t 1) = l(f(x(t)),y(t))+β − • r✓ r✓ r✓ Ø can get pass plateaus more quickly, by ‘‘gaining momentum’’ 7 4 Adapting Learning Rates • Updates with adaptive learning rates (“one learning rate per parameter”) Ø Adagrad: learning rates are scaled by the square root of the cumulative sum of squared gradients 2 (t) (t) (t) (t 1) (t) (t) (t) ✓l(f(x ),y ) γ = γ − + ✓l(f(x ),y ) ✓ = r r r γ(t) + ✏ ⇣ ⌘ Ø RMSProp: instead of cumulative sum, use exponentialp moving average 2 (t) (t 1) (t) (t) γ = βγ − +(1 β) l(f(x ),y ) − r✓ ⇣ ⌘ (t) (t) (t) ✓l(f(x ),y ) ✓ = r Ø Adam: essentially combines r γ(t) + ✏ RMSProp with momentum p 8 g0(a)=g(a)(1 g(a)) • − 2 g0(a)=1 g(a) • − 2 ⌦(✓)= W (k) = W(k) 2 • k i j i,j k || ||F ⇣ ⌘ P P P (k) P (k) ⌦(✓)=2W • rW ⌦(✓)= W (k) • k i j | i,j | (k) (k) ⌦P(✓)P = sign(P W ) • rW (k) g0(a)=sign(g(a)(1 Wg(a)) )i,j =1W(k)>0 1W(k)<0 • • − i,j − i,j g0(a)=g(a)(1 g(a)) 2 g0(a)=1 g(a) • • −− 2 (k) g0(a)=g(a)(1 g(a)) p g0(a)=1 g(a) • 2 − 6 (k) • − W U [ (k)b, b] b = (k) 2 Hk h (x) ⌦(✓)= ki,ji j Wi,j g0=(a)=k g(Wa)(12 Fg(a)) • • 2 −g0•(a)=1 ||g(a) p−|| Hk+Hk 1 g (a)=g(a)(1 g((ka))) (k) 2 − g (a⌦)=(✓)=g(a0)(1 g(a)) W ⇣ •= ⌘ W − 2 •0 • k iP jP−Pi,j (k) kg0||(a)=1P ||F g(a) 2 • W(−k) ⌦(✓)=22W • − (k) (k) 2 • rg0(a)=12 (3)g(⇣a) ⌘ ⌦((3)✓)= (3) W(2)i,j = W F g0(a)=1• Pg(a)P P− (k) P k i j 2 k (k) ⌦(✓)=2aW (x)=• (bk) + W h (k) || ||(k) 2 • W − 2 ⌦(✓)= W = W • r ⌦(✓)= k i 2 j W(ki,j) (kk) 2 i ⇣ j ⌘i,j k F ⌦(✓•)= (k) W • = PWP P (k) P || || • k i (kj) | i,j |W((kk)) ⌦2k(✓)=2WF ⌦(✓)=• k i j Wi,j = k W F || || ⇣ ⌘ • ⌦(✓)= k iP(2)jPWi,jP⇣ •(k||r⌘) (2)|| P (2)P P(1)(k) P • W(k) ⌦aP(⇣✓)P =|(P sign(x⌘ )=(k|)W b) PW+(k) ⌦W(✓)=2hW (k) •PrPW(kP) ⌦((✓k))=2W P ⌦•(✓r)= W W(k) ⌦•(✓r)=2•W (k) k i j i,j (k) ⌦P(✓)P =( sign(kP) W ) • | | • r W (k) (k) • r sign(W )i,j =1W(k)>0 ⌦1W(✓()=k)<0 W • ⌦(✓)= (1)k (k)i j Wi,ji,j −(1) i,jP Pk(1)Pi j(k) i,j ⌦(✓)=• (k) aW (x)=| |b•W(k) ⌦+(✓)W = sign(xW | ) | • sign(Wk )ii,j =1j | i,j(k|) 1• r(k) (k) P WPi,jP>0 (kW) i,jp<0 (k) • •(k) ⌦(✓) = sign(W− ) 6 (k) P P(k) P WW U [ b,(k) b] b = W(k)H⌦(✓)h = sign((x) W ) •PrPi,j P k (k) (k) W(k) ⌦•(✓) = sign(W− ) pHsign(k•+HrWk 1 )i,j =1W >0 1W <0 • r (k) (k)(3) p6 • (3)− (k) i,j − i,j sign(W ) =1 (k) 1 (k) (k) W U [ b, bh] bi,j=(xW)=>0 o(WHak <h0 (x(x))) (k) (k) i,j(k) i,j sign(i,j W )i,j =1 1 • (3) (k) (3) pHk+((3)kH) k −(2)1 W >0 W <0 sign(• W )i,j−=1W >0 1W <−0 •(k) i,jp − i,j • a •(x)=i,jb −+ Wi,j h 6 (k) (k) pWi,j U [ b, b] b = Hk h (x) • 6 (k) pHk+Hk 1 (3) Wi,j (3)U [ b, b(3)] b =(2) • (kH)k− h (x) p−6 (k) a(k) (•x)=(2)b +−(2)W(2)p6h p(2)Hk+(1)Hk (1k)(2) W U [ ab, b(]x)=b = b + W Hhk h−W(i,jx) U [ b, b] b = Hk h (x) • i,j h H(+xH)=g(a (x)) pHk+Hk 1 • • − p k k 1 (3)• (3)− (3) (2) − (2) (3) (2) (3)(2) (1)−(3) (2)a (x)=b + W h a (x)=a(1)b•(x)=+bW(1)+hW (1)h• •(3) • a(3)(x)=(3)b (2)+ W x (3) (3) (3) (2) a (x)=• b + W (1)h (2)a (1)(x)=(2)b +(2)W (1)h • (1) a(2)(x(1))=b(2)(1)+ W(2)h(1)a• (x)=b + W h a (x)=(3)b h+ W ((3)xx )=• g(a (x)) a•(2)(x)=• hb(2) (+x)=W(2)oh(a(1) (x)) (2) (2) (2) (1) • (1)• (1) (1) (1)a (x)=(1)b +(1)W h • (3) a (x)=(3)b + W x a• (x)=b + W x (1)h (•x)=(1)(2)o(a (1)(x))(2) • a• (x)=hb (+x)=W (3)gx(a (x(2))) (1)(1) (1) (1) • • h(3)(x)=boGradient(a(3)(bx)) b(3) Checkinga (x)=(3)b + W x (2) • (2) h• (x)=o(a (x)) h(3)h(x)=(x)=o(1)(a•(3)g(a(x))(x))(1) • • h(2) (x)=g(a(2) (x)) (3) (3) • • To• debugh (x your)=g implementation(a (x)) (2) ofh fprop/bprop(x)=(2)o(,a you(x can)) compare (2) (1) • (2) (1) (3) (2)h• (x)=(1)g(a (x)) h h(x)=(x)=g(3)(ag(a((2)xW))(x(1))) W• W xf(x) • • with abh finite-difference(1)(xb)=gb(a(1)( xapproximation)) (2) of the gradient:(2) • (1)h (x)=(1)g(a (x)) (1) (3) • (2) •(1)(1) h• (x)=g(a (x)) h b(x)=b g(3)(a(3)b ((2)x))(2)(1) (1) • • • Wb bW@fb(xW) xff((xx)+✏(1)) f(x ✏)(1) •• (3)h (2)(x)=(1)g(a (x)) b(3) b(3)(2) b(1)(2) (1) b• b− b − W WW@f((3)x) WW(2)f@(xx+Wxf✏)(1)f((xxxf) ✏)•(x) 2✏ • • • − ⇡− (3) (2) (1) (3) • (2)@x (1) 2✏ (3)b b(2) b(1) W @f(xW)• @ff(W(xx)+⇡✏) fxf(fx(+x✏()x✏)f(x ✏) W• W W xf(x) • − −− − • @xØ • f (@xx ) would⇡x2✏ ✏be 2the✏ loss (3) (2) (1) @•f(x) f⇡(x+✏) f(x ✏) @f(xW) f(Wx+✏) fW(x ✏) xf(x) @x • 2−✏ − • − − • ⇡Ø f(x) x ✏ @x 2✏ f(x)• x would✏ be a parameter• @f⇡(x) f(x+✏) f(x ✏) f•(x) x ✏ − − Ø f (x + ✏ ) wouldf(x be✏) the lossf if(• xyou) @ xaddx ⇡ ✏ to the parameter2✏ • • − • f(x +Ø✏) f (x ✏ ) would be the loss if youf( xsubtract) x ✏ to the parameter • − • 9 4 4 4 4 4 4 4 Debugging on Small Dataset • Next, make sure your model can overfit on a smaller dataset (~ 500-1000 examples) • If not, investigate the following situations: Ø Are some of
Details
-
File Typepdf
-
Upload Time-
-
Content LanguagesEnglish
-
Upload UserAnonymous/Not logged-in
-
File Pages58 Page
-
File Size-