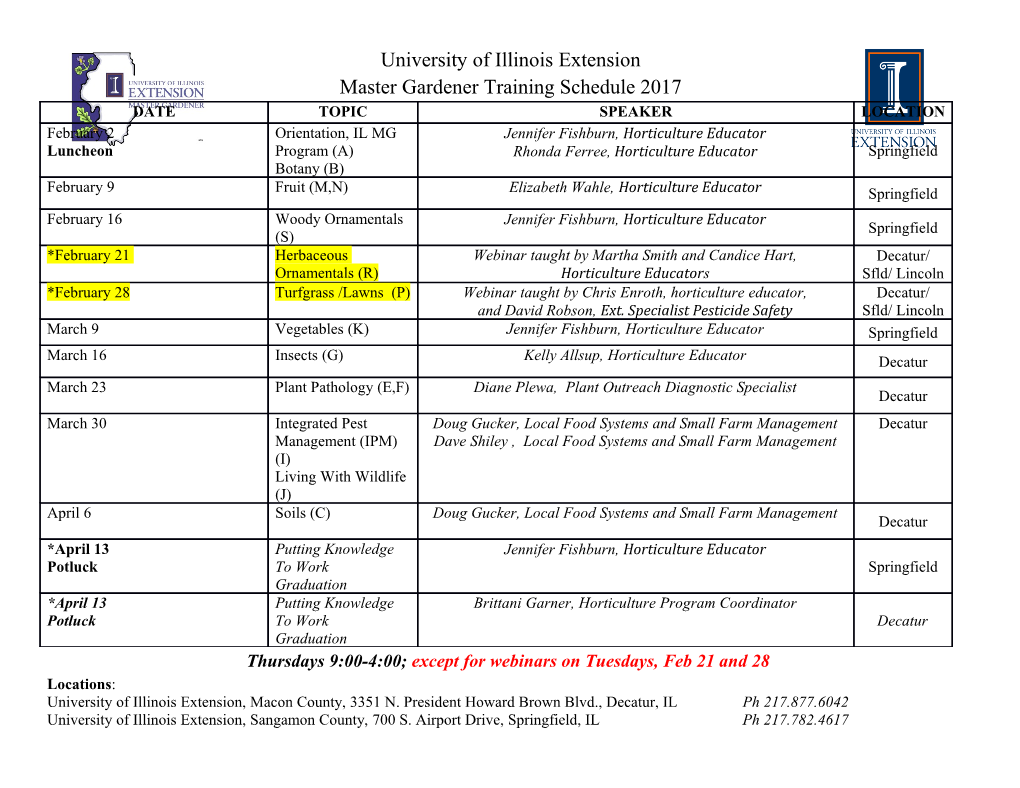
Scientia Iranica E (2017) 24(5), 2605{2622 Sharif University of Technology Scientia Iranica Transactions E: Industrial Engineering www.scientiairanica.com Maximum multivariate exponentially weighted moving average and maximum multivariate cumulative sum control charts for simultaneous monitoring of mean and variability of multivariate multiple linear regression pro les R. Ghashghaei and A. Amiri Department of Industrial Engineering, Faculty of Engineering, Shahed University, Tehran, Iran. Received 24 November 2015; received in revised form 15 March 2016; accepted 13 August 2016 KEYWORDS Abstract. In some applications, quality of product or performance of a process is described by some functional relationships among some variables known as multivariate Multivariate multiple linear pro le in the literature. In this paper, we propose Max-MEWMA and Max- linear regression MCUSUM control charts for simultaneous monitoring of mean vector and covariance pro les; matrix in multivariate multiple linear regression pro les in Phase II. The proposed control Simultaneous charts also have the ability to diagnose whether the location or variation of the process monitoring; is responsible for out-of-control signal. The performance of the proposed control charts is Phase II; compared with that of the existing method through Monte-Carlo simulations. Finally, the Diagnosis aids. applicability of the proposed control charts is illustrated using a real case of calibration application in the automotive industry. © 2017 Sharif University of Technology. All rights reserved. 1. Introduction Woodall et al. [3], Wang and Tsung [4], Woodall [5], Zou et al. [6], and Amiri et al. [7]. A pro le monitoring Sometimes, quality of a process or product in the indus- problem consists of two phases, including Phase I try and non-industry is characterized by a relationship and Phase II. The purpose of Phase I monitoring between two or more variables, which is presented by is providing an analysis of the preliminary data for a `pro le' in the literature. In di erent applications, estimating the process parameters. The main purpose simple linear regression, multiple linear or polynomial of Phase II pro le monitoring is designing a control regression, or even more complicated models such as scheme to detect di erent out-of-control scenarios in nonlinear regressions are used to model the quality of the process parameters. Monitoring of di erent types processes. The applications of pro le monitoring in of linear regression pro les, such as simple linear, the literature have been introduced by several authors multiple linear, and polynomial regression pro les, has such as Kang and Albin [1], Mahmoud and Woodall [2], been investigated by many researchers. Kang and Albin [1], Kim et al. [8], Zou et al. [6], Keshtelia et al. [9], Noorossana and Ayoubi [10], and Chuang et *. Corresponding author. Tel.: +98 21 51212023; E-mail addresses: [email protected] (R. Ghashghaei); al. [11] have studied Phase II monitoring of simple [email protected] (A. Amiri) linear pro les. Researchers such as Kang and Albin [1], Mahmoud and Woodall [2], Mahmoud et al. [12], and doi: 10.24200/sci.2017.4385 Chuang et al. [11] have considered monitoring of simple 2606 R. Ghashghaei and A. Amiri/Scientia Iranica, Transactions E: Industrial Engineering 24 (2017) 2605{2622 linear pro les in Phase I. Multiple linear regression process parameters. Cheng and Thaga [31] proposed and polynomial pro les have been studied by Zou et Max-MCUSUM control chart to detect any changes al. [6], Kazemzadeh et al. [13,14], Amiri et al. [15], and in mean vector and covariance matrix simultaneously. Wang [16]. Zhang et al. [32] proposed a new control chart (ELR In some situations, quality of a process or a control chart) based on the combination of Generalized product can be e ectively characterized by two or more Likelihood Ratio (GLR) test and the Exponentially multiple linear regression pro les in which response Weighted Moving Average (EWMA) control chart for variables are correlated, referred to as multivariate simultaneous monitoring of mean vector and covari- multiple linear regression pro les [17]. Noorossana et ance matrix in the multivariate process. Wang et al. [18] proposed three control chart schemes for Phase al. [33] applied the generalized likelihood ratio test II monitoring of multivariate simple linear pro les. and the multivariate exponentially weighted moving They also used the term Average Run Length (ARL) covariance control chart to monitor the mean vector to evaluate the proposed control charts. Noorossana et and the covariance matrix of a multivariate normal al. [19] developed four control charts in Phase I for process, simultaneously. Other research for simulta- monitoring of multivariate multiple linear regression neous monitoring of multivariate process consists of pro les. They compared the performance of the Khoo [34], Hawkins and Maboudou-Tchao [35], Ramos proposed control charts through simulation studies in et al. [36], and Pirhooshyaran and Niaki [37]. For terms of probability of a signal. Zhang et al. [20] detailed information on simultaneous monitoring of the developed a new modelling and monitoring framework process location and dispersion, refer to the review for analysis of multiple linear pro les in Phase I. Their paper provided by McCracken and Chakraborti [38]. framework used the regression-adjustment method in According to the literature, in most of the control the functional principal component analysis. Eyvazian charts proposed for monitoring of multivariate multiple et al. [21] suggested four control charts to monitor mul- linear regression pro les, mean vector and covariance tivariate multiple linear regression pro les in Phase II. matrix are monitored with separate control charts. In They evaluated the performance of the proposed con- addition, these control charts are unable to diagnose trol charts through simulation studies in terms of the whether the location or the dispersion is responsible ARL criterion. They also used a numerical example for out-of-control signal. In this paper, we propose to assess the performance of the developed control two methods for simultaneous monitoring of mean charts. Amiri et al. [17] introduced a diagnosis method vector and covariance matrix in multivariate multiple to identify the pro les and parameters responsible linear regression pro les. Moreover, the proposed for out-of-control signal in multivariate multiple linear control charts are able to diagnose whether location or regression pro les in Phase II. dispersion is responsible for out-of-control signal. The In addition to the problems noted above, prac- performance of the proposed control charts is compared titioners are interested in developing some kinds of with ELRT control chart proposed by Eyvazian et control charts which can monitor mean and variabil- al. [21] in Phase II in terms of Average Run Length ity of processes simultaneously. In fact, the quality (ARL) and Standard Deviation Run Length (SDRL). engineers want to have a single control chart instead The structure of the rest of this paper is as follows: of two or more. In practice, the control charts for In Section 2, we express multivariate multiple linear monitoring both process mean and variability should regression pro les model. In Section 3, the ELRT be implemented together because assignable causes can control chart is described. In Section 4, the proposed a ect both of them. In recent years, joint monitoring control charts are expressed. In Section 5, diagnosing of process mean and dispersion in both univariate procedure is explained. In Section 6, the comparison and multivariate cases has been considered by some analysis of the proposed control charts is provided researchers. Simultaneous monitoring of the mean and through an illustrative example. In Section 7, the variance in univariate process has been studied by application of the proposed control charts is illustrated Khoo et al. [22], Zhang et al. [23], Guh [24], Memar by a real dataset. Finally, our concluding remarks and and Niaki [25], Teh et al. [26], Sheu et al. [27], Haq et future research are given in Section 8. al. [28], and Prajapati and Singh [29]. The simultane- ous monitoring of multivariate process mean vector and 2. Model and Assumptions covariance matrix has also been addressed by several authors. Chen et al. [30] proposed a control scheme Let us assume that for the kth random sample col- called Max-EWMA for monitoring both mean and lected over time, we have n observations given as variance simultaneously. They performed a comparison (x1i; x2i; : : : ; xqi; y1ik; y2ik; : : : ; ypik), i = 1; 2; : : : ; n analysis and found that their proposed control chart where p and q are the numbers of response variables performed better than the combination of chi-square and explanatory variables, respectively. When the and jSj control charts when small shifts occurred in the process is in statistical control, the model that relates R. Ghashghaei and A. Amiri/Scientia Iranica, Transactions E: Industrial Engineering 24 (2017) 2605{2622 2607 the response variables with explanatory variables is a in which is covariance matrix of error terms, ECk multivariate multiple linear regression model and is and ESk are corresponding exponentially weighted given as follows [21]: moving average statistics given by: Yk = XB + Ek; (1) ECk = Ck + (1 ) ECk1; (6) or equivalently: ESk = Sk + (1 ) ESk1; (7) 2 3 y y : : : y Pn 11k 12k 1pk where C is equal to (y x B)1(y x B)T , 6y y : : : y 7 k ik i ik i 6 21k 22k 1pk 7 i=1 6 . 7 in which (y x B) is the ith row of matrix (Y XB). 4 . .. 5 ik i k Sk in Eq. (7) is computed as: yn1k yn2k : : : ynpk 2 3 2 3 ^ T ^ (Yk X(EBk)) (Yk X(EBk)) 1 x11 : : : x1q 01 02 : : : 0p Sk = ; (8) 6 7 6 7 n 61 x21 : : : x2q 7 6 11 12 : : : 1p7 = 6.
Details
-
File Typepdf
-
Upload Time-
-
Content LanguagesEnglish
-
Upload UserAnonymous/Not logged-in
-
File Pages18 Page
-
File Size-