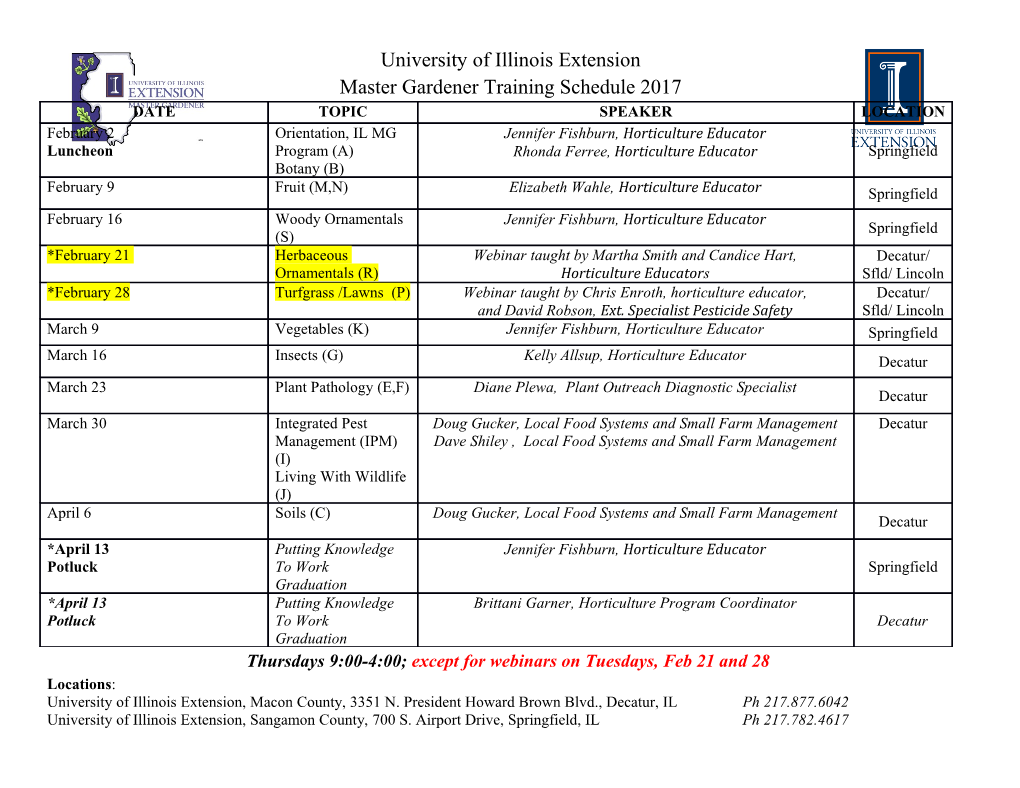
CAUSES, COINCIDENCES, AND THEORIES a dissertation submitted to the department of psychology and the committee on graduate studies of stanford university in partial fulfillment of the requirements for the degree of doctor of philosophy Thomas L. Griffiths December 2004 c Copyright by Thomas L. Griffiths 2005 All Rights Reserved ii I certify that I have read this dissertation and that, in my opinion, it is fully adequate in scope and quality as a disser- tation for the degree of Doctor of Philosophy. Joshua B. Tenenbaum (Principal Co-Advisor) I certify that I have read this dissertation and that, in my opinion, it is fully adequate in scope and quality as a disser- tation for the degree of Doctor of Philosophy. Gordon H. Bower (Principal Co-Advisor) I certify that I have read this dissertation and that, in my opinion, it is fully adequate in scope and quality as a disser- tation for the degree of Doctor of Philosophy. Ewart A. C. Thomas Approved for the University Committee on Graduate Studies. iii Preface Sir Edmond Halley was an astronomer, sailor, and arguably the first statistician. In 1695, Halley was computing the orbits of a set of comets for inclusion in Newton’s Principia mathematica when he noticed a surprising regularity: the comets of 1531, 1607, and 1682 took remarkably similar paths across the sky, and visited the Earth approximately 76 years apart. In the first edition of the Principia, Newton had argued that comets, like planets, follow orbits corresponding to conic sections – parabolas, hyperbolas, and ellipses – although he concluded that “as to the transverse diameters of their orbits, and the periodic times of their revolutions I leave them to be determined by comparing comets together which after long intervals of time return again on the same orbit” (Newton, 1687/1962, p. 532). Halley inferred that the comets of 1531, 1607, and 1682 were not three separate events, but three consequences of a single common cause: a comet that had visited the Earth three times, travelling in an elliptical orbit. He went on to state that “if according to what we have already said it should return again about the year 1758, candid posterity will not refuse to acknowledge that this was first discovered by an Englishman” (Halley, 1752, p. Ssss3).1 The comet returned in December 1758, as predicted, and has continued to visit the Earth approximately every 76 years since, providing a sensational confirmation of Newton’s physics. The discovery of Halley’s comet is a compelling example of causal induction: inferring causal structure from data. In modern scientific practice, causal relationships are identified through careful experimentation and statistical analysis. While the development of sta- tistical methods for designing and analyzing experiments has greatly streamlined scientific 1Halley gave the more precise prediction of “about the end of the year 1758, or the beginning of the next” (1752, p. Rrrr2) earlier in the text. Making this prediction was by no means trivial, as it had to take into account the perturbation of the orbit of the comet by Saturn and Jupiter. Detailed accounts of Halley’s discovery are provided by Cook (1998), Hughes (1990) and Yeomans (1991). iv argument, science was possible before statistics: in many cases, the causal relationships be- tween variables that are critical to understanding our world could be discovered without any need to perform explicit calculations. Science was possible because people have an intuitive capacity for causal induction, using data to assess the plausibility of causal relationships. This capacity is sufficiently accurate as to have resulted in genuine scientific discoveries, and allows us to construct the intuitive theories that express our knowledge about the world. The same notions about causality that allowed Halley to discover his comet guide our more mundane everyday inferences, from evaluating whether drinking coffee improves our productivity to working out which programs crash a computer. Explaining Halley’s discovery requires appealing to two factors: prior knowledge, and intuitive statistical inference. The prior knowledge that guided Halley was the physical theory laid out by Newton in the Principia mathematica. Newton’s theory identified the entities and properties relevant to understanding a physical system, formalizing notions like velocity and acceleration, and characterized the relations that can hold among these entities. This physical theory allowed Halley to generate a set of hypotheses about the causal structure responsible for his astronomical observations: they could have been produced by three different comets, each travelling in a parabolic orbit, or by one comet, travelling in an elliptical orbit. Choosing between these hypotheses required the use of statistical inference. While Halley made no formal computations of the probabilities involved, the similarity in the paths of the comets and the fixed interval between observations convinced him that “it was highly probable, not to say demonstrative, that these were but one and the same Comet” (from the Journal Book of the Royal Society, July 1696, reproduced in Hughes, 1990, p. 353). Scientific inferences like that of Halley can provide clues about how people solve problems of causal induction in the course of everyday life. The capacity to reason about the causes of events is an essential part of cognition from early childhood, whether it concerns the forces involved in physical systems (e.g., Shultz, 1982b), the essential properties of natural kinds (e.g., Gelman & Wellman, 1991), or the mental states of others (e.g., Perner, 1991). Often, these causal relationships need to be inferred from data. Explaining how people make these inferences is not just a matter of accounting for how causation is identified from correlation, but of accounting for how complex causal structure is inferred in the absence of (statistically significant) correlation. Halley’s discovery illustrates that people can infer causal relationships from samples too small for any statistical test to produce significant v results – in this case, three observations – and solve problems like inferring hidden causal structure that still pose a major challenge for statisticians and computer scientists. In this thesis, I will argue that both of the factors involved in Halley’s discovery – prior knowledge, in the form of a causal theory, and statistical inference – are necessary to make such inferences possible, and present a computational framework that indicates how they are combined. In the computational framework that forms the heart of this thesis, theory-based causal induction, prior knowledge is formalized as a theory which generates a hypothesis space of causal models that could have produced data. These hypotheses are evaluated by Bayesian inference, resulting in a tight coupling between prior knowledge, in the form of a causal theory, and statistical learning. I will argue that there are three aspects of prior knowledge that are central to causal induction – the ontology of entities, properties, and relations that organizes a domain, the plausibility of specific causal relationships, and the functional form of those relationships – and that these three aspects are the key constituents of causal theories. This account identifies the limitations of existing algorithms for causal induction, makes it possible to explore the relationship between causes and coincidences, identifies which aspects of causal induction should be domain-sensitive, clarifies how causal mecha- nism knowledge is involved in human causal induction (c.f. Ahn & Kalish, 2000; Glymour & Cheng, 1998; White, 1995), and suggests how statistical learning might be extended from evaluating single causal relationships to evaluating entire causal theories. The plan of the thesis is as follows. Chapter 1 introduces the key issues that I will address, discussing how prior knowledge can influence causal induction. The analysis of a scientific example indicates which aspects of prior knowledge are relevant to the assessment of new causal relationships, and I connect these aspects of prior knowledge with intuitive theories. The major challenge of the thesis is explaining how such theories can be integrated with statistical learning. Chapter 2 takes up this challenge, summarizing the background behind the compu- tational tools used throughout the thesis. The chapter introduces the fundamental ideas behind causal graphical models, which will be used as a basic representation of causal rela- tionships, and highlights the ways in which generic algorithms for learning causal structure are inadequate for explaining human inferences. Chapter 3 presents the theory-based causal induction framework. The chapter discusses how the aspects of intuitive theories relevant to causal induction can be formalized, and how vi they can be incorporated with statistical inference. The notion of a theory as a hypothesis space generator is introduced, and used to explain how top-down and bottom-up information interact in causal induction. Chapter 4 applies the theory-based approach to the problem of learning from contingency data. Causal graphical models are used to interpret two leading models of human judgments in this task, and to illustrate that they only evaluate the strength of a causal relationship, not asking the question of whether a causal relationship actually exists. A simple causal theory is used to develop an alternative model, causal support, that addresses this structural question. Causal support predicts several
Details
-
File Typepdf
-
Upload Time-
-
Content LanguagesEnglish
-
Upload UserAnonymous/Not logged-in
-
File Pages254 Page
-
File Size-