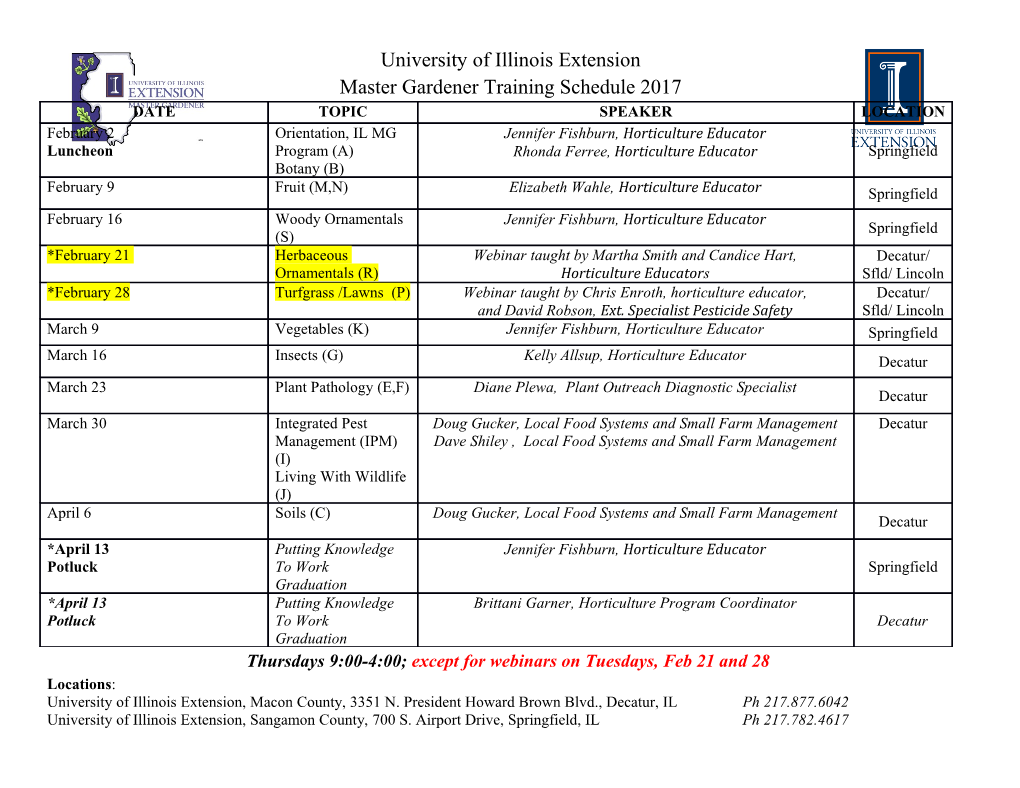
IEEE TRANSACTIONS ON AUDIO, SPEECH, AND LANGUAGE PROCESSING, VOL. 16, NO. 8, NOVEMBER 2008 1551 Integration of Speech Recognition and Machine Translation in Computer-Assisted Translation Shahram Khadivi and Hermann Ney, Senior Member, IEEE Abstract—Parallel integration of automatic speech recognition (ASR) models and statistical machine translation (MT) models is an unexplored research area in comparison to the large amount of works done on integrating them in series, i.e., speech-to-speech translation. Parallel integration of these models is possible when we have access to the speech of a target language text and to its corre- sponding source language text, like a computer-assisted translation system. To our knowledge, only a few methods for integrating ASR models with MT models in parallel have been studied. In this paper, we systematically study a number of different translation models in the context of the -best list rescoring. As an alternative to the -best list rescoring, we use ASR word graphs in order to arrive at a tighter integration of ASR and MT models. The experiments are carried out on two tasks: English-to-German with an ASR vo- cabulary size of 17 K words, and Spanish-to-English with an ASR Fig. 1. Schematic of automatic text dictation in computer-assisted translation. vocabulary of 58 K words. For the best method, the MT models re- duce the ASR word error rate by a relative of 18% and 29% on the 17 K and the 58 K tasks, respectively. language speech is a human-produced translation of the source Index Terms—Computer-assisted translation (CAT), speech language text. The overall schematic of a speech-enabled com- recognition, statistical machine translation (MT). puter-assisted translation system is depicted in Fig. 1. The idea of incorporating ASR and MT models in a CAT system has been early studied by researchers involved in the I. INTRODUCTION TransTalk project [4], [5], and researchers at IBM [1]. In [1], the authors proposed a method to integrate the IBM translation model 2 [6] with an ASR system. The main idea was to design HE GOAL of developing a computer-assisted translation a language model which combines the trigram language model T (CAT) system is to meet the growing demand for high- probability with the translation probability for each target word. quality translation. A desired feature of CAT systems is the They reported a perplexity reduction, but no recognition results. integration of human speech, as skilled human translators are In the TransTalk project, the authors improved the ASR per- faster in dictating than typing the translations [1]. In addition, formance by rescoring the ASR -best lists with a translation another useful feature of CAT systems is to embed a statistical model. They also introduced the idea of dynamic vocabulary machine translation (MT) engine within an interactive transla- in an ASR system, where the dynamic vocabulary was derived tion environment [2], [3]. In this way, the system combines the from a MT system for each source language sentence. The better best of two paradigms: the CAT paradigm, in which the human performing of the two is the -best rescoring. translator ensures high-quality output, and the MT paradigm, in Recently, [7], [8] and [9] have studied the integration of which the machine ensures significant productivity gains. In this ASR and MT models. In the first paper, we showed a detailed paper, we investigate the efficient ways to integrate automatic analysis of the effect of different MT models on rescoring the speech recognition (ASR) and MT models so as to increase the ASR -best lists. The other two papers considered two parallel overall CAT system performance. -best lists, generated by MT and ASR systems, respectively. In a CAT system with integrated speech, two sources of in- They showed improvement in the ASR -best rescoring when formation are available to recognize the speech input: the target several features are extracted from the MT -best list. The language speech and the given source language text. The target main concept among all features was to generate different kinds of language models from the MT -best list. All of the above Manuscript received December 21, 2007; revised June 08, 2008. This work methods were based on an -best rescoring approach. In [10], was supported by the European Union under the RTD project TransType2 (IST we studied different methods for integrating MT models to 2001 32091) and the integrated project TC-STAR—Technology and Corpora for ASR word graphs instead of the -best list. Speech to Speech Translation—(IST-2002-FP6-506738). The associate editor coordinating the review of this manuscript and approving it for publication was In [11], the integration of speech into a CAT system is studied Dr. Ruhi Sarikaya. from a different point of view. To facilitate the human and ma- The authors are with the Department of Computer Science, RWTH Aachen chine interactions, the speech is used to determine the accept- University, 52056 Aachen, Germany (e-mail: [email protected]; [email protected]). able partial translations by reading parts of the target sentence Digital Object Identifier 10.1109/TASL.2008.2004301 offered by the system in addition to keyboard and mouse. In this 1558-7916/$25.00 © 2008 IEEE 1552 IEEE TRANSACTIONS ON AUDIO, SPEECH, AND LANGUAGE PROCESSING, VOL. 16, NO. 8, NOVEMBER 2008 scenario, the ASR output is constrained to the translation pro- vided by the MT system, i.e., the ASR language model has to be adapted to the MT output. Then, the integrated model can be described as introducing the This paper makes the following four contributions. acoustic model into the MT system or as introducing the MT 1) All previous methods have employed an -best rescoring model into the ASR system. strategy to integrate ASR and MT models. Here, we will Another approach for modeling the posterior probability take another step towards a full single search for the inte- is direct modeling by using a log-linear combina- gration of ASR and MT models by using ASR word graphs. tion of different models A full single search means to generate the most likely hy- pothesis based on ASR and MT models in a single pass without any search constraints. (5) 2) Furthermore we will investigate several new techniques to integrate ASR and MT systems, a preliminary description of some of these integration techniques were already pre- then the decision rule can be written as sented in our previous paper [10]. 3) In all previous works, a phrase-based MT had the least im- pact on improving the ASR baseline. In this paper, we will (6) study the reason for this failure concluding in a solution for this problem. Each of the terms denotes one of the various 4) To our knowledge, up to now no experiments have been models which are involved in the recognition procedure. Each reported in this field on a large task. Here, we will perform individual model is weighted by its scaling factor . The our experiments using the -best rescoring method and direct modeling has the advantage that additional models can the word graph rescoring method on a standard large task, be easily integrated into the overall system. The model scaling namely the European parliament plenary sessions. factors are trained on a development corpus according to The remaining part is structured as follows. In Section II, a the final recognition quality measured by the word error rate general model for parallel integration of ASR and MT systems (WER) [12]. This approach has been suggested by [13] and is described. In Section III, the details of the MT system and the [14] for a natural language understanding task, by [15] for an ASR system are explained. In Section IV, different methods for ASR task, and by [16] for an MT task. integrating MT models into ASR models are described, and in In a CAT system, by assuming no direct dependence between Section V the experimental results are discussed. and , we have the following three main models: II. COMBINING ASR AND MT MODELS In parallel integration of ASR and MT systems, we are given a source language sentence , which is to be translated into a target language sentence , and an acoustic signal , which is the spoken target language sen- tence. Among all possible target language sentences, we will choose the sentence with the highest probability In these equations, is a language model (LM) -gram probability and represents an acoustic model (AM) probability, where is the most likely segment of (1) corresponding to . The definition of the machine translation (MT) model is intentionally left open, as it will be (2) later defined in different ways, e.g., as a log-linear combination of several sub-models. (3) The argmax operator in (6) denotes the search. The search (4) is the main challenge in the integration of the ASR and the MT models in a CAT system. The search in the MT and in the ASR systems are already very complex; therefore, a full single Equation (2) is decomposed into (4) by assuming no conditional search to combine the ASR and the MT models will consider- dependency between and . The decomposition into three ably increase the complexity. In addition, a full single search knowledge sources allows for an independent modeling of the becomes more complex since there is no any specific model nor target language model , the translation model , any training data to learn the alignment between and . and the acoustic model . The general decision rule for To reduce the complexity of the search, the recognition the (pure) ASR and the (pure) MT system are as follows: process is performed in two steps.
Details
-
File Typepdf
-
Upload Time-
-
Content LanguagesEnglish
-
Upload UserAnonymous/Not logged-in
-
File Pages14 Page
-
File Size-