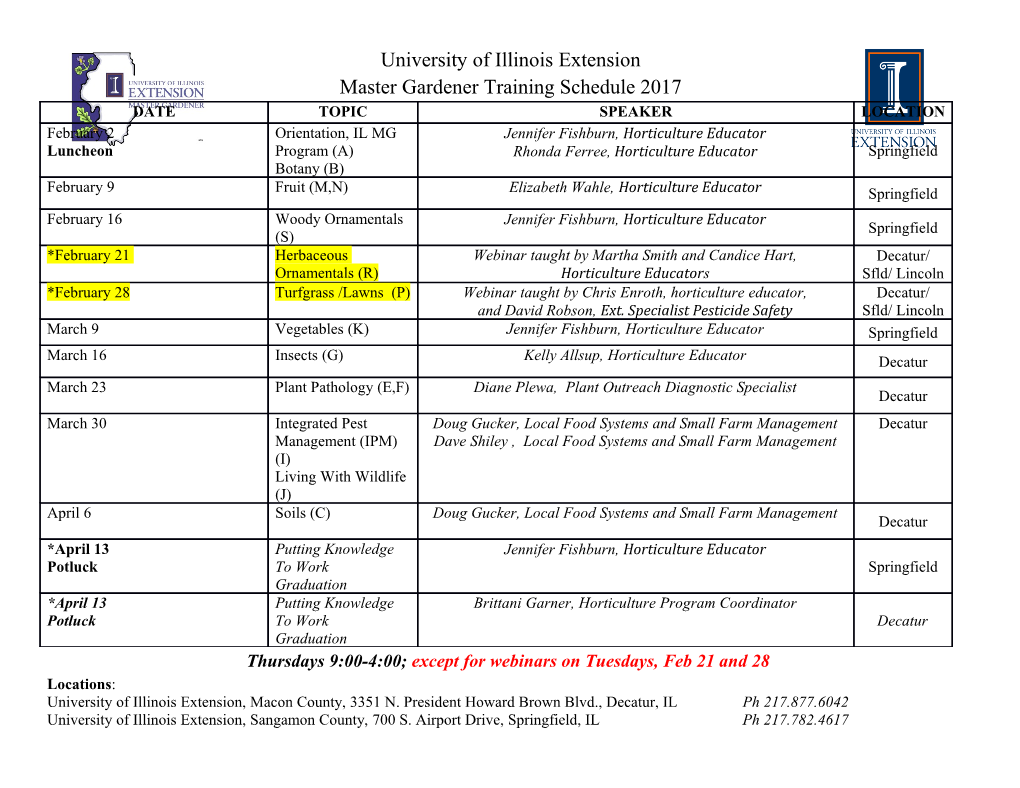
Blum et al. BMC Bioinformatics 2010, 11:368 http://www.biomedcentral.com/1471-2105/11/368 METHODOLOGY ARTICLE Open Access AMethodology factor article model to analyze heterogeneity in gene expression Yuna Blum*1,2,3, Guillaume Le Mignon1,2, Sandrine Lagarrigue1,2 and David Causeur3 Abstract Background: Microarray technology allows the simultaneous analysis of thousands of genes within a single experiment. Significance analyses of transcriptomic data ignore the gene dependence structure. This leads to correlation among test statistics which affects a strong control of the false discovery proportion. A recent method called FAMT allows capturing the gene dependence into factors in order to improve high-dimensional multiple testing procedures. In the subsequent analyses aiming at a functional characterization of the differentially expressed genes, our study shows how these factors can be used both to identify the components of expression heterogeneity and to give more insight into the underlying biological processes. Results: The use of factors to characterize simple patterns of heterogeneity is first demonstrated on illustrative gene expression data sets. An expression data set primarily generated to map QTL for fatness in chickens is then analyzed. Contrarily to the analysis based on the raw data, a relevant functional information about a QTL region is revealed by factor-adjustment of the gene expressions. Additionally, the interpretation of the independent factors regarding known information about both experimental design and genes shows that some factors may have different and complex origins. Conclusions: As biological information and technological biases are identified in what was before simply considered as statistical noise, analyzing heterogeneity in gene expression yields a new point of view on transcriptomic data. Background Recently, several works have introduced models for the Microarray technology allows the analysis of expression common information shared by all the genes. Especially levels for thousands of genes simultaneously and is a Friguet et al [4] propose to model this sharing of informa- powerful tool to characterize mRNA level variation due tion by a factor analysis structure in a method called Fac- to measured variables of interest (various phenotypes, tor Analysis for Multiple Testing (FAMT). The estimated treatments...). Typical approaches to find significant rela- factors in the model capture components of the expres- tionships between gene expressions and experimental sion heterogeneity. As well, Storey et al [3] introduce Sur- conditions ignore the correlations among expression pro- rogate Variable Analysis (SVA) to identify and estimate files and functional categories [1]. This dependence these extra sources of variation. The factors in FAMT and structure leads to correlation among test statistics which the surrogate variables in SVA are similarly designed to affects a strong control of the actual proportion of false model dependence among tests by a linear kernel but discoveries [2]. Indeed, a number of unmeasured or they are estimated differently. Contrarily to the SVA unmodeled factors independent of the variables of inter- model, independence between the factors and the experi- est may influence the expression of any particular gene mental conditions of interest is explicitly assumed in [3,4]. These factors may induce extra variability in the FAMT in order to separate clearly the effects of the expression levels and decrease the power to detect links experimental conditions on the gene expressions and the with the variables of interest. nuisance variability due to unmodeled technological effects and other known or unknown effects that could be uncontrolled in the experimental design. * Correspondence: [email protected] The major sources of expression variation are then 1 Agrocampus Ouest, UMR598, Animal Genetics, 35000 Rennes, France Full list of author information is available at the end of the article assumed to be the experimental conditions of interest, © 2010 Blum et al; licensee BioMed Central Ltd. This is an Open Access article distributed under the terms of the Creative Commons Attribution License (http://creativecommons.org/licenses/by/2.0), which permits unrestricted use, distribution, and reproduction in any medium, provided the original work is properly cited. Blum et al. BMC Bioinformatics 2010, 11:368 Page 2 of 12 http://www.biomedcentral.com/1471-2105/11/368 but also gene dependence and uncontrolled factors in the added on the first 100 gene expressions to mimic a differ- experimental design. Indeed, even after normalization, ential expression between these two groups. variation due to the experimental design still exists in Case 1: One independent variable affecting all genes expression data. The factors extracted in the residual part All genes are affected by an independent grouping vari- of the regression models explaining the gene expressions able marked by colors red and green on Figure 1A. A sin- by the experimental conditions of interest are therefore gle factor is extracted by FAMT. Figure 2A helps analyzed to give more insight both on expression hetero- interpreting this factor and shows that it clearly discrimi- geneity among sampling units and the contribution of nates the two colored groups of individuals (P-value ≤ 2.2 some biological processes to gene dependence. First, fac- × 10-16). This shows a high association between the factor tors are extracted from illustrative expression data sets and the independent grouping variable. The genes repre- with simple patterns of expression heterogeneity in order sentation does not show any particular structure. In this to show how they can straightforward be related to simple case the factor estimated by FAMT can therefore sources of heterogeneity. Henceforth, the same factor be easily interpreted through the individuals representa- model approach is used to analyze an expression data set tion. initially generated to map quantitative trait loci (QTL) for Case 2: One independent variable affecting a set of genes abdominal fatness (AF) in chickens, especially on chro- Only genes 70-170 are affected by an independent group- mosome 5 (GGA5) [5]. This data set concerns hepatic ing variable marked by colors red and green on Figure 1B. transcriptome profiles for 11213 genes of 45 half sib male A single factor is also found using FAMT. As shown on chickens generated from a same sire. This sire was gener- Figure 2B, the factor discriminates the two groups of indi- ated by successive inter-crossing of two experimental viduals (P-value ≤ 2.2 × 10-16) and the two groups of genes chicken lines divergently selected on AF and was known (P-value ≤ 2.2 × 10-16). In this case, the estimated factor to be heterozygous for an AF QTL on the GGA5 chromo- can be interpreted through the individuals and genes rep- some around 175 cM (For more details, see [5]). The 45 resentations. half sib chickens show therefore variation on AF. Accord- Case 3: Two independent variables affecting two different sets ing to the polygenic effect model of quantitative traits, of genes this variation is probably due to multiple mutations and Gene sets 70-170 and 171-271 are each affected by an biological processes. independent grouping variable marked respectively by Two lists of genes significantly correlated to the AF trait colors red and green and by colors orange and blue as are first generated using the raw and the factor-adjusted illustrated by Figure 1C. Two factors are identified by expression dataset. Then, the relevance of the two gene FAMT which are now interpreted regarding the two lists to characterize functionally fatness variation in the external sources of heterogeneity (Figure 2C). The red- family are compared, regarding the frequencies of biolog- green variable seems to be highly associated with the first ical processes related to the AF trait in their functional axis (P-value ≤ 2.2 × 10-16 in both representation). On the annotations. Factor-adjusted expression data is finally contrary, the orange-blue variable is not associated with used to identify a gene whose expression is controlled by this axis considering a significance level of 0.05 (P-value = the AF QTL region. 0.7933 for the individuals representation, p-value = Furthermore, the extracted factors are interpreted 0.1109 for the genes representation). The same strategy is using external information on the experimental design implemented for the second factor. The red-green vari- such as the hatch, dam and body weight and also gene able appears to be not associated with this factor (P-value information such as functional categories, oligonucle- = 0.7949 for the individuals representation, p-value = otide size and location on the microarray. It is deduced 0.1926 for the genes representation) whereas the orange- that some factors may have different and complex origins, blue variable is highly associated (P-value ≤ 2.2 × 10-16 in which confirms the importance of taking into account both representations). In this case, each of the two esti- these extra sources of variability to be more relevant in mated factors can be explained by one of the two inde- the transcriptomic analyses. pendent grouping variables. Results Analysis of the AF expression data set Illustrative Examples Classical approach Similarly to Storey et al (2007) [3], three simple situations Examination of the Pearson coefficient correlation of heterogeneity are considered. For each one, indepen- between hepatic transcript levels and AF trait shows that dent expressions for 1000 genes on 20 arrays are simu- 287 genes are significantly correlated considering a signif- lated according to a standard normal distribution. The icance threshold of 0.05 without any correction for multi- sample is split into two equal groups and a constant is ple tests. This low amount of differentially expressed genes might be explained by a poor genetic variability Blum et al. BMC Bioinformatics 2010, 11:368 Page 3 of 12 http://www.biomedcentral.com/1471-2105/11/368 Figure 1 Structure of the illustrative data sets. Representation of three illustrative studies consisting of 1000 genes on 20 arrays divided between two groups.
Details
-
File Typepdf
-
Upload Time-
-
Content LanguagesEnglish
-
Upload UserAnonymous/Not logged-in
-
File Pages12 Page
-
File Size-