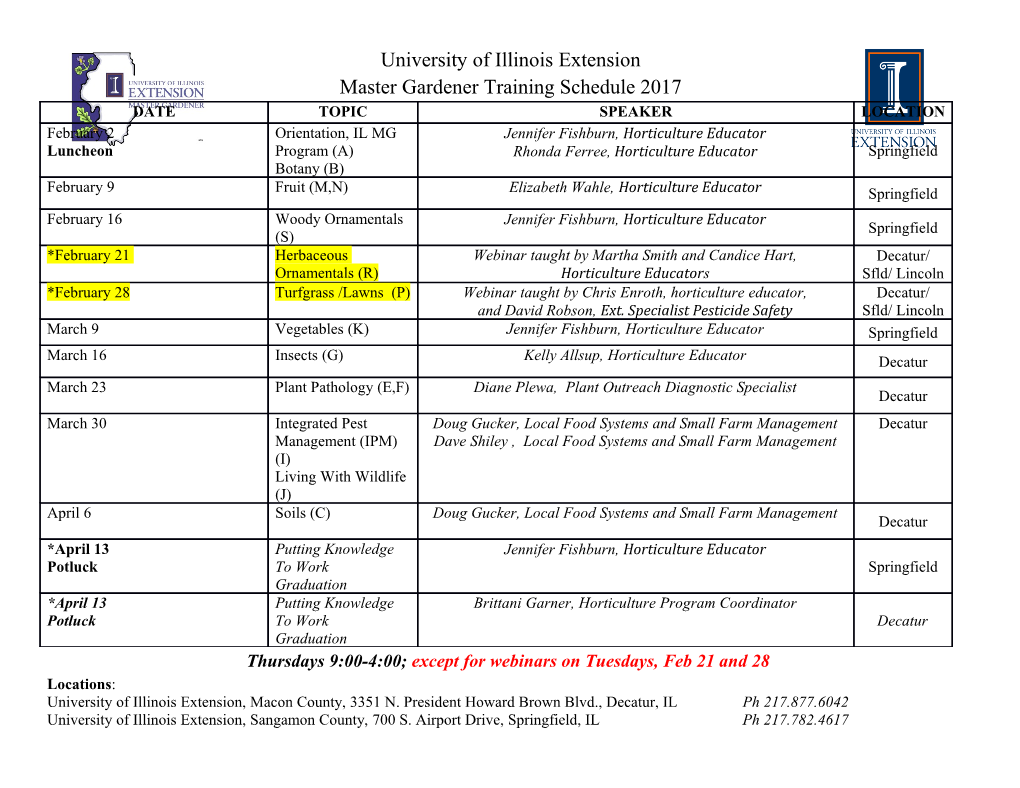
Statistical Machine Learning Introduction to R Niklas Wahlström Andreas Svensson Division of Systems and Control Department of Information Technology Uppsala University [email protected] [email protected] 1 / 47 Introduction to R [email protected], [email protected] About R I The programming language S developed at Bell laboratories in the 70’s I R appeared as an open source implementation of S in the 90’s I Today, there are thousands of available R packages I Widely used by statisticians 2 / 47 Introduction to R [email protected], [email protected] About R Ranked 7th most popular programming language in 2017 by IEEE http://spectrum.ieee.org/computing/software/the-2017-top-programming-languages 3 / 47 Introduction to R [email protected], [email protected] The R environment I R (download from http://cran.r-project.org/) I Graphical interface RStudio (open source, download from http://www.rstudio.com/products/rstudio/) I Alternatives: Emacs Speaks Statistics (ESS), Tinn-R, RKward, R Commander, . I Packages 4 / 47 Introduction to R [email protected], [email protected] RStudio 5 / 47 Introduction to R [email protected], [email protected] Outline About R R basics Vectors and matrices Scripts Plotting Implementing linear regression Data frames The linear regression command Working with data sets: Example Random number generation Control structures: for and if Functions 6 / 47 Introduction to R [email protected], [email protected] Outline About R R basics Vectors and matrices Scripts Plotting Implementing linear regression Data frames The linear regression command Working with data sets: Example Random number generation Control structures: for and if Functions 7 / 47 Introduction to R [email protected], [email protected] Variables You do not need to declare variable types in R. Native R syntax for creating a variable and assign a value to it: > x <- 2 (Alternative syntax: > x = 2) Variable types: numeric, integer, character, factor, logical Check type with class(), e.g., class(x) 8 / 47 Introduction to R [email protected], [email protected] Basics Add two numbers x = 2 + 2 > x <- 2 + 2 and print the result on the terminal > print(x) [1] 4 or > x [1] 4 9 / 47 Introduction to R [email protected], [email protected] Help resources I ? opens the help file for a command, e.g., > ?predict I ?? searches the entire R repository for a keyword, e.g., > ??predict I "Labs" at the end of each chapter in the ISL book I Internet I http://www.stats.ox.ac.uk/~evans/Rprog/LectureNotes.pdf I http://www.r-bloggers.com/ I http://www.ats.ucla.edu/stat/r/ I ... 10 / 47 Introduction to R [email protected], [email protected] Outline About R R basics Vectors and matrices Scripts Plotting Implementing linear regression Data frames The linear regression command Working with data sets: Example Random number generation Control structures: for and if Functions 11 / 47 Introduction to R [email protected], [email protected] Vectors 213 627 A vector v = 6 7 is written 435 4 > v <- c(1,2,3,4) > v [1] 1 2 3 4 12 / 47 Introduction to R [email protected], [email protected] Matrices 22 1 53 I A matrix A = 43 6 15 is written 4 2 4 > A <- cbind(c(2,3,4), c(1,6,2), c(5,1,4)) (cf. > B <- rbind(c(2,3,4), c(1,6,2), c(5,1,4))) or > u <- c(2,3,4,1,6,2,5,1,4) > C <- matrix(u,3,3) 21 1 13 I A matrix D = 41 1 15 is written 1 1 1 > D <- matrix(1,3,3) 13 / 47 Introduction to R [email protected], [email protected] Matrix indexing R uses 1 based indexes (not 0) I Consider the same matrix > A I Access second column: [,1] [,2] [,3] > A[,2] [1,] 2 1 5 [1] 2 3 4 [2,] 3 6 1 [3,] 4 2 4 I Access all but third column: > A[,-3] I Access element (1,2): [,1] [,2] > A[1,2] [1,] 2 1 [1] 1 [2,] 3 6 I Access first row: [3,] 4 2 > A[1,] [1] 2 1 5 14 / 47 Introduction to R [email protected], [email protected] Vector and matrix operations I Matrix transpose AT > t(A) I Matrix inverse A−1 > solve(A) I Elementwise multiplication Eij = AijCij > E <- A*C I Matrix multiplication F = AC > F <- A%*%C 15 / 47 Introduction to R [email protected], [email protected] Outline About R R basics Vectors and matrices Scripts Plotting Implementing linear regression Data frames The linear regression command Working with data sets: Example Random number generation Control structures: for and if Functions 16 / 47 Introduction to R [email protected], [email protected] R scripts A script works essentially as writing in the terminal, but you have everything saved and can easily go back and change things, and run it from the beginning again. To run a single line (or selected lines) in RStudio: Ctrl + Return To run the entire script: Ctrl + Shift + Return Use scripts!! 17 / 47 Introduction to R [email protected], [email protected] Outline About R R basics Vectors and matrices Scripts Plotting Implementing linear regression Data frames The linear regression command Working with data sets: Example Random number generation Control structures: for and if Functions 18 / 47 Introduction to R [email protected], [email protected] Plotting Now, we want to plot the following data describing the length of an infant at different ages Age (months) 0 6 12 18 24 Length (cm) 51 67 74 82 88 # Insert the data: > age <- c(0,6,12,18,24) > length <- c(51,67,74,82,88) # Plot data: > plot(x = age, y = length, col = 1, pch = 0, main="Infant length", xlab="age", ylab="length (cm)") # Add legend: > legend(x = "topleft", legend = "Data", col = 1, pch=0) 19 / 47 Introduction to R [email protected], [email protected] Outline About R R basics Vectors and matrices Scripts Plotting Implementing linear regression Data frames The linear regression command Working with data sets: Example Random number generation Control structures: for and if Functions 20 / 47 Introduction to R [email protected], [email protected] Linear regression example (I/II) I We would like to fit a straight line to the data with the model Y = β0 + β1X + "; X : age;Y : length I The normal equations 2 3 2 3 1 x1 y1 T −1 T 6 . 7 6 . 7 βb = (X X) X y where X = 4 . 5 ; y = 4 . 5 1 x5 y5 I # Solve normal equations > X <- cbind(matrix(1,5,1),age) > beta <- solve(t(X)%*%X)%*%t(X)%*%length 21 / 47 Introduction to R [email protected], [email protected] Linear regression example (II/II) I Compute the prediction according to Yb = βb0 + βb1X # Do predictions > lengthhat <- beta[1]+age*beta[2] I Plot a line corresponding to these predictions # Plot > lines(x=age,y=lengthhat,col=2, lty=1) > legend(x = "topleft", legend = c("Data","LR"), col = c(1,2), pch=c(0,NA), lty=c(NA,1)) I You need to keep track of the color and style of the lines for making the legend correct when using the built-in plot functions. (The popular package ggplot2 does it automatically, if you would prefer using that.) 22 / 47 Introduction to R [email protected], [email protected] Outline About R R basics Vectors and matrices Scripts Plotting Implementing linear regression Data frames The linear regression command Working with data sets: Example Random number generation Control structures: for and if Functions 23 / 47 Introduction to R [email protected], [email protected] Data frames (I/III) I Data frames can be used to store data tables. I # Create a data frame > infantdata <- data.frame(age,length) > infantdata age length 1 0 51 2 6 67 3 12 74 4 18 82 5 24 88 I Each column in a data frame may be of a different type, and may also have a descriptive name. 24 / 47 Introduction to R [email protected], [email protected] Data frames (II/III) I A data frame can be indexed with number or names I # Either ... # ... or # ... or > infantdata[1] > infantdata["age"] > infantdata$age age age [1] 0 6 12 18 24 1 0 1 0 2 6 2 6 3 12 3 12 4 18 4 18 5 24 5 24 I # Plot the data > plot(x = infantdata$age, y = infantdata$length, col = 1, pch = 0, main="Infant length", xlab="age", ylab="length (cm)") 25 / 47 Introduction to R [email protected], [email protected] Data frames (III/III) I Data frames are used by many high level commands in R, such as lm(), glm(), lda() and qda(). I Some commands (e.g., knn() and glmnet()), however, works with the matrix format instead, in which case you may need to do as.matrix(infantdata) etc. 26 / 47 Introduction to R [email protected], [email protected] Outline About R R basics Vectors and matrices Scripts Plotting Implementing linear regression Data frames The linear regression command Working with data sets: Example Random number generation Control structures: for and if Functions 27 / 47 Introduction to R [email protected], [email protected] The linear regression command lm (I/III) I Consider the same regression model as before Y = β0 + β1X + " where X is age and Y is length.
Details
-
File Typepdf
-
Upload Time-
-
Content LanguagesEnglish
-
Upload UserAnonymous/Not logged-in
-
File Pages47 Page
-
File Size-