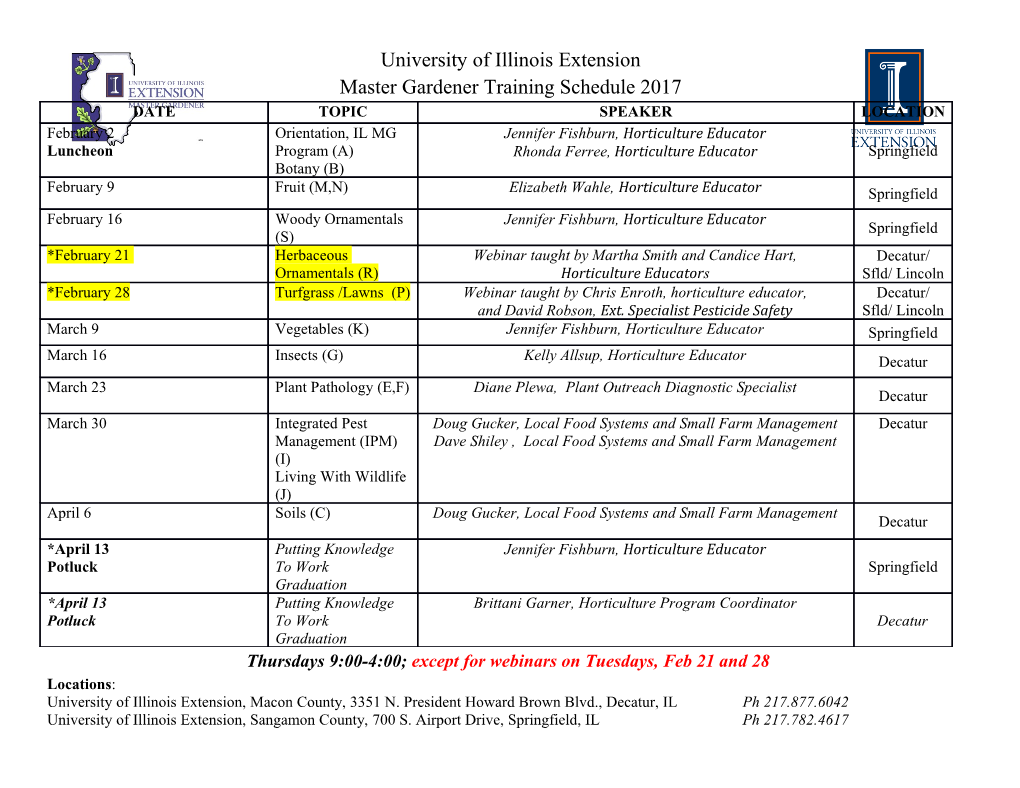
Predicting Music Popularity on Streaming Platforms Carlos V. S. Araujo1∗, Marco A. P. Cristo1 , Rafael Giusti1 1Federal University of Amazonas Manaus, Brazil {vicente, marco.cristo, rgiusti}@icomp.ufam.edu.br Abstract. Online streaming platforms have become one of American magazine Billboard, who has been evaluating the most important forms of music consumption. Most the music market since the 1940s. Some of the most fa- streaming platforms provide tools to assess the popular- mous rankings from this magazine are the Hot 100 Songs ity of a song in the forms of scores and rankings. In this and the Billboard 2004. The Billboard Hot 100 Songs list paper, we address two issues related to song popularity. ranks the most popular songs from each week, while the First, we predict whether an already popular song may at- latter ranks the most popular albums. Billboard also pro- tract higher-than-average public interest and become “vi- vides rankings that are specific to genres, countries, and ral”. Second, we predict whether sudden spikes in public distribution methods, as well as year-end rankings, which interest will translate into long-term popularity growth. We are versions of the previously mentioned charts, but for the base our findings in data from the streaming platform Spo- popularity of music and albums over the entire year [1]. tify and consider appearances in its “Most-Popular” list In spite of being fairly known to the general public, and as indicative of popularity, and appearances in its “Virals” also having been used for prediction of popularity in the list as indicative of interest growth. We approach the prob- past [2], Billboard charts are specific to the American mar- lem as a classification task and employ a Support Vector ket, and we do not employ them as data source in our work. Machine model built on popularity information to predict To assess the global market, we must focus on interest, and vice versa. We also verify if acoustic informa- platforms that provide worldwide service. According to tion can provide useful features for both tasks. Our results the IFPI, music streaming has become the most popular show that the popularity information alone is sufficient to method of music distribution2, finally surpassing physical predict future interest growth, achieving a F1-score above media in 2017. Streaming services also provide several 90% at predicting whether a song will be featured in the types of statistics concerning artists, albums, and scores, “Virals” list after being observed in the “Most-Popular”. which we may explore to measure worldwide popularity. We shall focus our attention on the streaming platform 1 Introduction Spotify, but we do note that our results may be extended to incorporate data from other streaming platforms, such The global entertainment market (movies, games, music, as Tidal and Apple Music. and television) is a billion-dollar industry. According to the Recording Industry Association of America – RIAA –, The problem of predicting success in the musi- in 2018 the music industry was worth US$ 9.8 billion in the cal market has been addressed in the literature before, with United States alone, 75% of which were due to streaming Machine Learning algorithms providing the best results in services and 11% to downloadable media1. Also according general. Some authors employed acoustic features to cre- to the International Federation of the Phonographic Indus- ate predictive models [3, 4], while others resorted to so- try – IFPI –, in the same year the global industry was worth cial network data [5, 6]. Our proposal relies only on his- US$ 17.3 billion, 38% of which were due to streaming ser- torical data of popularity, which we measure with the aid vices and 16% to downloadable media2. of streaming services, to predict the continued success or popularity growth of a song. The major reason for seek- It is no surprise that such market is fiercely com- ing a model that is free from acoustic data is that such data petitive. Wikipedia lists over 1,400 record labels in the may not always be available—Spotify, for instance, only United States alone3. In face of such competition, the un- provides a 30-second sample that may not be the most rep- derstanding of what makes an album or song successful is resentative segment of a song. While social network data key information. It could be used to plan better marketing may be very rich, its collection and preprocessing may be campaigns, to decide the best moment for the release of very labour-intensive and expensive. a new album or song, and to align the artists’ effort with public interest in, e.g., genre, theme, etc. The remainder of this paper is as follows. In sec- tion 2 we discuss related work, mostly focusing on previ- The success of an album or single song may be ous works that predicts popularity from music charts and assessed in several manners. The most common are proba- social network data. Spotify’s “Most-Popular” and “Vi- bly by means of rankings, such as those provided by the rals” lists are presented in Section 3. The methodology ∗Supported by CAPES. used at this research is described in section 4, and our re- 1www.riaa.com/wp-content/uploads/2019/02/RIAA- sults are presented in Section 5. Finally, in Section 6 we 2018-Year-End-Music-Industry-Revenue-Report.pdf draw our conclusions and discuss possibilities for future 2www.ifpi.org/downloads/GMR2018.pdf 3en.wikipedia.org/wiki/Category:American_ record_labels. Visited 2019-06-10. 4https://www.billboard.com/charts 17th Brazilian Symposium on Computer Music - SBCM 2019 141 work. ranking from the following sources between April 28th, 2013 and December 28th, 2014: Billboard, Last.fm, and 2 Related Work Spotify. Additionally, they retrieved data from songs of the albums in which these popular tracks were released. We have observed few popular approaches to the prob- This resulted in a data set of popularity scores and acous- lem of music success prediction, despite it having received tic information of 23,385 songs. Their goal was to employ growing attention for several years. We remark two gen- knowledge of the most successful songs from past albums eral strategies: the first uses social network data to assess to predict which song will be the most successful from current public perception and extrapolate how successful an unseen album. The authors employed two temporal- a song or album will be in the future. The second relies data models, namely a non-linear autoregressive network on acoustic information of previously successful songs to classifier (NAR) and its variation with exogenous inputs predict the success of a song or album. (NARX). The authors reported precision of 0.46 and accu- racy of . The work by Kim et al. [7] is an example of the 0 52 first strategy. The authors collected their data from the In addition to the previously discussed strategies, social network Twitter. Specifically, they analyzed posts Arakelyan et al. [10] compiled data from SongKick5 about (tweets) associated with the tags nowplaying, its shortened live performances and festivals. Their task was to predict version np, and itunes (Apple’s digital music sale plat- whether artists feature in those performances and festivals form). The task at hand was to predict whether a music would sign contracts with major record labels. They em- would be successful, which the authors defined as being ployed logistic regressors and reported precision of 0.39. featured among the 10 first entries in the Billboard Hot-100 Steininger and Gatzemeier [11] analyzed data from live ranking. Despite having found a correlation of only 0.41 performances in Germany and their task was to predict between the number of tweets and the number of weeks whether songs from the observed artists would be featured a song stayed in the ranking, the authors observed that a among the 500 most popular German songs in 2011. Using Random Forest classifier was able to achieve an accuracy Partial Least Squares Structural Equation Modeling (PLS- of 0.90. SEM), the authors reported an estimated precision of 0.43. Araujo et al. [8] also made use of Twitter data. The authors collected tweets with mentions to 15 popu- 3 Spotify’s Lists lar albums from 2016 and 2017. Their goal was to pre- dict the number of Billboard units achieved by the albums We collected our data from the streaming platform Spo- and also their popularity according to Spotify. A Billboard tify. Spotify is the third largest music streaming platform, 6 unit can be reached with a single physical or digital sale according to Forbes . Spotify publishes daily lists of pop- or with 1,500 music streams. Sentiment analysis was em- ular and “viral” songs which, according to Kevin Gold- 7 ployed to verify whether tweet mentions were positive or smith , a company’s former vice-president of engineering, negative. The authors observed linear correlation between are constructed in the following ways: the “Most-Popular” positive tweets and Spotify popularity, but no correlation list ranks songs according to the total number of streams in between negative tweets and Spotify popularity, neither be- the previous day, while the “Virals” list ranks song accord- tween tweets of any polarity and Billboard data. The au- ing to the growth in number of streams. thors hypothesized that Billboard’s choice of prioritizing From Goldsmith’s description we draw that a physical sales does not reflect the modern market. song will remain among the “Virals” if its number of lis- Among the works that resorted to acoustic data, teners is constantly rising, which implies that the song is Lee and Lee [3] observed 867 songs that made it to at least reaching a broader public than what is usual for a partic- three consecutive weeks in Billboard’s “Hot Rock Songs”, ular artist.
Details
-
File Typepdf
-
Upload Time-
-
Content LanguagesEnglish
-
Upload UserAnonymous/Not logged-in
-
File Pages8 Page
-
File Size-