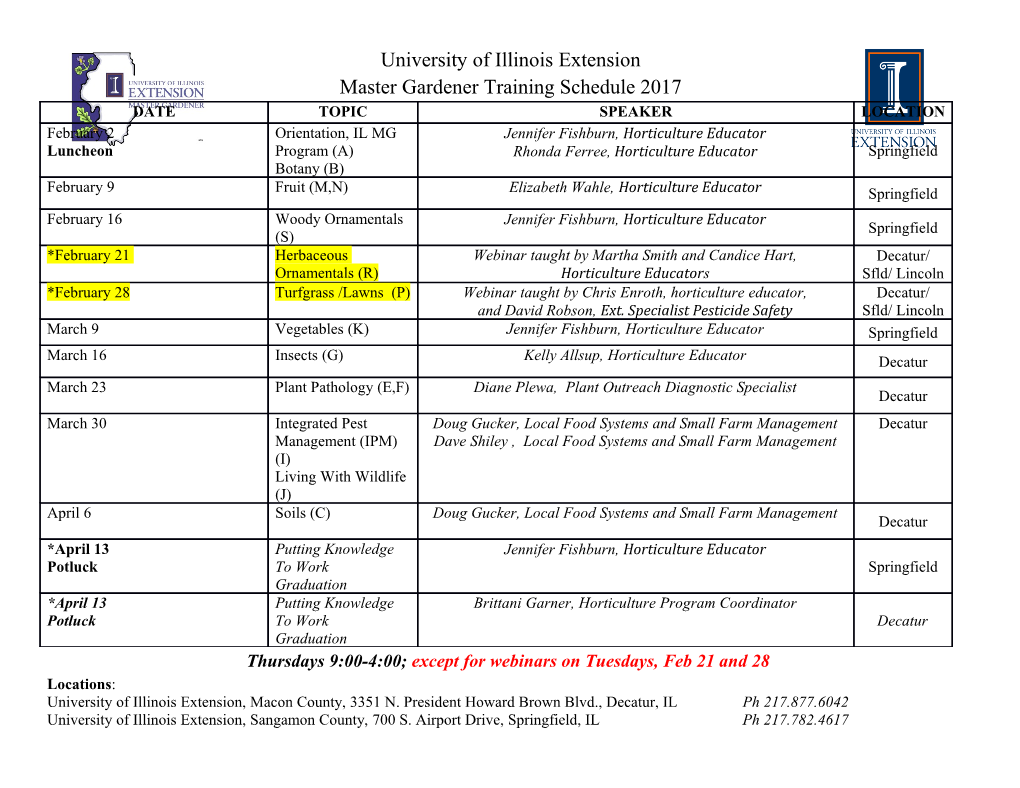
Parallel & Scalable Machine Learning Introduction to Machine Learning Algorithms Dr. Jenia Jitsev Head of Cross-Sectional Team Deep Learning (CST-DL) Scientific Lead Helmholtz AI Local (HAICU Local) Institute for Advanced Simulation (IAS) Juelich Supercomputing Center (JSC) @Jenia Jitsev LECTURE 9 Introduction to Deep Reinforcement Learning Feb 19th, 2020 JSC, Germany Machine Learning: Forms of Learning ● Supervised learning: correct responses Y for input data X are given; → Y “teacher” signal, correct “outcomes”, “labels” for the data X - usually : estimate unknown f: X→Y, y = f(x; W) - classical frameworks: classification, regression ● Unsupervised learning: only data X is given - find “hidden” structure, patterns in the data; - in general, estimate unknown probability density p(X) - in general : find a model that underlies / generates X - broad class of latent (“hidden”) variable models - classical frameworks: clustering, dimensionality reduction (e.g PCA) ● Reinforcement learning: data X, including (sparse) reward r(X) - discover actions a that maximize total future reward R t f a - active learning: experience X depends on choice of a h c s n i e - estimate p(a|X), p(r|X), V(X), Q(X,a) – future reward predictors m e G - z t l o h m l ● e H For all holds: r e d n i - Define a loss L(D,W), optimize by tuning free parameters W d e i l g t i M Deep Neural Networks: Forms of Learning ● Supervised learning: correct responses Y for input data X are given - find unknown f: y = f(x;W) or density p(Y|X;W) for data (x,y) - Deep CNN for visual object recognition (e.g, Inception, ResNet, ...) ● Unsupervised learning: only data X is given - general setting: estimate unknown density p(X;W) - find a model that underlies / generates X - broad class of latent (“hidden”) variable models - Variational Autoencoder (VAE), data generation and inference - Generative Adversarial Networks (GANs), data generation - Autoregressive models : PixelRNN, PixelCNN, ... ● Reinforcement learning: data X, including (sparse) reward r(X) - find predictors R = f(X;W) to estimate total future reward R(X) t f a - Deep Q-Learning Network (DQN – Atari games: Breakout, ...) h c s n i e - Deep Actor-Critic Networks (A3C, PPO, SAC, ...) m e G - z t l - Parts of AlphaGo; AlphaZero; AlphaFold, ... o h m l e H r e d n i → For all holds: d e i l g t i - Define a loss L(x,y,W), optimize by tuning parameters W M Mitglied in der Helmholtz-Gemeinschaft Deep Reinforcement Learning: breakthroughs Learning: Reinforcement Deep Mitglied in der Helmholtz-Gemeinschaft Deep Reinforcement Learning: breakthroughs Learning: Reinforcement Deep Mnih Mnih et al, Nature,2015 Mitglied in der Helmholtz-Gemeinschaft Deep neural networks: reinforcement learning neuralreinforcement networks: Deep Mnihet al, Nature, 2015 Mitglied in der Helmholtz-Gemeinschaft Deep Reinforcement Learning: breakthroughs Learning: Reinforcement Deep Mnih Mnih et al, Nature,2015 Mitglied in der Helmholtz-Gemeinschaft Deep neural networks: reinforcement learning neuralreinforcement networks: Deep Mitglied in der Helmholtz-Gemeinschaft Deep Learning: transforming the field transforming Learning: Deep Silver Silver et al,Nature, 2015 ● world championLee Sedol AlphaGowins against4:1 GO 18-time March2016: DeepMind Mitglied in der Helmholtz-Gemeinschaft Deep Reinforcement Learning: breakthroughs Learning: Reinforcement Deep Silver et Silver Nature,al, 2016 Mitglied in der Helmholtz-Gemeinschaft Deep Learning: transforming the field transforming Learning: Deep Silver et Silver Nature,al, 2017 ● ● Learning a function instead hardof wiring throughit previous insight AlphaGo:surpassing previous state artby the of dramatic margin Mitglied in der Helmholtz-Gemeinschaft Deep Reinforcement Learning: breakthroughs Learning: Reinforcement Deep Silver Silver et al, Nature,2017 that provide new of new insightsgames.” the oldest into provide that as well novelstrategies as knowledge, of this Go rasa days,starting few of a space books.In the collectively and distilledproverbs into patterns, of years, thousands millionsplayed games over of “ Humankind has accumulated Go knowledge from from knowledge Go accumulated has Humankind , AlphaGo Zero was able to rediscover rediscover much to was able Zero AlphaGo , tabula Deep Reinforcement Learning: breakthroughs ● Learning from self-play only – reward for winning games ● Human play data is no longer necessary for training Hardware Elo rating Matches Versions AlphaGo 176 GPUs,[2] 3,144[1] 5:0 against Fan Hui Fan distributed AlphaGo 48 TPUs,[2] 3,739[1] 4:1 against Lee distributed Lee Sedol AlphaGo 4 TPUs[2] v2, 4,858[1] 60:0 against Master single machine professional players; Future of Go Summit t f a h c s n i e AlphaGo 4 TPUs[2] v2, 5,185[1] 100:0 against m e G - z Zero single machine AlphaGo Lee t l o h m l e H 89:11 against r e d n i AlphaGo Master d e i l g t i M Silver et al, Nature, 2017 Mitglied in der Helmholtz-Gemeinschaft Deep Reinforcement Learning: breakthroughs Learning: Reinforcement Deep Silver et Silver Nature,al, 2017; Segler et al, Nature, 2018 ● (“bad”,“incorrect”) asoutcome some- states are desired (“good”, “correct”) or undesired suitable- for anytypeproblem of with statetransitions optimizationproblems (e.g,chemical synthesis, …) AlphaZero employsdeep neural networks:generic control / Chemistry Game... Mitglied in der Helmholtz-Gemeinschaft Deep Reinforcement Learning: breakthroughs Learning: Reinforcement Deep Evanset. al., 2018 ● AlphaFold Mitglied in der Helmholtz-Gemeinschaft Deep Reinforcement Learning: breakthroughs Learning: Reinforcement Deep ● AlphaStar Mitglied in der Helmholtz-Gemeinschaft Deep Reinforcement Learning: breakthroughs Learning: Reinforcement Deep Vinyals et al, Vinyals Nature,2019 Mitglied in der Helmholtz-Gemeinschaft Deep RL: JURECA RL: Deep /p/project/training2001/$USER/ cp -r/p/project/training2001/practicals_DeepRL folder: Copy DeepRL ( . python . python OpenAI Gym Environment: Testing . source . source . source . source the environment: Prepare cd variables variables /p/project/training2001/$USER/DeepRL /Test/test_opengym_pong_breakout.py /Test/test_opengym_CartPole.py /scripts/install_packages_DeepRL.sh /scripts/load_env_DeepRL.sh /scripts/init_env_DeepRL.sh /scripts/load_module_DeepRL.sh render , steps , episodes may be adapted accordingly) adapted be may (https://gym.openai.com) Deep Neural Networks: Forms of Learning ● Supervised learning: Find unknown f: y = f(x) for data (x,y) ● For each input x, correct example y is provided ● Define a loss L(x,y,W), optimize by tuning parameters W W1 . Wk . X . ... Y t f a h c s n i e m e G - z t l Unknown function: Y = f(X;W) o h m l e H Probabilistic view: unknown density P(Y|X,W) r e d n i d e i l g t i M Deep Neural Networks: Forms of Learning ● Supervised learning: Find unknown f: y = f(x) for data (x,y) ● For each input x, correct example y is provided ● Define a loss L(x,y,W), optimize by tuning parameters W W1 . Wk . X . ... Y t f a h c s n i e m e G - z t l Unknown function: Y = f(X;W) o h m l e H Probabilistic view: unknown density P(Y|X,W) r e d n i d Learning: Loss L(x,y,W) (defined over observed data; e i l g t i M e.g., gradient descent adapting W to minimize loss) Deep Neural Networks: Forms of Learning ● Learn y = f(x) from data (x,y) by adapting W ● However: delivering data with explicit specific teacher signal implausible or impractical (expensive labeling required) Z1 Zk W1 . Wk . X . ... Y t f a h c s n i e m e G - z t l Unknown function: Y = f(X;W) o h m l e H Probabilistic view: unknown density P(Y|X,W) r e d n i d Learning: Loss L(x,y,W) (defined over observed data; e i l g t i M gradient descent adapting W to minimize loss) Deep Neural Networks: Forms of Learning ● What if the correct output y for inputs x to the network is unknown? ● What are desired, relevant f(x) in this case anyway? Z1 Zk W1 . Wk . X . ... Y ? t ? f a h c s n i e m e G - z t l Unknown function: Y = f(X;W) o h m l e H Probabilistic view: unknown density P(Y|X,W) r e d n i d Learning: Loss L(x,y,W) (defined over observed data; e i l g t i M gradient descent adapting W to minimize loss) Mitglied in der Helmholtz-Gemeinschaft Reinforcement Learning Reinforcement ● ● (aboutstates, rewards, etc) predictions Predictionerror driven learning relevant consequences Reinforcementlearning: - using- “reward/punishment” signals defineto loss f( x ) and incoming observations updateto beliefs/expectations from of loss self-generatedactions L( x useavailable sensory andinput , W ) : use : mismatch (“good”/”bad”) extractto between L( x , own W ) Mitglied in der Helmholtz-Gemeinschaft Reinforcement Learning (RL) Learning Reinforcement Barto et al, 1983; et Niv 2006al, Reinforcement Learning (RL) Environment/ a) Tasks reward r Agent/Control X, s V(s,a)s) π(s,a)s,a)) sensory input Q(s,a)s,a)) action t f a ● h c MDP: Markov Decision Process < S, A, P, R, γ > s n i e m ● e Objective: “solve” towards optimal “policy” π(s,a)s,a)) - getting maximal G - z t l o h reward in the given environment m l e H r ● e For each state , select a response, action that is “optimal” d s a) n i d ● e i l Estimating Q(s,a)s,a)), π(s,a)s,a)), V(s,a)s) would provide full solution g t i M Barto et al, 1983; Niv et al, 2006 Mitglied in der Helmholtz-Gemeinschaft Deep Reinforcement Learning Reinforcement Deep ● ● Usenotion “good”,of “bad” outcome: “reward/punishment” signals correctWhatthe if output X W 1 Z . 1 y forinputs . W ... k Z .
Details
-
File Typepdf
-
Upload Time-
-
Content LanguagesEnglish
-
Upload UserAnonymous/Not logged-in
-
File Pages85 Page
-
File Size-