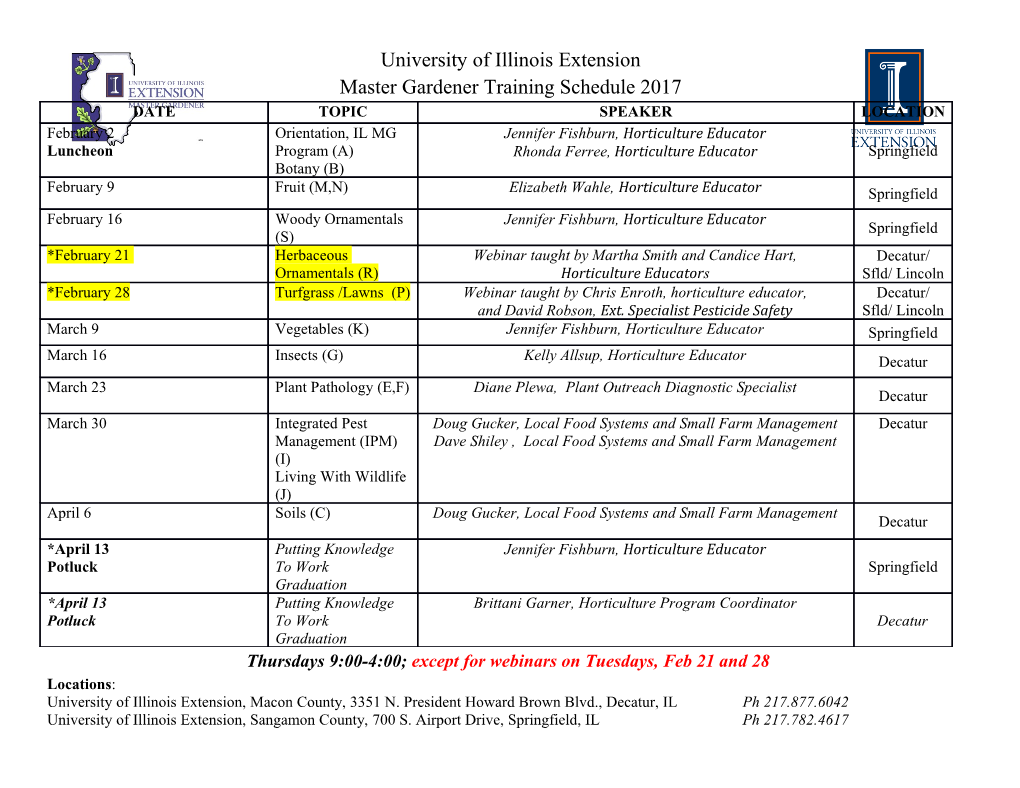
Journal of Multivariate Analysis MV1678 journal of multivariate analysis 62, 7499 (1997) article no. MV971678 Characterization of Multivariate Stationary Gaussian Reciprocal Diffusions* Bernard C. Levy- University of California, Davis Jamison's classification of scalar stationary Gaussian reciprocal processes is extended to multivariate Gaussian reciprocal diffusions (GRDs). The second-order self-adjoint differential equation satisfied by the covariance of a GRD specifies a Hamiltonian matrix whose eigenstructure is employed to parametrize the covariance of stationary GRDs. Characterizations of the conservation matrix and of View metadata,the covariance citation and matrix similar of thepapers end at point core.ac.uk values of a stationary GRD are also brought to you by CORE provided. 1997 Academic Press provided by Elsevier - Publisher Connector 1. INTRODUCTION Reciprocal processes were introduced in 1932 by Bernstein [2], who was influenced by an attempt of Schrodinger [3, 4] at developing a stochastic interpretation of quantum mechanics in terms of a class of Markov pro- cesses for which boundary conditions are imposed at both ends of the inter- val of definition. A stochastic process x(t)onI=[0, T] taking values in Rn is called reciprocal if for any two times t0<t1 of I, the process interior to [t0 , t1] is conditionally independent of the process exterior to [t0 , t1], given x(t0) and x(t1). Reciprocal processes contain Markov processes as a subclass. Also, Markov random fields in the sense of Levy [5] reduce for the case of a single parameter to reciprocal, rather than Markov, processes. The properties of reciprocal processes have been examined in detail by a number of authors, and in particular Jamison [1, 6], who obtained, among other results, a classification of all stationary scalar Gaussian reciprocal Received July 11, 1995. AMS subject classification numbers: primary 60G10, 60G15, 60G60, 60J60; secondary 15A21. Key words and phrases: Gaussian reciprocal diffusions, Hamiltonian matrix, stationary processes. * The research described in this paper was supported by the Office of Naval Research under Grant USN-N00014-89-J-3153. - E-mail: levyÄece.ucdavis.edu. 74 0047-259XÂ97 25.00 Copyright 1997 by Academic Press All rights of reproduction in any form reserved. File: 683J 167801 . By:CV . Date:14:07:01 . Time:10:44 LOP8M. V8.0. Page 01:01 Codes: 3862 Signs: 2167 . Length: 50 pic 3 pts, 212 mm MULTIVARIATE STATIONARY GRDs 75 processes over a finite interval. However, this classification turned out to be incomplete and was subsequently amended first by Chay [7], and then by Carmichael, Masse, and Theodorescu [8]. Other studies of Gaussian reciprocal processes include [914]. The goal of the present paper is to extend Jamison's classification to the class of multivariate Gaussian reciprocal diffusions (GRDs) introduced in [12, 15]. We recall that a zero-mean Gaussian process x(t) is a Gaussian reciprocal diffusion if it satisfies the following four axioms. A1. x(t) is a zero-mean Gaussian reciprocal process over [0, T] taking values in Rn, whose sample paths are continuous almost surely. A2. Let R(t, s)=E[x(t) xT(s)] be the covariance of x(t), where ``T'' denotes the matrix transpose. R(t, s)isC2over the triangle 0stT,in the sense that continuous limits of R and its first and second derivatives exist on the closure of this triangle. A3. For 0<t0<t1<T, the two time covariance matrix R(t , t ) R(t , t ) 0 0 0 1 (1.1) _R(t1 , t0) R(t1 , t1)& is invertible. A4. The matrix R R Q(t)= (t&, t)& (t+, t) (1.2) t t is positive definite for all t. Assumption A4 ensures that the diffusion metric of x(t) is nondegenerate. This assumption excludes from consideration higher-order reciprocal pro- cesses of the type examined in [1618], which all have a singular diffusion metric. It was shown in [12, 15] that if x(t) is a GRD, its covariance R(t, s) satisfies a second-order self-adjoint differential equation, LR(t, s)=In$(t&s), (1.3) where L takes the form 2 &1 d d L=Q (t) & 2+G(t) +F(t) (1.4) _ dt dt & File: 683J 167802 . By:CV . Date:14:07:01 . Time:10:44 LOP8M. V8.0. Page 01:01 Codes: 2311 Signs: 1564 . Length: 45 pic 0 pts, 190 mm 76 BERNARD C. LEVY with 2 2 R + R & &1 G(t)=& 2 (t , t)& 2 (t , t) Q (t) (1.5a) _t t & 2 R + R + &1 F(t)= 2 (t , t)&G(t) (t , t) R (t, t). (1.5b) _t t & The formal differential operator L is self-adjoint, so that the matrix func- tions F and G satisfy the constraints 1 d (Q&1G+GTQ&1)=& (Q&1) (1.6a) 2 dt 1 d Q&1F&F TQ&1= (Q&1G&GTQ&1). (1.6b) 2 dt Together with the differential equation (1.3), we can apply either Dirichlet conditions R(0, 0) R(0, T) =P, (1.7) _T(T,0) R(T, T)& where P is a symmetric nonnegative definite matrix, or cyclic boundary conditions of the type discussed in [15]. In this paper we restrict our atten- tion to the Dirichlet case. Also, if 1 R R V(t)= (t+, t)+ (t&, t) R&1(t, t) (1.8a) 2 _t t & 1 2R 2R ?(t)= (t+, t)+ (t&, t) &V(t) R(t, t) VT(t), (1.8b) 2 _t s t s & the matrix R(t, t) R(t, t) VT(t) 0(t)= T (1.9) _V(t) R(t, t) ?(t)+V(t) R(t, t) V (t)& satisfies the conservation law d 0=H0+0HT, (1.10) dt File: 683J 167803 . By:CV . Date:14:07:01 . Time:10:44 LOP8M. V8.0. Page 01:01 Codes: 1998 Signs: 818 . Length: 45 pic 0 pts, 190 mm MULTIVARIATE STATIONARY GRDs 77 where H denotes the Hamiltonian matrix 0 I H(t)= n . (1.11) _F(t) G(t)& The conservation law (1.10) plays the same role for GRDs as the Lyapunov equation used to propagate the covariance matrix of Gaussian Markov diffusions (GMDs). However, for GRDs, more quantities need to be propagated; in addition to the covariance matrix P(t)=R(t, t), we must propagate the matrix V(t), which specifies the mean velocity v(x, t)= V(t) x of the diffusion, and the stress tensor ?(t). Both of these quantities arise in descriptions of the kinematics of reciprocal diffusions, which are studied for the general case of non-Gaussian reciprocal diffusions in [19, 20]. A complete analysis of the conservation laws of GRDs can be found in [21, 22]. In this paper, we examine the restrictions which are imposed on the above model when x(t)isstationary, i.e., when R(t, s)=R(t&s). Clearly, from (1.1), (1.5a), and (1.5b), the matrices Q(t)=Q, F(t)=F and G(t)=G must be constant, so that the self-adjointness conditions (1.6a)(1.6b) reduce to Q&1G+GTQ&1=0 (1.12a) Q&1F&F TQ&1 =0. (1.12b) By observing that we can always perform the transformation z(t)= Q&1Â2x(t), where Q1Â2 is an arbitrary square-root of Q, we can assume without loss of generality that Q=In , in which case (1.12a)(1.12b) to reduce to G=&GT, F=F T, (1.13) so that G and F are respectively skew-symmetric and symmetric. The expressions (1.8a)(1.8b) also show that when x(t) is stationary, the matrices V, ?, and 0 are constant, so that the conservation law (1.10) reduces to the algebraic equation H0+0HT=0. (1.14) It is assumed in the following that d 2 d L=&I +G +F (1.15) n dt2 dt File: 683J 167804 . By:CV . Date:14:07:01 . Time:10:44 LOP8M. V8.0. Page 01:01 Codes: 2397 Signs: 1635 . Length: 45 pic 0 pts, 190 mm 78 BERNARD C. LEVY is given, and we seek to characterize the constraints imposed on P and 0 by the requirement that x(t) must be stationary. Specifically, we seek to characterize all the stationary GRDs belonging to the reciprocal equivalence class specified by L (two GRDs are said to be reciprocally equivalent [23] if they admit the same L). At the same time, we will obtain a representation of the covariance functions R( } ) of stationary GRDs which extends Jamison's classification of scalar stationary GRDs. Jamison's classification can be described as follows. First, observe that in the scalar case G is necessarily zero, so that R(t) satisfies the differential equation R (t)=FR(t). (1.16) Then, depending on whether F is positive, zero, or negative, three cases can occur. Case 1. For F=a2>0 with a>0, the covariance R(t) can be expressed as 1 R(t)= (A cosh(at)&sinh(at)) (1.17) 2a with A>0. We then have three subcases. (i) For A>1, we get the hyperbolic cosine process 1 cosh(a(TÂ2&|t|)) R(t)= (1.18a) 2a sinh(aTÂ2) with 0|t|T, where T denotes the lifetime of the process x(t), and coth(aTÂ2)=A. (1.18b) This process is periodic, since x(T)=x(0). (ii) A=1 gives the OrnsteinUhlenbeck process 1 R(t)= exp(&at), (1.19) 2a which is Markov. (iii) For A<1, we obtain the hyperbolic sine process, 1 sinh(a(TÂ2&|t|)) R(t)= (1.20a) 2a cosh(aTÂ2) File: 683J 167805 .
Details
-
File Typepdf
-
Upload Time-
-
Content LanguagesEnglish
-
Upload UserAnonymous/Not logged-in
-
File Pages26 Page
-
File Size-