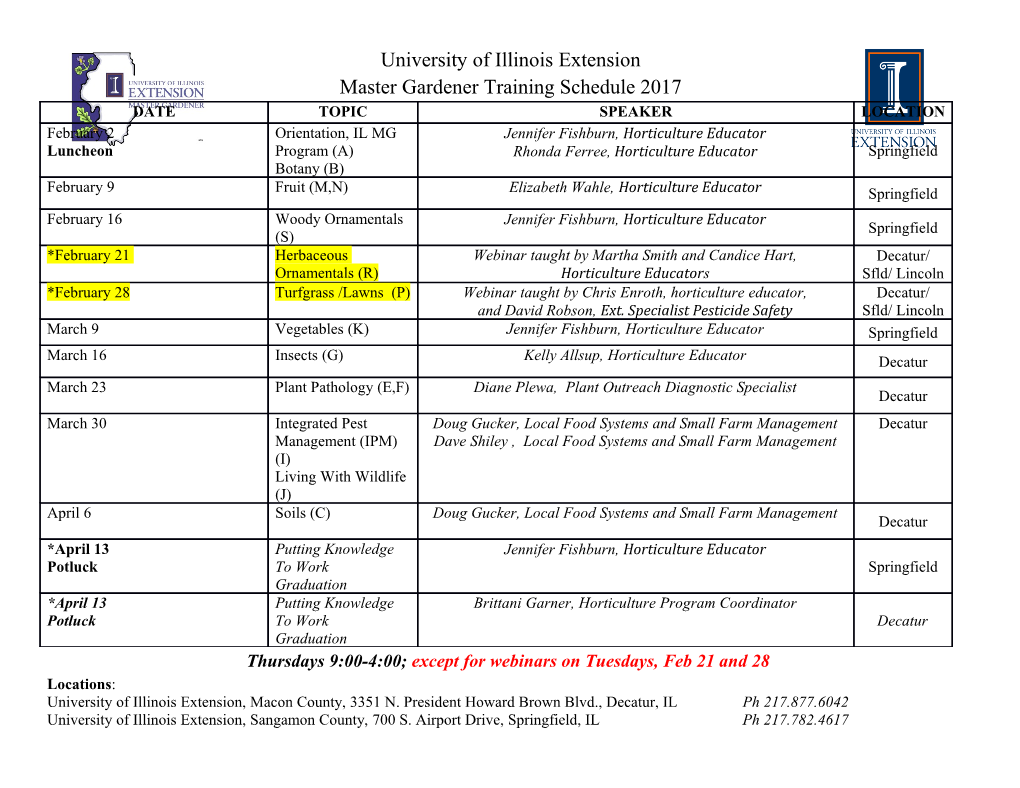
Foundations and TrendsR in Marketing Vol. 4, No. 3 (2009) 129–207 c 2010 D. Bowman and H. Gatignon DOI: 10.1561/1700000015 Market Response and Marketing Mix Models: Trends and Research Opportunities By Douglas Bowman and Hubert Gatignon Contents 1 Introduction 130 2 Stimulus or Inputs 134 2.1 “New” (or Under-Studied) Inputs 134 2.2 ‘Richer’ Measures of Inputs Constructs 138 2.3 Summary: Stimulus or Inputs 141 3 Intervening Factors: Explicitly Accounting for the Process Linking Inputs to Outputs 142 3.1 Chain-Link or Cascading Frameworks 142 3.2 Hierarchical Models 144 3.3 Accounting for Spatial Aspects in Market Response 145 3.4 Data Fusion 147 3.5 Summary: Explicitly Accounting for the Process Linking Inputs to Outputs 148 4 Response or Output: “New” or Under-Studied Dependent Variables 149 4.1 Financial Variables 149 4.2 Under-Studied Levels of Aggregation 162 4.3 Better Accounting of Processes That Do Not End in a Purchase 165 4.4 Intangible-Dependent Variables 167 4.5 Summary: New or Under-studied Dependent Variables 168 5 Under-Studied or Emerging Contexts 169 5.1 Increasing Receptivity for Some Industry-Specific Contexts 169 5.2 Marketing in a Downturn Economy 175 5.3 Across-Country and Spatial Market Response Models 179 5.4 Accounting for New (Internet) and Multichannel Contexts 182 5.5 Business Market Response Models 187 5.6 Summary: Under-Studied or Emerging Contexts 188 6 Conclusions 189 References 191 Foundations and TrendsR in Marketing Vol. 4, No. 3 (2009) 129–207 c 2010 D. Bowman and H. Gatignon DOI: 10.1561/1700000015 Market Response and Marketing Mix Models: Trends and Research Opportunities Douglas Bowman1 and Hubert Gatignon2 1 Emory University, Atlanta, GA 30322, USA, Doug [email protected] 2 INSEAD, Boulevard de Constance, 77305, Fontainebleau, France, [email protected] Abstract Market response models help managers understand how customers col- lectively respond to marketing activities, and how competitors interact. When appropriately estimated, market response models can be a basis for improved marketing decision-making. Market response models can be broadly classified as: (a) those directly linking marketing stimuli or more generally relevant inputs to market response outputs; and (b) those that also model a mediating process. Inputs include mar- keting instruments (i.e., marketing mix variables) and environmental variables. This monograph takes a forward-looking perspective, includ- ing trends, to identify research opportunities related to market response and marketing mix models as falling under four broad areas: (1) “New” or under-studied inputs and/or “richer” measures of inputs constructs; (2) Explicitly accounting for the process linking inputs to outputs; (3) “New” or under-studied dependent variables; and (4) Under-studied or emerging contexts. 1 Introduction As quantitative information about markets and marketing actions becomes more widely available, modern marketing is presented with both a challenge and an opportunity: how to analyze this informa- tion accurately and efficiently, and how to use it to enhance market- ing productivity. Marketing response models are tools for achieving these objectives. They relate variables that describe actions available to managers (i.e., the marketing mix), and variables that describe the environment, to performance outputs. Table 1.1. Market response models. 130 Table 1.2. Summary of trends and research opportunities. Representative studies Research opportunities Stimulus or inputs New (or Emerging aspects Stephen and Galak (2009); Including variables describing under-studied) of marketing Stephen and Toubia (2010); digital marketing (social input variables Trusov et al. (2009) media, paid search, etc.) as additional explanatory variables. Relative Bowman and Narayandas Word-of-mouth importance was (2004) thought to be minimal Effort to collect Bolton et al. (2006) Variables describing actions measures outside the traditional thought not to purview of marketing; be worth the Public relations benefit “Richer” measures Refinements in Hui et al. (2009a); Distribution measures that of inputs construct Hui et al. (2009b) better account for in-store constructs measurement merchandising Neglected aspects Bass et al. (2007) Accounting for message content, of a given not just effort construct Decomposition of Fader and Hardie (1996); Disaggregating advertising into constructs Fang et al. (2008); media type Naik and Raman (2003); Sorescu and Spanjol (2008) Intervening factors Chain-link or Service-profit chain Bowman and Narayandas Dynamics cascading (2004); frameworks Heskett et al. (1994); Kamakura et al. (2002) Brand-value chain Keller and Lehmann (2003) Dynamics 131 (Continued) 132 Table 1.2. (Continued) Representative studies Research opportunities Introduction Hierarchical Inman et al. (2009) Better accounting for nested models structures in data Spatial models Bronnenberg and Sismeiro Better accounting for spatial (2002); aspects of data Choi et al. (2010) Data fusion Gauri et al. (2008); Insights from merging Horsky et al. (2006) behavioral and mindset data Response or Financial variables Profit Boulding and Christen (2003); Decomposition into various output Christen and Gatignon (2009) elements under managerial control Stock market Srinivasan and Hanssens (2009) Augmenting model from the valuation finance literature to include variables that describe marketing strategies and tactics Under-studied Geographic Bronnenberg et al. (2006); Richer accounting for the role levels of Ataman et al. (2007) played by distribution aggregation Aggregate versus Chen and Yang (2007); Structural models that better individual-level Musalem et al. (2008) accommodate a larger number of explanatory variables Process that do Briesch et al. (2008) Combining insights from not end in van Heerde et al. (2008); purchase and non-purchase purchase Smith et al. (2006) events; Measuring processes that do not end in a purchase Intangible- Slotegraaf and Pauwels (2008); More comprehensive models dependent Villanueva and Hanssens (2007) that better account for the variables underlying processes 133 This monograph takes a forward-looking perspective, including trends, to identify research opportunities related to market response and marketing mix models as falling under four broad areas: (1) “New” or under-studied inputs and/or “richer” measures of inputs constructs; (2) Explicitly accounting for the process linking inputs to outputs; (3) “New” or under-studied-dependent variables; and (4) Under-studied or emerging contexts. Table 1.1 presents the process linking inputs to outputs that is the framework for the monograph. Table 1.2 presents a representative sample of research and future research opportunity for each of the four sections. Each of the following sections will cover three broad areas related to marketing mix models: data issues and requirements; methodologies (i.e., traditional econometrics; Bayesian methods; structural models); and substantive findings. 2 Stimulus or Inputs Stimulus or inputs include marketing instruments (i.e., marketing mix variables) and environmental variables. We identify opportuni- ties broadly related to two aspects of inputs: new (or under-studied) input variables and “richer” measures of inputs constructs. The former include (1) variables that describe actions that only recently have been used by marketers such as many aspects of interactive marketing; and (2) variables that have been neglected because their relative importance was thought to be minimal, or the effort to collect measures was not deemed to be worth the benefit from including them in a model. The latter include (1) refinements in construct measurement, (2) neglected aspects of a given construct, and (3) decomposition of constructs. 2.1 “New” (or Under-Studied) Inputs Parsimony is a key objective of a model-building effort, implying pru- dence in decisions to expand the number of explanatory variables included in a model. As Farley et al. (1998) note, simply “including vari- ables that have been measured infrequently is not necessarily optimal . [r]educing collinearity among design variables is the key to improved knowledge”. 134 2.1 “New” (or Under-Studied) Inputs 135 The first papers to investigate the effects of new or under-studied variables typically seek to establish a main-effect result, follow-on research then focuses on identifying contingencies. With respect to con- tingencies, conceptually it can be useful to distinguish between situa- tions where the new variable is viewed as a component of the base response function with the goal being to specify a process function that describes contingent effects for its parameter, Performance = βk(i)New Variable + ··· (response function); βk(i)=f(Contingency #1,...) (process function). And, situations where the new variable is viewed as a component of a process function of a well-established response function variable, Performance = βk(i)Price + ··· (response function); βk(i)=g(New Variable,...) (process function). Consider for example, research studying effects due to order-of-entry into a market: early papers focused on establishing an automatic reg- ularity due to order-of-entry (e.g., Robinson and Fornell, 1985); later papers first concentrated on identifying situations where the main effect of order-of-entry was accentuated or attenuated (e.g., consumer ver- sus industrial markets; Robinson, 1988), and then examined situations where order-of-entry was part of a process function that described vari- ations in responsiveness to often-studied
Details
-
File Typepdf
-
Upload Time-
-
Content LanguagesEnglish
-
Upload UserAnonymous/Not logged-in
-
File Pages81 Page
-
File Size-