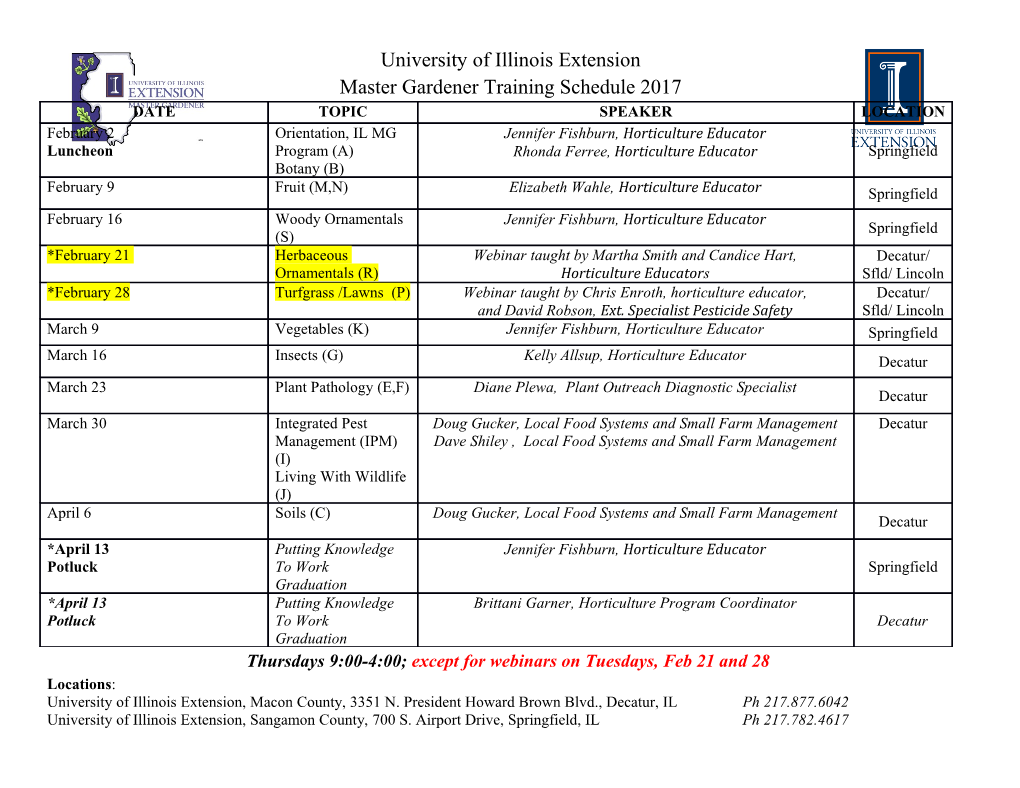
Tropical Ecology 59(3): 457–472, 2018 ISSN 0564-3295 © International Society for Tropical Ecology www.tropecol.com Variation in biomass and carbon allocation in various components of tree species along different forest types in high mountain regions 1 1,2* 1 1 1 RAM KRISHAN , ASHISH K. MISHRA , OM PRAKASH TIWARI , Y. S. RANA & C. M. SHARMA 1Department of Botany, HNB Garhwal University, Srinagar Garhwal-246174, Uttarakhand, India 2Department of Environmental Science, Babasaheb Bhimrao Ambedkar (Central) University, Vidya Vihar Raebareli Road, Lucknow-226025, Uttar Pradesh, India Abstract: Live component utility of carbon discrimination play a vital role in forest carbon cycle through photosynthesis and actively help in mitigation of global warming. The present study was conducted in different mountain forests (situated between 1428-3460 m asl) in Garhwal Himalaya. The aim of this study is to present an assessment of the carbon stock variation in tree biomass purposing recommendations for future forest management. Eight temperate forest types viz., Abies spectabilis forest, Betula utilis forest, Cedrus deodara forest, mixed coniferous forest, Pinus wallichiana forest, Quercus floribunda forest, Q. leucotrichophora, and Q. semecarpifolia forests were studied to enumerate its growing stocks, biomass density and carbon stocks of the area. The mean Growing Stock Volume Density (GSVD) was calculated as 488±39.11 m3 ha-1, which ranged from 366.68±71.27 m3 ha-1 (Q. floribunda forest) to 676.38±155.19 m3 ha-1 (Cedrus deodara forests). The values of total biomass density (TBD) was recorded the highest for Abies forests (636.3±64.6 Mg ha-1) and the lowest for Betula forests (286.37±29.9 Mg ha-1). Highest total carbon density was recorded for Abies spectabilis forest (292.7±29.72 Mg C ha-1) followed by Q. floribunda forest (265.19±18.41 C Mg ha-1) and the lowest in Betula forest (131.73±13.75 Mg C ha-1). The study revealed that the conifer-dominated forest types had higher carbon storage potential than broadleaf-dominated forest types. Therefore, results will be useful for conservation practices and their plantation which should be necessary for controlling the greenhouse effect and climate change. Key words: Climate change; forest carbon; Garhwal Himalaya; live tree biomass. Introduction (IPCC 2003). Quantification of biomass and carbon storage has recently become important all over the Concern over global climate change related to world and is presently recognised as an important increasing carbon dioxide (CO2) and the enhanced component in the implementation of the emerging C greenhouse effect has encouraged the detail study of credit market mechanism (Chave et al. 2004). The carbon (C) balance in the ecosystem. The increase in quantity of biomass in a forest determines the greenhouse gases is projected to lead to 1–3.5 ºC in potential amount of C that can be added to the global mean surface temperature by 2100 (IPCC atmosphere and managed for meeting emission 1996). However, a common framework and good targets (Brown et al. 1999). Forests have a unique practice guidance for C reporting through biomass status in the whole scenario of climate change, as equation has been recognised by United Nations they act as a sink as well as source of carbon and *Corresponding Author; e-mail: [email protected] 458 BIOMASS AND CARBON ALLOCATION IN TREE SPECIES IN HIGH MOUNTAIN FORESTS are valued globally for the services they provide to to assess the role of climate change in predicting the society. Forest ecosystems contain the majority effect on future species coexistence and shift in (approximately 60%) of the C stored in terrestrial Himalayan range (Sharma et al. 2016a, b). ecosystems (IPCC 2000). The main carbon pools in In earlier studies from Uttarakhand, various forests include plant biomass (above and below researchers from Kumoun Himalaya (Adhikari et ground), coarse woody debris, litter and soil. In al. 1995; Chaturvedi & Singh 1987; Rawat & most forested ecosystems, the majority of the carbon Singh 1988) and Garhwal Himalaya (Gairola et al. in biomass is stored below ground as dead organic 2011; Sharma et al. 2010, 2011) have attempted to matter (DOM). Within forest biomes as a whole, predict biomass and carbon stocks. Biomass 68% of the total forest carbon is in the DOM pool i.e. content of different components of tree were earlier 50% in tropical forests, 63% in temperate forests, studied from Kumaun division but not reported and 84% in the boreal forests (Kimble et al. 2003). A from Garhwal region. In addition to this, an up-to- better understanding of C changes is critical for date quantification of growing stock is necessary projecting future atmospheric CO2 growth and for better management, planning and decision guiding the design and implementation of making, as forests are source of timber and non mitigation policies. timber forest products (NTFPs). This paper aims In India total 34651 km2 (about 4.49% of the at (i) the assessment of tree diversity, growing country) areas occupied by forest cover of stocks volume, component wise contribution of Uttarakhand, while 38.52% of forest area is located biomass accumulation of tree species and their in temperate zone which is a major portion in the carbon stocks in the different forests of temperate Garhwal Himalaya (FSI 2013). Hence region of Uttarkashi district of Garhwal Himalaya, understanding of biomass and carbon potential of and at the assessment of (ii) altitudinal and these forests is seems to be essential. Although, the climatic effects on these forests. carbon pool of a Himalayan forest ecosystems varies Material and methods within the different forest (Sharma et al. 2010; Zhang et al. 2013) and along altitude (Zhu et al. Study area 2010). The Garhwal Himalayas contains a wide The Uttarakhand state is located in North- range of rich vegetation from dry tropical to West part of the country. Its geographical area is subalpine forests. However, conifer and oak forests 53,483 km2 which constitutes 1.63% of total area of dominantly occupy ridges, which constituted the the country (FSI 2013). This study was conducted major part of total forest area. In forest ecosystems, in several mountain ranges in temperate zone of trees produce and maintain the overall physical Bhagirathi catchment area (between 1428–3460 m structure of habitats, and thus, define funda- asl) in Uttarkashi district of Garhwal Himalaya mentally the templates for structural complexity (India). The district Uttarkashi is the largest and environmental heterogeneity (Jones et al. district of Uttarakhand occupying an area of about 1997). Various factors like climate, stand age, 8016 km2 and is situated at latitude 30º13’-30º14’ disturbance regimes, and edaphic conditions are N and longitude 78º47’–78º43’ E (Fig. 1). Out of sturdily affected the carbon pools in forest total area, only 39.23% of area covered with forest ecosystems (Pregitzer & Euskirchen 2004). But the cover (FSI 2013). The average temperature of the most important predictors of aboveground biomass study area is 18.8 ºC and the average rainfall is (AGB) of a tree are, in the order of less importance; 1693 mm, the maximum rainfall was recorded in its trunk diameter, wood specific gravity, total the months of June to August. The climatic height and forest type (Chave et al. 2005). Globally, variables including temperature and rainfall of the temperate forest is a widely distributed forest type, area observed during the year were estimated by and its carbon flux has significantly altered by the data published by Indian Meteorological changing climate (Dai et al. 2013). However, the Department, Uttarkashi for the year 1901-2002 carbon flux of coniferous forests has been more (Fig. 2). After the reconnaissance survey of the strongly influenced by climate change as compared study area, we selected eight dominant forest to deciduous forests (Ma et al. 2014). types and named according to the classification Composition of forest vegetation and its given by Champion & Seth (1968), viz. analysis can effectively predict the influence of climate change on migration of woody species. (i) Abies spectabilis forest (AF) - West Hence, quantification of the current forest Himalayan Sub-alpine Fir Forest (14/C1a) composition and carbon dynamics is crucial in order (ii) Betula utilis mixed forest (BF) - West Hima- RAM KRISHAN et al. 459 Fig. 1. Map of district Uttarkashi with studied forest types. (iv) Pure Pinus wallichiana forest (PF) - Pine Forest (9/C1b) (v) Mixed conifer forest (MCF) - West Himalayan mixed coniferous forests (12/C2) (vi) Quercus floribunda forest (QFF) - Moru Oak Forest (12/C1b) (vii) Quercus leucotrichophora forest (QLF) - Banj Oak forest (12/C1a) (viii) Quercus semecarpifolia forest (QSF) - West Himalayan Upper Oak Forest (12/C2b) Selected forest types were undisturbed and exist on inherently different types of woody plants in sub-alpine vegetation. Fig. 2. Meteorological data of district Uttarkashi Vegetation analysis (1901-2002) (source: Indian Meterological Department, Uttarkashi). To analyse the forest vegetation on different ridge tops, 10 sample plots of 0.1 ha each were laid layan Sub-Alpine Birch/Fir Forest (14/C1b) out in eight selected ridge tops of each forest types (iii) Cedrus deodara forest (CF) - Dry Deodar (10 plots (2 plots in each ridge top × 5 ridge tops) × Forest (13/C2b) 08 forest types = total 80 sample plots). All indivi- 460 BIOMASS AND CARBON ALLOCATION IN TREE SPECIES IN HIGH MOUNTAIN FORESTS Table 1. Details of elevation, SR, TBC, Density, GSVD, TBD and TCD values in different forest types. Forest types Elevation SR TBC Density GSVD TBD TCD (m asl.) (m2 ha-1) (ha-1) (m3 ha-1) (Mg ha-1) (Mg C ha-1) Abies spectabilis 2580–3460 10 20.73 778±89.97 602.28±69.93 636.30±64.6 292.70±29.72 Betula utilis 3220–3460 6 13.44 498±36.11 406.39±28.67 286.37±29.9 131.73±13.75 Cedrus deodara 2425–3125 9 21.18 592±94.41 676.38±155.19 366.13±59.1 168.42±27.19 Mixed Coniferous 2072–2737 14 22.73 610±48.68 550.83±76.03 570.82±102.5 262.58±47.15 Pinus wallichiana 2577–3252 13 14.69 560±69.93 446.51±70.86 355.38±30.6 163.47±14.08 Quercus floribunda 2530–2690 12 14.61 662±18.81 366.68±71.21 589.30±40.9 265.19±18.41 Q.
Details
-
File Typepdf
-
Upload Time-
-
Content LanguagesEnglish
-
Upload UserAnonymous/Not logged-in
-
File Pages16 Page
-
File Size-