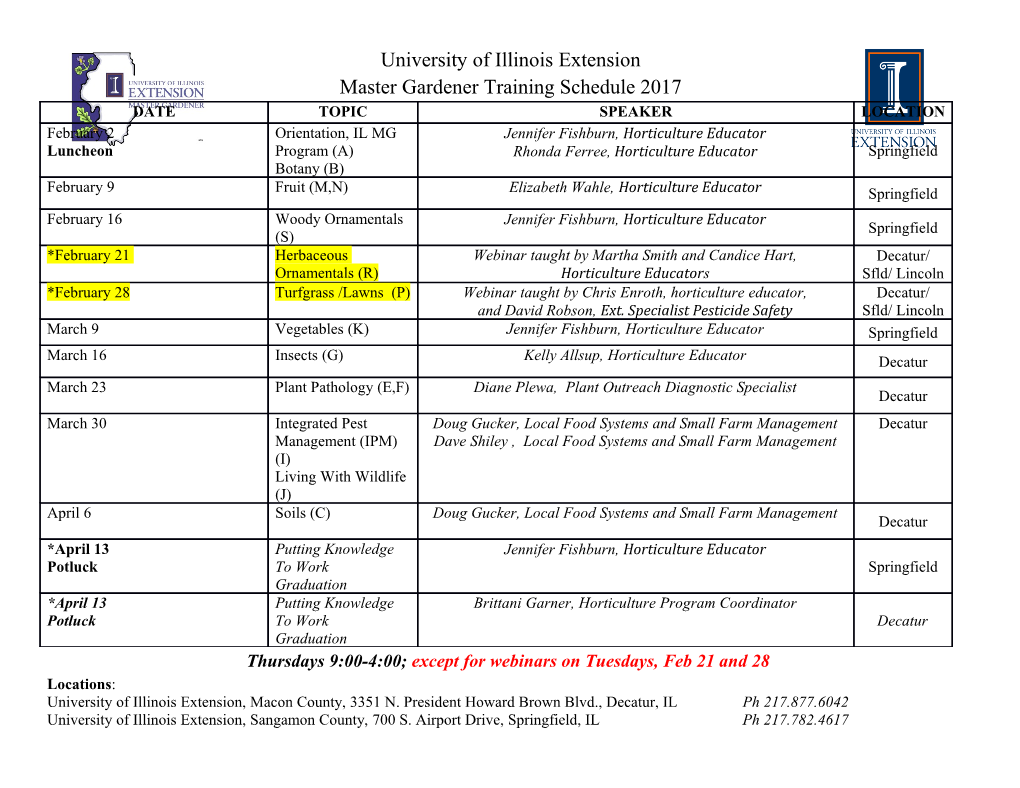
961 Learning47. Learning Classifier Cla Systems s Martin V. Butz 47.1 Background......................................... 962 Learning Classifier SystemsLCS ( s) essentially 47.1.1 Early Applications...................... 962 combine fast approximation techniques with evo- 47.1.2 The Pitt and Michigan Approach . 963 lutionary optimization techniques. Despite their 47.1.3 Basic Knowledge Representation 964 somewhat misleading name, LCSs are not only sys- tems suitable for classification problems, but may 47.2 XCS...................................................... 965 be rather viewed as a very general, distributed 47.2.1 System Overview ....................... 965 47.2.2 When and How XCS Works .......... 968 optimization technique. Essentially, LCSshavevery 47.2.3 When and How to Apply XCS....... 968 high potential to be applied in any problem do- 47.2.4 Parameter Tuning in XCS............. 969 main that is best solved or approximated by means of a distributed set of local approximations, or 47.3 XCSF .................................................... 970 predictions. The evolutionary component is de- 47.4 Data Mining ........................................ 972 signed to optimize a partitioning of the problem 47.5 Behavioral Learning............................. 973 domain for generating maximally useful predic- 47.5.1 Reward-Based Learning with LCSs 973 tions within each subspace of the partitioning. 47.5.2 Anticipatory Learning The predictions are generated and adapted by Classifier Systems ...................... 974 the approximation technique. Generally any form 47.5.3 Controlling a Robot Arm of spatial partitioning and prediction are pos- with an LCS ............................... 975 sible – such as a Gaussian-based partitioning combined with linear approximations, yielding 47.6 Conclusions ......................................... 977 a Gaussian mixture of linear predictions. In fact, 47.7 Books and Source Code ........................ 978 such a solution is developed and optimized by References................................................... 979 XCSF (XCS for function approximation). The LCSs XCS (X classifier system) and the function approxima- Part E | 47 tion version XCSF, indeed, are probably the most provides historical background on LCSs. Then XCS well-known LCS architectures to date. Their opti- and XCSF are introduced in detail providing enough mization technique is very-well balanced with the information to be able to implement, understand, approximation technique: as long as the approxi- and apply these systems. Further LCS architectures mation technique yields reasonably good solutions are surveyed and their potential for future research and evaluations of these solutions fast, the evolu- and for applications is discussed. The conclusions tionary component will pick-up on the evaluation provide an outlook on the many possible future signal and optimize the partitioning. This chapter LCS applications and developments. Learning classifier systems (LCSs) are machine learn- The main feature of LCSs is their innovative combina- ing algorithms that combine gradient-based approx- tion of two learning principles; whereas gradient-based imation with evolutionary optimization. Due to this approximation adapts local, predictive approximations flexibility, LCSs have been successfully applied to of target function values, evolutionary optimization classification and data mining problems, reinforcement structures individual classifiers to enable the formation learning (RL) problems, regression problems, cogni- of effectively distributed and accurate approximations. tive map learning, and even robot control problems. The two learning methods interact bidirectionally in 962 Part E Evolutionary Computation that the gradient-based approximations yield local fit- general are compared with other machine learning tech- ness quality estimates of the generated approximations, niques. which the evolutionary optimization technique uses This chapter starts with a historical perspective, for optimizing classifier structures. Concurrently, the providing information on the beginnings of LCSsand evolutionary optimization technique is generating new establishing some terminology background. We then in- classifier structures, which again need to be evaluated troduce the XCS classifier system providing a detailed by the gradient-based approach in competition with the system overview as well as theoretical and facet-wise other, locally overlapping, interacting classifiers. conceptual insights on its performance. Also tricks Due to the innovative combination of two learning and tweaks are discussed to tune the system to the and optimization techniques, LCSs are often perceived problem at hand. Next, the XCS counterpart for regres- as being hard to understand. Facet-wise analyses of sion problems, XCSF, is introduced. Focusing then on the individual LCS components and their interactions, the application-side, LCS applications to data mining however, give both mathematical scalability bounds tasks and to behavioral learning and cognitive modeling for learning and an intuitive understanding of the sys- tasks are surveyed. We cover various LCS architectures tems in general. Moreover, the currently most common that have been successfully applied in the data mining LCS, which is the XCS classifier system (note that realm. With respect to behavioral learning, we point out the X in XCS does not really encode any particular the relation of LCSs to reinforcement learning. More- acronym according to the system creator Wilson), is over, we cover anticipatory learning classifier systems comparatively easy to understand, to tune, and to ap- (ALCSs) – which learn predictive schema models of ply. Thus, the core of this chapter focuses on XCS, the environment rather than reward prediction maps – gives a facet-wise overview of its functionality, de- and we introduce the modified XCSF version that can tails several enhancements, and highlights various suc- effectively learn a redundant forward-inverse kinemat- cessful application domains. However, XCS is also ics model of a robot arm. A summary and conclusions compared with other LCS architectures and LCSsin wrap up the chapter. 47.1 Background Learning classifier systems (LCS) were proposed over rently experienced reward. Meanwhile, Holland’s cog- 30 years ago by Holland [47.1–3]. Originally, Hol- nitive system applied a genetic algorithm (GA) [47.1, land and Reitman actually called LCSs cognitive sys- 8] as its second learning mechanism. The GA modified Part E | 47.1 tems [47.4], focusing on problems related to reinforce- the taxa in Holland and Reitman’s cognitive system. ment learning (RL) [47.5, 6]. His cognitive system In sum, the first actual LCS implementation, i. e., developed a memory of classifiers, where each classi- the cognitive system by Holland and Reitman [47.4], fier consisted of a condition part (taxon), an action part was ahead of its time. It implemented various reward- (originally consisting of a message,andaneffector bit), related ideas that were later established in the reinforce- a payoff prediction part, and several other parameters ment learning community – and can now partially be that stored the age, the application frequency, and the regarded as standard RL techniques. However, the com- attenuation of the classifier. bination with GAs yielded a highly interactive and very Concurrently with the development of temporal dif- complex system that was and still is hard to analyze. ference learning techniques in RL – such as the now Thus, while proposing a highly innovative cognitive well-known state-action-reward-state-action (SARSA) learning approach, the applicability of the system re- algorithm [47.6]–Holland and Reitman introduced mained limited at the time. the bucket brigade algorithm [47.4, 7], which also dis- tributes reward backwards in time with a discounting 47.1.1 Early Applications mechanism. In addition, the attenuation parameter in a classifier realized something similar to an eligibility Nonetheless, early applications of LCSs were pub- trace in RL – distributing a currently encountered re- lished in the 1980s. Smith developed a poker deci- ward also to classifiers that were active several time sion making system [47.9] based on De Jong’s ap- steps ago and that thus indirectly led to gaining the cur- proach to LCSs [47.10]. Booker worked on animal-like Learning Classifier Systems 47.1 Background 963 automation based on the cognitive systems architec- the GA. As a consequence, the Pitt-style LCSsaremuch ture [47.11]. Wilson proposed and worked on the animat closer to general GAs because each individual consti- problem with LCS architectures derived from Hol- tutes an overall problem solution. In the Michigan-style land and Reitman’s cognitive systems approach [47.12, LCSs, on the other hand, each individual only applies 13]. Goldberg solved a gas pipeline control task with in a subspace of the overall problem and only the whole a simplified version of the cognitive system archi- set of rules that evolves constitutes the overall problem tecture [47.8, 14]. Despite these successful early ap- solution. Figure 47.1 illustrates this fundamental con- plications, a decade passed until a growing research trast between the two approaches. community developed that worked on learning classi- As a consequence of this contrast, Pitt-style LCSs fier systems. usually apply rather standard GA approaches. The whole population of rule sets is evolved. For fitness 47.1.2 The Pitt and Michigan Approach
Details
-
File Typepdf
-
Upload Time-
-
Content LanguagesEnglish
-
Upload UserAnonymous/Not logged-in
-
File Pages21 Page
-
File Size-