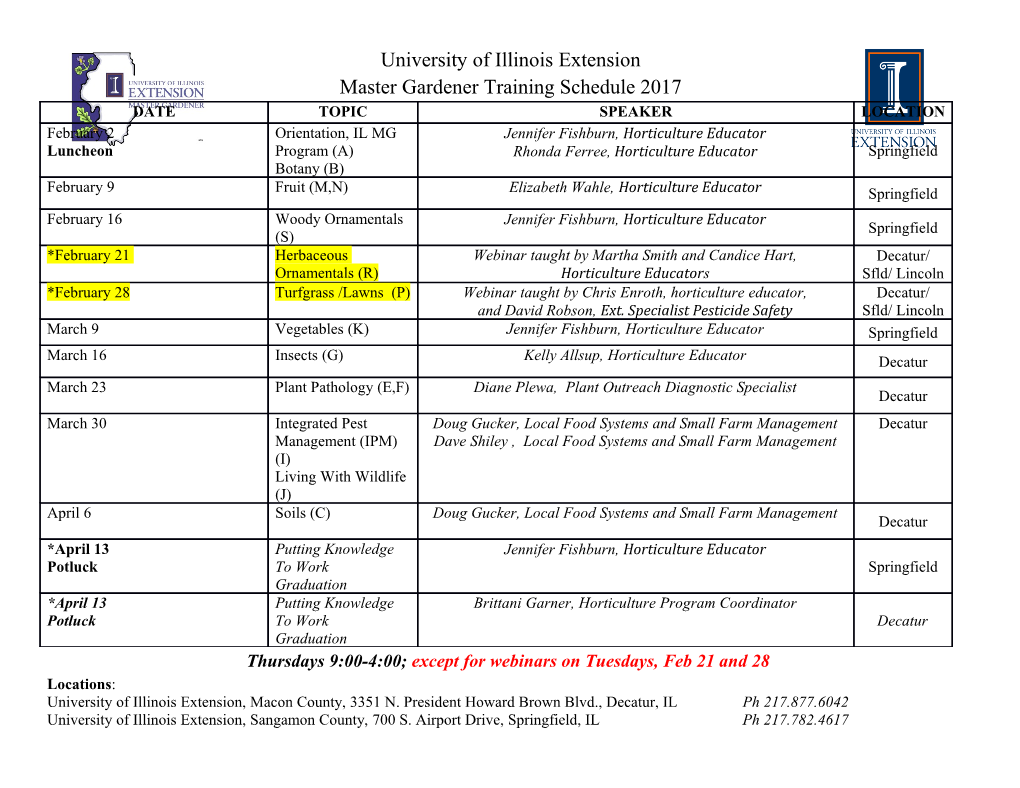
Biomedical Research and Therapy, 8(3):4277-4285 Open Access Full Text Article Original Research Advantages of functional analysis in comparison of different chemometric techniques for selecting obesity-related genes of adipose tissue from high-fat diet-fed mice Saravanan Dharmaraj*, Mahadeva Rao U. S. , Nordin Simbak ABSTRACT Introduction: Obesity is a lifestyle disease that is becoming prevalent nowadays and is associated with a surplus in energy balance related to lipid metabolism, inflammation and hypoxic condition, Use your smartphone to scan this resulting in maladaptive adipose tissue expansion. This study used the publicly available gene QR code and download this article dataset to identify a small subset of important genes for diagnostics or as potential targets for therapeutics. Methods: Chemometric analyses by principal component analysis (PCA), random forest (RF), and genetic algorithm (GA) were used to identify 50 genes that differentiate adipose samples from high-fat diet- and normal diet-fed mice. The first 30 important genes were studied for classifying the samples using six different classification techniques. Gene ontology (GO), pathway analysis, and protein-protein interaction studies on the 50 selected genes were subsequently done to identify important functional genes. Finally, gene regulatory effects by microRNA were assessed to confirm the genes' potential as targets for new therapeutic drugs. Results: The genes identified by RF are best for differentiating the samples, followed by PCA, with the least predictability shown by genes chosen by GA. However, PCA identified more genes with functional importance, such as the hub genes ATP5a1 and Apoa1. ATP5a1 is the main hub gene, whereas Apoa1 is involved in cholesterol metabolism. Vapa and Npc2 are crosstalk genes that link both of these main genes and could be targeted for therapeutic drug design. Conclusion: The combination of different chemometric techniques and functional analysis of genes could be used to select for a small number of genes which could serve as more suitable diagnostic or therapeutic targets. Key words: gene ontology, obesity, principal component analysis, protein-protein interaction, random forest Faculty of Medicine, Universiti Sultan Zainal Abidin, Medical Campus, 20400 Kuala Terengganu, Terengganu, Malaysia. INTRODUCTION sity, with the monogenic type being further classified Obesity is defined as an accumulation of white adi- as syndromic or non-syndromic. People with mono- Correspondence pose tissue, with the disease often occurring together genic obesity represent only a small percentage of the Saravanan Dharmaraj, Faculty of obese population, whereas common obesity with no Medicine, Universiti Sultan Zainal Abidin, with hyperglycemia, hypercholesterolemia and hy- Medical Campus, 20400 Kuala pertension; this cluster is often termed metabolic syn- obvious Mendelian inheritance pattern is polygenetic 5 Terengganu, Terengganu, Malaysia. drome 1. Data analysis between 1980 and 2015 from and highly prevalent . It has been mentioned that Email: 68.5 million persons showed an increasing prevalence for any disease, one of the greatest challenges lies not [email protected] of obesity and overweight condition in children and in the identification of association genes but in as- History adults. In 2015, approximately 108 million children certaining the molecular mechanisms by which those • Received: Jan 09, 2021 and 604 million adults were designated as obese 2. factors/genes reduce the disease risk or phenotypic • Accepted: Mar 26, 2021 6 Adipose tissue plays a key role in systemic en- expression . • Published: Mar 31, 2021 ergy homeostasis; indeed, any dysfunction involving The explosion of genomic data in terms of expres- DOI : 10.15419/bmrat.v8i3.666 adipocytes, such as hypertrophy, fibrosis, hypoxia and sion levels of thousands of genes from microarray robust inflammation, is known to contribute to obe- studies, combined with chemometric and bioinfor- sity 3. The wide imbalance between energy intake and matic tools, has enabled the identification of candi- expenditure in obesity results from a combination of date biomarker genes and pathways. The aim of the Copyright genetic, epigenetic, physiological, behavioral, socio- study was to use chemometric analyses of principal © Biomedpress. This is an open- cultural and environmental factors which make the component analysis (PCA), random forest (RF), and access article distributed under the diagnosis and management of obesity difficult 4. Obe- genetic algorithm (GA) to identify a small fraction of terms of the Creative Commons Attribution 4.0 International license. sity can be divided into monogenic or polygenic obe- genes that differentiate high-fat diet- and normal diet- Cite this article : Dharmaraj S, M R U S, Simbak N. Advantages of functional analysis in comparison of different chemometric techniques for selecting obesity-related genes of adipose tissue from high- fat diet-fed mice. Biomed. Res. Ther.; 8(3):4277-4285. 4277 Biomedical Research and Therapy, 8(3):4277-4285 fed adipose samples from mice using the microar- and related apps which were downloaded from the ray dataset GSE39549. Various classification tech- Cytoscape website (https://cytoscape.org/). The anal- niques were used to check which set of genes are best yses were all carried out on an Intel® CoreTM i5-7400 for classification purposes, whereas the underlying CPU@ 3.0 GHz with 16.0 GB RAM. mechanisms were studied using functional gene an- notation, pathway analysis, protein-protein interac- Gene selection algorithms tion, and miRNA regulation. The PCA was carried out using the prcomp function MATERIALS - METHODS in the R program. The RF method has only a cou- ple parameters which need to be chosen (mtry and Overview of Methods ntree). The mtry was set to 120 and ntree was set The methods’ workflow consisted of dataset selection to 1000. The GA was carried out with Matlab using and pre-processing, selection of genes by three mul- the approach described previously 10,11. The parame- tivariate techniques, and evaluation of the classifica- ters chosen were the number of chromosomes of 100, tion accuracy of the selected genes. In addition, eval- ndims of 3, and the algorithm was run for 400 gen- uations of the biomechanism of the genes and their erations. The number of genes selected from each potential clinical significance, functional annotation, chemometric method was 50. protein-protein interaction, and miRNA-target gene interactions were conducted. Use of machine learning for classification The gene selection method had chosen 50 genes from Data retrieval and pre-processing either PCA, RF or GA, and the ability of the first 30 The Gene Expression Omnibus (GEO; https://www. genes from each were selected for differentiating be- ncbi.nlm.nih.gov/gds), a public functional genomics tween the high-fat diet and control diet. The cor- data repository, was searched for ‘obesity’ and the rect classifications were predicted using six differ- choice of the dataset was based on an adequate num- ent supervised chemometric techniques, which con- ber of samples. The chosen dataset of GSE 39549 was sisted of k-nearest neighbors (kNN), logistic regres- downloaded from the Gene Expression Omnibus to sion, linear discriminant analysis, Naïve-Bayes, and gain insight into the relationship between obesity and two types of singular vector machines (SVM) 12,13. hypoxia. This dataset consisted of both adipose and The first SVM evaluator used was a non-kernel or liver samples from mice fed with a high-fat diet and linear-based method, whereas the second SVM used the corresponding control diet 7. The data used in this was the sigmoid-based kernel. The other parameters study consisted of gene expression data from the adi- chosen for the above techniques were k = 5 for kNN, pose samples. Microsoft Access was used to map the as well as use of the binomial option for logistic re- probe sets of the genes (which were differentially ex- gression. pressed by more than 2.0-fold) to Entrez Gene IDs, 8,9 and the average expression values of 15.000 genes Functional enrichment and pathway analy- were obtained. The original data consisted of differ- sis (Functional annotation clustering) ent time points but in this study the data were pooled to compare the high-fat diet and control/normal diet. Functional enrichment analysis was carried on the This helped overcome the dimensionality problem as- genes chosen by the three methods by loading the se- sociated with microarray data where variables are very lected genes into the Functional Annotation tool in large but the number of samples is limited. the Database for Annotation Visualization and Inte- grated Discovery (DAVID; https://david.ncifcrf.gov/ Software and packages ) to identify Gene Ontology (GO) functions, espe- Three approaches were used to carry out the selection cially those pertinent to biological processes, molec- of genes. In the first approach, the free R package ular functions and cellular components. A total of 50 with prcomp as well as randomForest libraries were chosen genes by each method was evaluated for func- used for selecting genes by PCA and RF; conversely, tional annotation, and the similarity term overlap was GA was undertaken using Matlab R2019b. The se- set to 3. The similarity threshold was 0.50, whereas p- lected variables or genes’ ability to classify the sam- value < 0.05 was used to obtain the optimal and sta- ples was further carried by the use of glm and e1071
Details
-
File Typepdf
-
Upload Time-
-
Content LanguagesEnglish
-
Upload UserAnonymous/Not logged-in
-
File Pages9 Page
-
File Size-