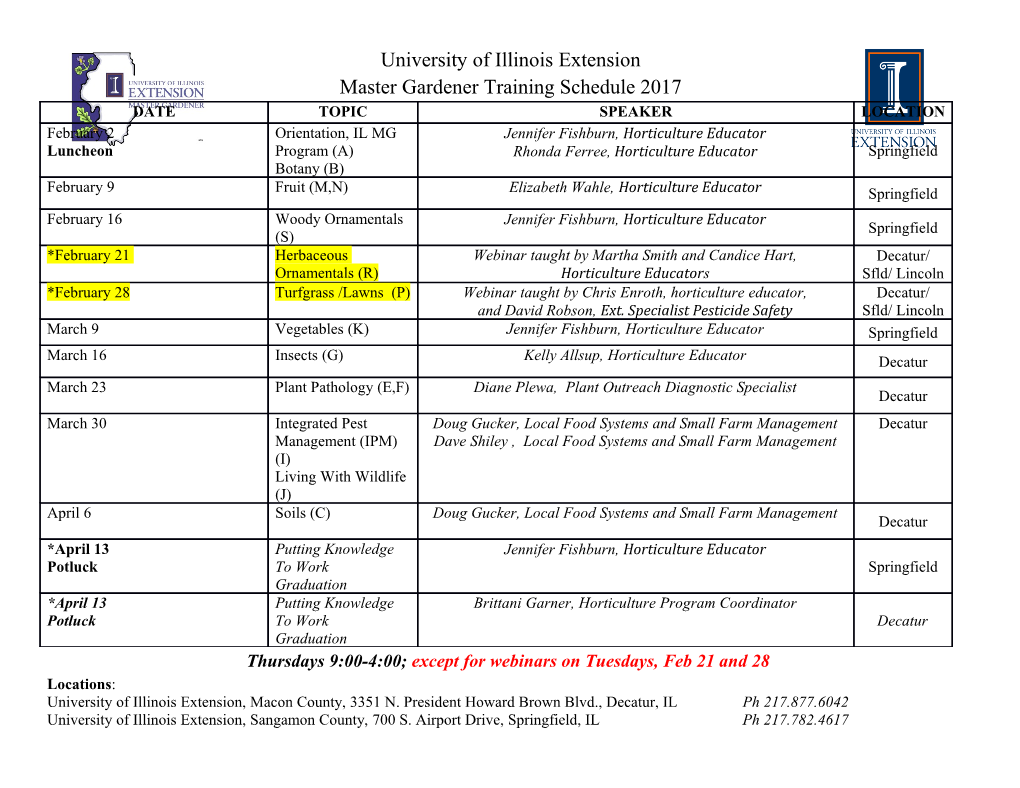
Automated Feature Learning for Intrusion Detection in Unstructured Log Data Master Thesis submitted for completion of the MSc Cognitive Science and Artificial Intelligence at Tilburg University by Martijn van Laar under supervision of Martijn van Otterlo (Tilburg University) Abstract: Log-based anomaly detection for cybersecurity has focused on different types of log files. Natural language-based log files, particularly the more dynamic log types, have received little attention in previous research. In the current thesis, features are automatically learned from HTTP log data from a live server, using various classifiers. An Host-based Intrusion Detection System (HIDS) provides input for this task. An LSTM-based classifier with a final AUROC of 0.98 shows features in dynamic unstructured log data can be learned from examples. 1. Motivation According to a recent survey, more than half of all internet traffic is generated automatically (Zeifman 2017). These automated agents or bots can be defined as systems being part of an online environment, acting within that environment in pursuit of their own agenda (Franklin and Graesser 1997). Bots in that respect can perform different actions, depending on their built-in agenda. Some benign bots index the internet for search engines, while others identify and reverse so-called Wikipedia vandalism. On the other hand, malicious bots search the internet for email addresses to send spam to, or perform DDoS attacks (Tsvetkova et al. 2017). Of all internet traffic in 2016, as much as 28.9% originated from different kinds of malicious bots. A large portion of this traffic can be attributed to DDoS-attacks and other traffic without immediate security risks for end users. However, as much as 2.6% of all traffic can be traced back to hackers looking for sites with vulnerabilities they might exploit. 94.2% of websites part of a survey auditing 100,000 websites experienced at least one bot attack within a period of 90 days (Zeifman 2017). With the internet by now an essential part of everyday life, security on the internet is of greater importance than ever before. Most attacks are preventable, for example by having up to date software installed, having secure passwords and monitoring known vulnerabilities. However, not all websites and servers are maintained as well as would be desirable. Bots oriented towards intrusion on websites or servers can have differing agendas. Some try and infiltrate contact forms in order to send spam using a website’s email functionality, while others work towards database intrusion to find badly encrypted user data. Results from these efforts can include selling user accounts and passwords or using badly secured servers as nodes for the TOR network, aimed at masking identities and used for accessing the dark web. A widely-used strategy for locating insecure websites entails requesting possible paths on a website for applications or plugins that are known to have vulnerabilities. Once such an application is located, these vulnerabilities are employed, with varying consequences depending on the application in question. This scanning tactic can be used to identify bots in website access logs. Identifying these bots can require large amounts of domain knowledge, which is not always available. This thesis will focus on modelling an Intrusion Detection System (IDS), automatically detecting features using deep neural networks in access log data gathered from a live environment. Since the amount of available data is vast and labelled data is lacking, defined intrusions by an often used implementation of these IDSs, OSSEC (Hay, Cid, and Bray 2008), will provide labelled input for this task. The main goal in this thesis is not to replace the already fully functional IDS, but to indicate how tools originating in natural language processing (NLP) can enhance and support future text-based log file anomaly detection, ultimately showing features of these types of logs can be machine-learnable. - 2 - 2. Introduction In the following section, an overview will be provided of what Intrusion Detection Systems exactly entail and how the field of anomaly detection offers insights in improving these systems. Furthermore, data used in these studies and current problems with regard to data collection and preparation will be discussed. These issues will lead to defining the central research question in this thesis, which is: “To what extent can rule-based anomaly detection in text-based log files be approximated by a statistical model, using self-learned features?” 2.1 Intrusion Detection Systems Because of the large amount and variety of internet traffic, keeping track of possible security issues by hand is not feasible for any system administrator. On top of implementing the appropriate security measures, automatically detecting any possible remaining intrusions is of a high priority for any network. For these kinds of situations, Intrusion Detection Systems (IDSs) are set up. Two main branches of IDSs can be identified: Network IDS (NIDS) and Host-based IDS (HIDS). NIDSs detect intrusion from a centralised location in a defined network. Since the current thesis focuses on web and server site security, centralised intrusion detection is more difficult because of secure Hypertext Transfer Protocol (HTTP) traffic, or HTTPS. HTTPS traffic is encrypted, and is in most applications only decrypted and thus readable for an IDS on the host server. Consequently, intrusion detection for websites and webservers is usually performed at the host server. Within the realm of HIDSs, the two main branches are signature-based and anomaly- based intrusion detection. Signature-based HIDS works by tracking known attack signatures in the analysed traffic. While signature-based IDS can be reliable in detecting known attacks with low false positive rates (Patcha and Park 2007), this signature has to be provided by some expert (Ahmed, Naser Mahmood, and Hu 2016), and can thus be cumbersome and less sufficient in case of new, unique or yet undocumented attacks (Wang and Jones 2017). Considering signature-based HIDS’s limitations, the more robust and suitable solution for the general purpose of defending against constantly evolving online attacks is anomaly-based HIDS. This type of IDS refers to the way this behaviour manifests itself. Once behaviour is sufficiently divergent from what would be expected behaviour from a regular user, it can be classified as anomalous. With this technique, malicious behaviour can be detected without the need for knowledge about this specific behaviour beforehand, like with fingerprinting. However, because of the higher false positive rates (Patcha and Park 2007), behaviour marked as divergent can only be viewed as evidence for this traffic being malicious. Anomalous behaviour cannot be regarded as conclusive proof of an attack (Ahmed, Naser Mahmood, and Hu 2016; Zuech, - 3 - Khoshgoftaar, and Wald 2015). 2.2 Anomaly detection Anomalies can be defined as patterns in data that are not in line with expected or normal behaviour (Chandola, Banerjee, and Kumar 2009). The detection of anomalies has been widely studied, both in general as well as specifically applied to intrusion detection. In the general sense, anomaly detection techniques can be related to anything from engine health monitoring (Hayton et al. 2007) to crowd control (Mahadevan et al. 2010). To understand the focus of previous work on different types of anomalies, it is vital to distinguish these prior to discussing any studies. Chandola et al. (2009) describe point anomalies, contextual anomalies and collective anomalies. Point anomalies can be defined as a single data point that differs with respect to the rest of the data, the most basic type of anomaly. Contextual anomalies are those data points that could be considered normal in a certain context, but anomalous when that context differs. For instance, consider a normal distribution S where µ is its mean at Xµ and a standard deviation σ at Xµ + Xσ. A data point with the value of µ should be considered normal around Xµ, but anomalous at Xµ + Xσ. Finally, collective anomalies refer to those sets of observations that would individually be considered non-anomalous, but are determined to be anomalous because of their order, duration or quantity (Chandola, Banerjee, and Kumar 2009). Some issues arise throughout cybersecurity-oriented anomaly detection studies. Ahmed et al. define some of these issues (Ahmed, Naser Mahmood, and Hu 2016). First of all, defining normal behaviour proves challenging. For example, once a user requests a webpage that does not exist and receives a 404 status code, this could be considered normal use for a human typing error. However, when such a request is made by a malicious bot performing a scan, this could be classified as anomalous because of the source, not because of the content. These requests might be equal content-wise, but only their intentions provide the information on which a classification could be reliably made. However, this information is rarely available or should be learned from context, making these kinds of request a type of collective anomaly, unseparable as point anomalies without external information. Additionally, even when a normal state has been defined to distinguish anomalies from, this might be subject to rapid change at all times. The dynamic nature of server implementations could result in activity originating from a newly installed application to be considered anomalous at first, but prove unharmful or even benificial after further inspection. These anomalies can only be eliminated after further context has been produced. Furthermore, a lack of labelled and reliable datasets results in studies not having as much of an impact as they claim. - 4 - 2.3 Data used in cybersecurity-oriented anomaly detection Defining distinctions between log data types commonly researched in log data-based anomaly detection studies will help zeroing in on methods particularly fit for the current problem. See figure 1 for a flowchart of different log data types and their methods of pre- processing for use in classification problems.
Details
-
File Typepdf
-
Upload Time-
-
Content LanguagesEnglish
-
Upload UserAnonymous/Not logged-in
-
File Pages49 Page
-
File Size-