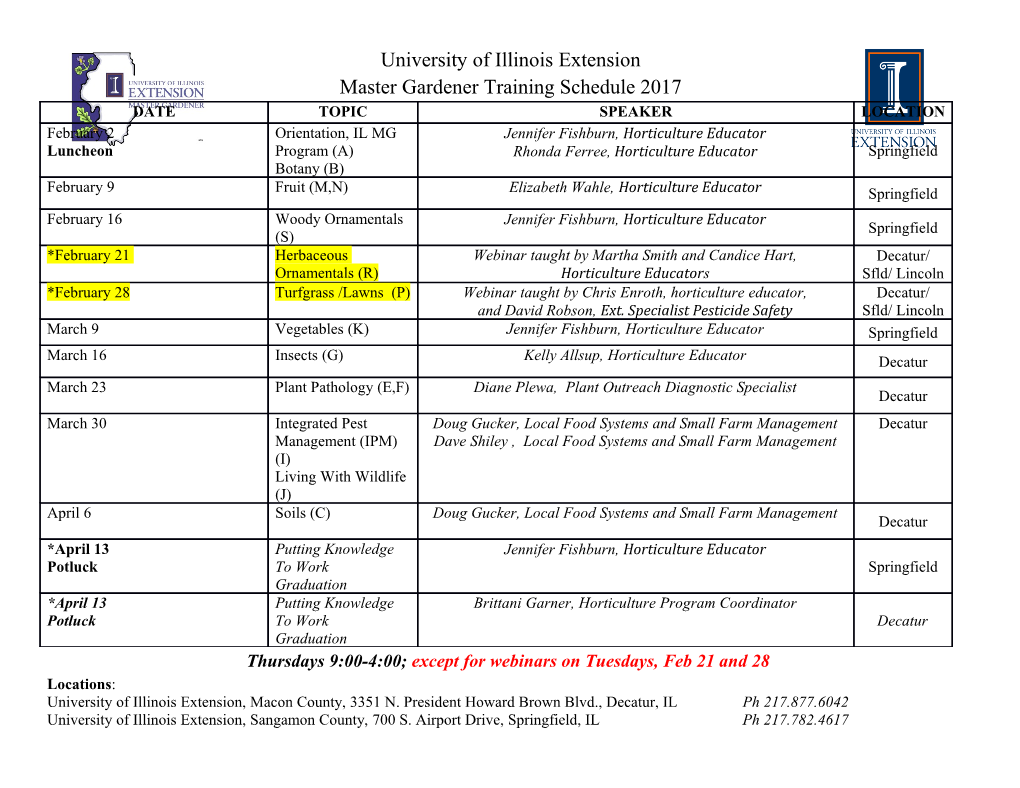
Tolerance Intervals K. Krishnamoorthy University of Louisiana at Lafayette, Lafayette, LA, USA Dakar International Conference on Recent Developments in Applied Statistics March 17, 2014 1 / 60 A motivating example Air lead levels collected by the National Institute of Occupational Safety and Health (NIOSH) at a laboratory, for health hazard evaluation. The air lead levels were collected from 15 different areas within the facility. Air lead levels (µg/m3) 200 120 15 7 8 6 48 61 380 80 29 1000 350 1400 110 A normal distribution fitted the log-transformed lead levels quite well (that is, the sample is from a lognormal distribution). Objective: Are 90% of air lead levels in the facility below the occupational exposure limit (OEL) 50 µg/m3 ? 2 / 60 A motivating example Y : Air lead levels, X : log-transformed air lead levels. µ and σ2: population mean and variance for X . X N(µ,σ2). ∼ exp(µ): median air lead level. The usual confidence interval for µ: X¯ and S, the sample mean and standard deviation of the log-transformed data for a sample of size n. S A 95% confidence interval for µ: X¯ tn 1;.975 ± − √n S A 95% upper confidence bound for µ: X¯ + tn 1;.95 . − √n Confidence intervals for the median air lead level can be obtained. 3 / 60 A motivating example To predict the air lead level at a particular area within the laboratory, a 95% prediction interval 1 X¯ tn 1;.975S 1+ ± − r n for the log-transformed lead level can be used. However, the confidence interval and prediction interval cannot answer this question, “Are 90% of the population lead levels below a threshold?” What is required is a tolerance interval; more specifically, an upper tolerance limit. 4 / 60 One-Sided Tolerance Limits Let X = (X1, ..., Xn) be a sample from a population. A (p, 1 α) upper tolerance limit U(X) is constructed so − that at least 100p percent of the population is U(X ) with ≤ confidence 1 α. − A (p, 1 α) lower tolerance limit L(X ) is constructed so − that at least 100p percent of the population is L(X ) with ≥ confidence 1 α. − A (p, 1 α) tolerance interval (L(X ), U(X )) is constructed − so that the interval would include at least 100p percent of the population with confidence 1 α. − 5 / 60 One-Sided Tolerance Limits A (p, 1 α) upper tolerance limit is a 100(1 α)% upper − − confidence limit for the 100pth percentile of the population of interest. For example, let Q.90 denote the 90th percentile of a population, and let U(X ) be a 95% upper confidence limit for Q.90. Note that 90% of the population Q U(X ) with confidence 95%. ≤ .90 ≤ So at least 90% of the population is less than or equal to U(X ) with confidence 95%. Similarly, we can argue that the (p, 1 α) lower tolerance limit − is a 100(1 α)% lower confidence limit for the 100(1 p)th − − percentile of the population of interest. 6 / 60 Two-Sided Tolerance Intervals Construction of one-sided tolerance limits simplifies to finding one-sided confidence limits for appropriate percentiles of the population. Thus, the problem simplifies to interval estimation of some parametric function. A (p, 1 α) two-sided tolerance interval (L(X ), U(X )) contains at − least a proportion p of the population with confidence 1 α. i.e., − PX % of population in (L(X ), U(X )) p = 1 α { ≥ } − PX PX L(X ) X U(X ) X p = 1 α. ≤ ≤ ≥ − We here notice that the computation of L(X ) and U(X ) does not reduce to the computation of confidence limits for certain quantiles. 7 / 60 Normal Distribution: One-Sided Tolerance Limits 2 Let X1, ..., Xn be a sample from a N(µ,σ ) population with unknown mean µ and unknown variance σ2. The sample mean X¯ and sample variance S2 are defined by n n 1 2 1 2 X¯ = Xi and S = (Xi X¯) . n n 1 − i i X=1 − X=1 We shall describe the computation of one-sided tolerance limits based on X¯ and S2 for a normal population. zp: p quantile of a standard normal distribution. The 100pth percentile of N(µ,σ2) is qp = µ + zpσ, where zp is 100pth percentile of the std norma; distribution. A 1 α upper confidence limit for qp is a (p, 1 α) one-sided upper − − tolerance limit for the normal population. 8 / 60 Normal Distribution: One-Sided Tolerance Limits (.90, .95) upper tolerance limit is a 95% upper confidence limit for µ + z.90σ. 9 / 60 Normal Distribution: One-Sided Tolerance Limits (.90, .95) lower tolerance limit is a 95% lower confidence limit for µ − z.90σ. 10 / 60 Normal Distribution: One-Sided Tolerance Limits The (p, 1 α) upper tolerance limit is taken to be of the form − X¯ + k1S, and k1 is referred to as the tolerance factor and is to be determined so that P(X¯ + k S µ + zpσ) = 1 α. 1 ≥ − It can be shown that the pivotal quantity µ + zpσ X¯ 1 − tn 1(zp√n), S ∼ √n − where tm(δ) is the noncentral t distribution with df = m and the noncentrality parameter δ. Therefore, µ + zpσ X¯ 1 − tn 1;1 α(zp√n) with probability 1 α. S ≤ √n − − − 11 / 60 Normal Distribution: One-Sided Tolerance Limits Rearranging the terms, we find µ + zpσ X¯ + k S with probability 1 α, ≤ 1 − where 1 k1 = tn 1;1 α(zp√n). √n − − Similarly, it can shown that X¯ k S − 1 is a (p, 1 α) lower tolerance limit for the N(µ,σ2) distribution. − 12 / 60 Normal Distribution: Two-Sided Tolerance Intervals (.90, .95) lower tolerance limit is a 95% lower confidence limit for µ − z.90σ. 13 / 60 Normal Distribution: Equal-tailed TI 14 / 60 Normal Distribution: Equal-Tailed TI A (p, 1 α) equal-tailed tolerance interval (L, U) is constructed so − that it will include the interval µ z 1+p σ, µ + z 1+p σ − 2 2 with probability 1 α. Note that the interval is determined so that − 1 p no more than a proportion −2 of the population is < L and 1 p no more than a proportion −2 of the population is > L In may applications, such restriction is not needed, and so we shall consider only two-sided TIs in the sequel. 15 / 60 Normal Distribution: Two-Sided Tolerance Intervals A two-sided tolerance interval: X¯ k S. ± 2 k2 is determined such that the interval would contain at least a proportion p of the normal population with confidence 1 α. − P ¯ PX (X¯ k S X X¯ + k S X¯, S) p = 1 α, X ,S − 2 ≤ ≤ 2 | ≥ − X N(µ,σ 2), independently of X¯ and S. ∼ The computation of tolerance factor k2 is numerically involved. Software packages such as StatCalc can be used. We can approximate k2 as 1 mχ2 (1/n) 2 k 1;p 2 2 , (1) ≃ χm;α ! where m = n 1, and χ2 (δ) denotes the α quantile of a − m;α noncentral chisquare distribution with df m and noncentrality parameter δ. 16 / 60 Normal Distribution: Example 1 (Air Lead Level) In this example, we like to assess the air lead level in a laboratory. The data in Table 2.1 represent air lead levels collected by the National Institute of Occupational Safety and Health (NIOSH) at a laboratory, for health hazard evaluation. The air lead levels were collected from 15 different areas within the facility. Table 2.1 Air lead levels (µg/m3) 200 120 15 7 8 6 48 61 380 80 29 1000 350 1400 110 Log-transformed lead levels fit a normal distribution (that is, the sample is from a lognormal distribution). 17 / 60 Normal Distribution: Example 1 18 / 60 Normal Distribution: Example 1 We compute an upper tolerance limit based on the log-transformed data in order to assess the maximum air lead level in the laboratory. The sample mean and standard deviation of the log-transformed data:x ¯ = 4.333 and s = 1.739. To compute a (0.90, 0.95) upper tolerance limit for the air lead level, the tolerance factor 2.068, and x¯ + k1s = 4.333 + 2.068(1.739) = 7.929. Thus, exp(7.929) = 2777 is a (0.90, 0.95) upper tolerance limit for the air lead levels. 19 / 60 Normal Distribution: Example 1 The occupational exposure limit (OEL) for lead exposure set by the Occupational Safety and Health Administration (OSHA) is 50 µg/m3. A work place is considered safe if an upper tolerance limit does not exceed the OEL. In this case, the upper limit of 2777 far exceeds the OEL; hence we can not conclude that the workplace is safe. 20 / 60 Normal Distribution: Assessing Survival Probability In many applications it is desired to estimate the probability that a random variable exceeds a specified value. For example, in lifetime data analysis, it is of interest to assess the probability that the lifetime of an item exceeds a value (survival probability). In industrial hygiene, it is of interest to estimate the probability that the exposure level (level of exposure to a contaminant in a workplace) of a worker exceeds the occupational exposure limit (OEL). This is referred to as the exceedance probabilitiy. To assess the lifetime of an item, a lower confidence limit for the survival probability is warranted, and to assess the exposure level in a workplace, one needs an upper confidence limit for the exceedance probability.
Details
-
File Typepdf
-
Upload Time-
-
Content LanguagesEnglish
-
Upload UserAnonymous/Not logged-in
-
File Pages60 Page
-
File Size-