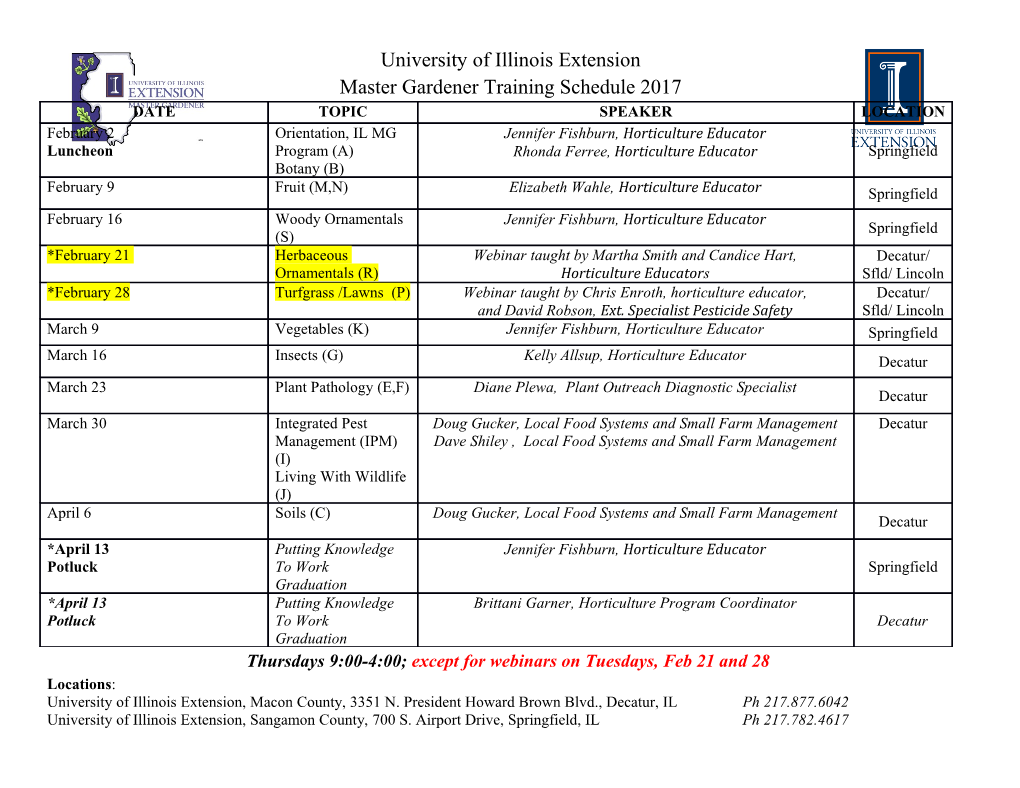
50 Years of Data Analysis from EDA to predictive modelling and machine learning Gilbert Saporta CEDRIC- CNAM, 292 rue Saint Martin, F-75003 Paris [email protected] http://cedric.cnam.fr/~saporta Data analysis vs mathematical statistics • An international movement in reaction to the abuses of formalization • Let the data speak • Computerized statistics ASMDA 2017 2 • « He (Tukey) seems to identify statistics with the grotesque phenomenon generally known as mathematical statistics and find it necessary to replace statistics by data analysis » (Anscombe, 1967). John Wilder Tukey (1915-2000) ASMDA 2017 3 • « Statistics is not probability, under the name of mathematical statistics was built a pompous discipline based on theoretical assumptions that are rarely met in practice » (Benzécri, 1972) • « Data analysis is a tool to release from the gangue of the data the pure diamond of the true nature » Jean-Paul Benzécri (1932- ) ASMDA 2017 4 Japan, Netherlands, Canada, Italy… Chikio Hayashi (1918-2002) Jan de Leeuw (1945-) Shizuiko Nishisato (1935-) Carlo Lauro (1943-) ASMDA 2017 5 Meetings • Data Analysis and Informatics • ASMDA from 1981 from 1977 Edwin Diday Ludovic Lebart Jacques Janssen ASMDA 2017 6 Part 1 Exploratory data analysis « Data Analysis »: a collection of unsupervised methods for dimension reduction • « Factor » analysis • PCA, • CA, MCA • Cluster analysis • k-means partitioning • Hierarchical clustering ASMDA 2017 8 and came the time of syntheses: • All (factorial) methods are particular cases of: PCA canonical correlation analysis 1976 J.Douglas Carroll (1939 -2011) ASMDA 2017 9 Even more methods are particular cases of the maximum association principle: p MaxYj(,) Y X j1 M.Tenenhaus (1977), J.F. Marcotorchino (1986), G.S. (1988) ASMDA 2017 10 • A few cases criterium analysis p 2 PCA max r ( c , xjj ) with x numerical j1 p 2 xx MCA max (c ,jj ) with categorical j1 p GCA (Carroll) 2 max Rc ( ,XXjj ) with data set j1 p Central partition xx max Rand ( Y ,jj ) with Y and categorical j1 p Condorcet aggregation rule max (yx ,j ) with rank orders j1 ASMDA 2017 11 …and the time of clusterwise methods: • Looking simultaneously for a partition and k local models instead of a global model (PCA, regression etc.) Diday, 1974 Charles, 1977 Späth, 1979 DeSarbo & Cron, 1988 Preda & S., 2005 Bougeard, Niang, & S. 2017 Adapted from Hennig, 2000 ASMDA 2017 12 followed by the time of extensions to new types of data • PCA and MCA of functional data X in xt(n) xt(2) i2 xt(1) i1 0 t T in state 1 state 2 i state 3 2 state 4 i1 Jean-Claude Deville Jim Ramsay 0 T ASMDA 2017 13 • Symbolic data • Textual Data • Intervals, histograms, distributions etc. (Bock, Diday, Billard , …) ASMDA 2017 14 Towards non-linear data analysis • Semi linear PCA (Dauxois & Pousse 1976, Gifi 1990) p p j arg maxV a x j arg maxVx j instead of j j1 j1 • Kernel PCA (Schölkopf, B., Smola, A., Müller, K.L., 1998) • Metric (Torgerson) MDS in the feature space where the dot product k(,)xy is a simple function of xy, ASMDA 2017 15 The time of sparse methods • Inspired by the Lasso. • Useful for high dimensional data: • Lack of interpretability • Unstable results • Sparse PCA βˆ arg min z - Xβ22 + β + β 1 1 Zou et al., 2006 • Alternates SVD (z) and elastic-net (β) • Sparse multiple correspondence analysis (Bernard, Guinot & S., 2012) ASMDA 2017 16 Application on genetic data Single Nucleotide Polymorphisms Data: n=502 individuals p=537 SNPs (among more than 800 000 of the original data base, 15000 genes) q=1554 (total number of columns) X : 502 x 537matrix of qualitative variables K : 502 x 1554 complete disjunctive table K=(K1, …, K1554) 1 block = 1 SNP = 1 Kj matrix ASMDA 2017 17 Application on genetic data Comparison of the loadings . ASMDA 2017 18 Part 2 Predictive modelling Machine Learning • A continuation of Data Analysis • “the models should follow the data, not vice versa” JPB principle n°2 * • “use the computer implies the abandonment of all the techniques designed before of computing” JPB principle n°5 * • Data driven methods vs hypothesis driven methods • No (or few) prespecified distribution assumptions * Translated by C.Lauro: https://www.researchgate.net/post/The_origin_of_Data_Science_the_5_principles_of_Data_Analysis_Analyse _des_donnees_by_JP_Benzecri ASMDA 2017 20 The two cultures 1928-2005 ASMDA 2017 21 • The generative modelling culture • seeks to develop stochastic models which fits the data, and then make inferences about the data-generating mechanism based on the structure of those models. Implicit (…) is the notion that there is a true model generating the data, and often a truly “best” way to analyze the data. • The predictive modelling culture • is silent about the underlying mechanism generating the data, and allows for many different predictive algorithms, preferring to discuss only accuracy of prediction made by different algorithm on various datasets. Machine Learning is identified by Breiman as the epicenter of the Predictive Modeling culture. From Donoho, 2015 ASMDA 2017 22 • Standard conception (models for understanding) • Provide some comprehension of data and their generative mechanism through a parsimonious representation. • A model should be simple and its parameters interpretable for the specialist : elasticity, odds-ratio, etc. • In « Big Data Analytics » one focus on prediction • For new observations: generalization • Models are merely algorithms Cf GS, compstat 2008 ASMDA 2017 23 Same formula: y= f(x;)+ • Generative modelling • Predictive modelling • Underlying theory • Models come from data • Narrow set of models • Algorithmic models • Focus on parameter estimation and • Focus on control of generalization goodness of fit: predict the past error : predict the future • Error: white noise • Error: minimal ASMDA 2017 24 Paradigms and paradoxes • Understanding but poorly predict • a model with a good fit may provide poor predictions at an individual level (eg epidemiology) • Predict without understanding? • Good predictions may be obtained with uninterpretable models (targetting customers, or approving loans, do not need a consumer theory) • Simplicity • « Occam’s Razor, long admired, is usually interpreted to mean that simpler is better. Unfortunately in prediction, accuracy and simplicity (interpretability) are in conflict » Breiman, 2011 ASMDA 2017 25 The black box model Vladimir Vapnik (1936-) • Let y=f(x)+ be an unknown generative model . One looks for a good approximation of the black box rule. • Two very different concepts : • Be close to the true f • Provide good enough predictions (mimic the black box behaviour). ASMDA 2017 26 • Modern statistical thinking makes a clear distinction between the statistical model and the world. The actual mechanisms underlying the data are considered unknown. The statistical models do not need to reproduce these mechanisms to emulate the observable data (Breiman, 2001). • Better models are sometimes obtained by deliberately avoiding to reproduce the true mechanisms (Vapnik, 2006). • Statistical significance plays a minor or no role in assessing predictive performance. In fact, it is sometimes the case that removing inputs with small coefficients, even if they are statistically significant, results in improved prediction accuracy (Shmueli, 2010) ASMDA 2017 27 Too big ? • Estimation and tests become useless • Everything is significant! • with n=106 a correlation coefficient = 0,002 is significantly different from 0 but without any interest • Usual distributional models are rejected since small discrepancies between model and data are significant • Confidence intervals have zero length • George Box: « All models are wrong, some are useful » ASMDA 2017 28 Meta or ensemble models • Bagging, boosting, random forests etc. dramatically improve simple models • Stacking (Wolpert, Breiman) linearly combines predictions coming from various models (linear, trees, knn, neural networks, etc.) ˆ ˆ ˆ f12(x ), f ( x ),..., fm ( x ) • First idea: OLS 2 nm ˆ minyi w j f j (x ) ij11 • Favorize most complex models, risk of overfitting ASMDA 2017 29 • Better solution: use leave one out predicted values 2 nm ˆ i minyi w j f j (x ) ij11 • Improvements : (Noçairi, Gomes, Thomas & S , 2016) • Nonnegative coefficients adding to 1 • Regularised regression (eg PLS) since predictions are usually highly correlated ASMDA 2017 30 Empirical validation • Combining Machine Learning and Statistics • A good model must give good predictions • Bootstrap, cross-validation, etc. • Learning and validation sets ASMDA 2017 31 The three samples procedure for selecting a model inside a family of models • Learning set: estimate parameters for all models in competition • Test set : choice of the best model in terms of prediction • NB Reestimation of the final model: with all available observations • Validation set : estimate the performance for future data. « Generalization » • Parameter estimation ≠ performance estimation ASMDA 2017 32 • One split is not enough! ASMDA 2017 33 • Elementary? • Not that sure… • Have a look on publications in econometrics, epidemiology, .. prediction is rarely checked on a hold-out sample (except in time series forecasting) • Forerunners: • « the usefulness of a prediction procedure is not established when it is found to predict adequately on the original sample; the necessary next step must be its application to at least a second group. Only if it predicts adequately on subsequent samples can the value of the procedure be regarded as established » Horst, 1941 • Leave one out : Lachenbruch et Mickey,
Details
-
File Typepdf
-
Upload Time-
-
Content LanguagesEnglish
-
Upload UserAnonymous/Not logged-in
-
File Pages52 Page
-
File Size-