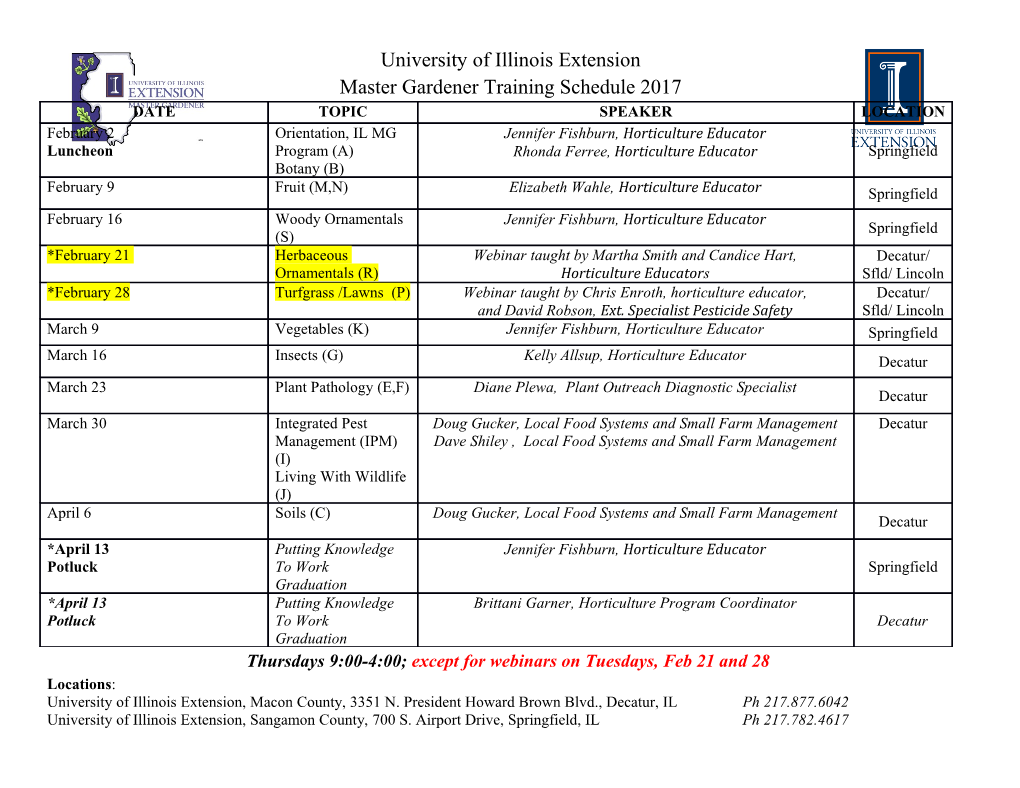
Data Based Identification and Prediction of Nonlinear and Complex Dynamical Systems Wen-Xu Wanga;b, Ying-Cheng Laic;d;e, and Celso Grebogie a School of Systems Science, Beijing Normal University, Beijing, 100875, China b Business School, University of Shanghai for Science and Technology, Shanghai 200093, China c School of Electrical, Computer and Energy Engineering, Arizona State University, Tempe, Arizona 85287, USA d Department of Physics, Arizona State University, Tempe, Arizona 85287, USA e Institute for Complex Systems and Mathematical Biology, King’s College, University of Aberdeen, Aberdeen AB24 3UE, UK arXiv:1704.08764v1 [physics.data-an] 27 Apr 2017 Wen-Xu Wang, Ying-Cheng Lai, and Celso Grebogi 1 Abstract The problem of reconstructing nonlinear and complex dynamical systems from measured data or time series is central to many scientific disciplines including physical, biological, computer, and social sciences, as well as engineering and economics. The classic approach to phase-space re- construction through the methodology of delay-coordinate embedding has been practiced for more than three decades, but the paradigm is effective mostly for low-dimensional dynamical systems. Often, the methodology yields only a topological correspondence of the original system. There are situations in various fields of science and engineering where the systems of interest are complex and high dimensional with many interacting components. A complex system typically exhibits a rich variety of collective dynamics, and it is of great interest to be able to detect, classify, under- stand, predict, and control the dynamics using data that are becoming increasingly accessible due to the advances of modern information technology. To accomplish these tasks, especially prediction and control, an accurate reconstruction of the original system is required. Nonlinear and complex systems identification aims at inferring, from data, the mathematical equations that govern the dynamical evolution and the complex interaction patterns, or topology, among the various components of the system. With successful reconstruction of the system equa- tions and the connecting topology, it may be possible to address challenging and significant prob- lems such as identification of causal relations among the interacting components and detection of hidden nodes. The “inverse” problem thus presents a grand challenge, requiring new paradigms beyond the traditional delay-coordinate embedding methodology. The past fifteen years have witnessed rapid development of contemporary complex graph the- ory with broad applications in interdisciplinary science and engineering. The combination of graph, information, and nonlinear dynamical systems theories with tools from statistical physics, optimization, engineering control, applied mathematics, and scientific computing enables the de- velopment of a number of paradigms to address the problem of nonlinear and complex systems reconstruction. In this Review, we review the recent advances in this forefront and rapidly evolv- ing field, with a focus on compressive sensing based methods. In particular, compressive sensing is a paradigm developed in recent years in applied mathematics, electrical engineering, and nonlin- ear physics to reconstruct sparse signals using only limited data. It has broad applications ranging from image compression/reconstruction to the analysis of large-scale sensor networks, and it has become a powerful technique to obtain high-fidelity signals for applications where sufficient ob- servations are not available. We will describe in detail how compressive sensing can be exploited to address a diverse array of problems in data based reconstruction of nonlinear and complex net- worked systems. The problems include identification of chaotic systems and prediction of catas- trophic bifurcations, forecasting future attractors of time-varying nonlinear systems, reconstruction of complex networks with oscillatory and evolutionary game dynamics, detection of hidden nodes, identification of chaotic elements in neuronal networks, and reconstruction of complex geospatial networks and nodal positioning. A number of alternative methods, such as those based on system response to external driving, synchronization, noise-induced dynamical correlation, will also be discussed. Due to the high relevance of network reconstruction to biological sciences, a special Section is devoted to a brief survey of the current methods to infer biological networks. Finally, a number of open problems including control and controllability of complex nonlinear dynamical networks are discussed. Wen-Xu Wang, Ying-Cheng Lai, and Celso Grebogi 2 The methods reviewed in this Review are principled on various concepts in complexity science and engineering such as phase transitions, bifurcations, stabilities, and robustness. The method- ologies have the potential to significantly improve our ability to understand a variety of complex dynamical systems ranging from gene regulatory systems to social networks towards the ultimate goal of controlling such systems. Wen-Xu Wang, Ying-Cheng Lai, and Celso Grebogi 3 Contents 1 Introduction 5 1.1 Existing works on data based reconstruction of nonlinear dynamical systems . .5 1.2 Existing works on data based reconstruction of complex networks and dynamical processes . .6 1.3 Compressive sensing based reconstruction of nonlinear and complex dynamical systems . .7 1.4 Plan of this review . .8 2 Compressive sensing based nonlinear dynamical systems identification 12 2.1 Introduction to the compressive sensing algorithm . 12 2.2 Mathematical formulation of systems identification based on compressive sensing . 14 2.3 Reconstruction and identification of chaotic systems . 15 2.4 Predicting catastrophe in nonlinear dynamical systems . 18 2.4.1 Predicting catastrophic bifurcations based on compressive sensing . 18 2.4.2 Using compressive sensing to predict tipping points in complex systems . 19 2.5 Forecasting future states (attractors) of nonlinear dynamical systems . 22 3 Compressive sensing based reconstruction of complex networked systems 29 3.1 Reconstruction of coupled oscillator networks . 29 3.2 Reconstruction of complex networks with evolutionary-game dynamics . 35 3.3 Detection of hidden nodes in complex networks . 42 3.3.1 Principle of detecting hidden nodes based on compressive sensing . 42 3.3.2 Mathematical formulation of compressive sensing based detection of a hid- den node . 44 3.3.3 Examples of hidden node detection in the presence of noise . 48 3.4 Identifying chaotic elements in neuronal networks . 57 3.4.1 Basic procedure of identifying chaotic neurons . 59 3.4.2 Example: identifying chaotic neurons in the FitzHugh-Nagumo (FHN) network . 60 3.5 Data based reconstruction of complex geospatial networks and nodal positioning . 63 3.6 Reconstruction of complex spreading networks from binary data . 70 3.6.1 Mathematical formulation . 71 3.6.2 Reconstructing complex spreading networks: examples . 77 4 Alternative methods for reconstructing complex, nonlinear dynamical networks 83 4.1 Reconstructing complex networks from response dynamics . 83 4.2 Reconstructing complex networks via system clone . 84 Wen-Xu Wang, Ying-Cheng Lai, and Celso Grebogi 4 4.3 Network reconstruction via phase-space linearization . 85 4.4 Reconstruction of oscillator networks based on noise induced dynamical correlation 86 4.5 Reverse engineering of complex systems . 91 5 Inference approaches to reconstruction of biological networks 94 5.1 Correlation based methods . 94 5.1.1 Value based methods . 94 5.1.2 Rank based methods . 95 5.2 Causality based measures . 96 5.3 Information-theoretic based methods . 98 5.3.1 Mutual information . 98 5.3.2 Maximal information coefficient . 99 5.3.3 Maximum entropy . 100 5.4 Bayesian network . 100 5.5 Regression and resampling . 100 5.6 Supervised and semi-supervised methods . 101 5.7 Transfer and joint entropies . 101 5.8 Distinguishing between direct and indirect interactions . 102 6 Discussions and future perspectives 104 6.1 Localization of diffusion sources in complex networks . 105 6.2 Data based reconstruction of complex networks with binary-state dynamics . 106 6.3 Universal structural estimator and dynamics approximator for complex networks . 108 6.4 Controlling nonlinear and complex dynamical networks . 109 Wen-Xu Wang, Ying-Cheng Lai, and Celso Grebogi 5 1 Introduction An outstanding problem in interdisciplinary science is nonlinear and complex systems identifica- tion, prediction, and control. Given a complex dynamical system, the various types of dynamical processes are of great interest. The ultimate goal in the study of complex systems is to devise prac- tically implementable strategies to control the collective dynamics. A great challenge is that the network structure and the nodal dynamics are often unknown but only limited measured time series are available. To control the system dynamics, it is imperative to map out the system details from data. Reconstructing complex network structure and dynamics from data, the inverse problem, has thus become a central issue in contemporary network science and engineering [1–38]. There are broad applications of the solutions of the network reconstruction problem, due to the ubiquity of complex interacting patterns arising from many systems in a variety of disciplines [39–42]. 1.1 Existing works on data based reconstruction of nonlinear dynamical systems The traditional paradigm of nonlinear time series analysis is the delay-coordinate embedding method,
Details
-
File Typepdf
-
Upload Time-
-
Content LanguagesEnglish
-
Upload UserAnonymous/Not logged-in
-
File Pages138 Page
-
File Size-