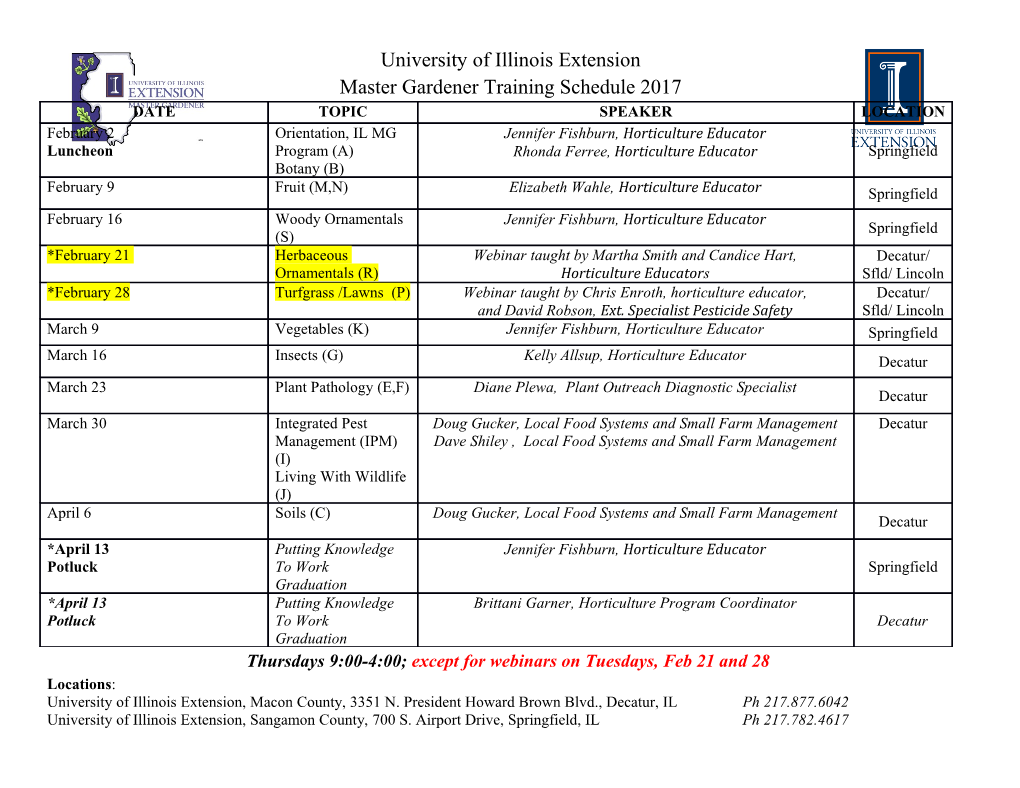
Amazon SageMaker Ground Truth 第9回 Amazon SageMaker 事例祭り #sagemaker_fes 大渕 麻莉 アマゾン ウェブ サービス ジャパン株式会社 機械学習 ソリューション アーキテクト October 30, 2019 © 2019, Amazon Web Services, Inc. or its Affiliates. 本セッションで話すこと • 機械学習のワークフローとアノテーション (5分) • Amazon SageMaker Ground Truth (15分) • 6種類の組み込みラベリングツールと3種類のワーカー • 自動ラベリングとラベルの統合 • ラベリングジョブの作り方 • Demo (25分) © 2019, Amazon Web Services, Inc. or its Affiliates. 機械学習のワークフロー © 2019, Amazon Web Services, Inc. or its Affiliates. 機械学習の一般的なワークフロー データ収集 クリーン モデルのデプロイ 推論・監視 学習データの生成 アップ 本番環境に データ変換・ デプロイ ラベル付け モデル評価 トレーニング モデルの学習 © 2019, Amazon Web Services, Inc. or its Affiliates. 教師あり学習 入力データ モデル (Neural Network) 予測値 “イヌ” 勾配 損失 © 2019, Amazon Web Services, Inc. or its Affiliates. 機械学習の一般的なワークフロー 教師あり学習では ラベル付け (アノテーション) データ収集 が必要 クリーン 推論・監視 アップ 本番環境に データ変換・ デプロイ ラベル付け モデル評価 トレーニング © 2019, Amazon Web Services, Inc. or its Affiliates. Amazon SageMaker Ground Truth © 2019, Amazon Web Services, Inc. or its Affiliates. ラベル付けの難しさ • 深層学習には大規模なデータセットが必要 • 大量のデータへのラベル付けはしんどい • ラベル付けの精度も求められる • 時間もお金もかかる • ツールの準備 (認証・UI・進捗管理) © 2019, Amazon Web Services, Inc. or its Affiliates. Amazon SageMaker Ground Truth 簡単かつ正確にデータセットのラベル付けができるサービス • アノテーションの一般的なワークフローをサポート • 6種類の組み込みラベリングツールを提供 • アノテーション作業を行うワーカーとの連携・管理機能を提供 • 大規模データセットに対しては自動ラベリング機能で最大70%のコスト削減 迅速なラベル付け ワーカーとの連携が容易 高精度 © 2019, Amazon Web Services, Inc. or its Affiliates. 6種類の組み込みラベリングツール + カスタムジョブ New! カスタム © 2019, Amazon Web Services, Inc. or its Affiliates. カスタムラベリングのワークフロー AWS Lambda AWS Lambda © 2019, Amazon Web Services, Inc. or its Affiliates. 3種類のワーカーが選択可能 • パブリック • Amazon Mechanical Turk で 24時間 x 365日、50万人以上のワーカー • プライベート • 社員などを登録。データを組織内でのみ使用 • ワーカー管理は Amazon Cognito を利用 (OIDC や SAML とも連携可) • ベンダー • AWS Marketplace 登録済みの 3rd パーティーベンダーに依頼 © 2019, Amazon Web Services, Inc. or its Affiliates. アクティブラーニングと自動データラベリング 自動で ラベル付け 入力データセット アクティブ 精度の高い ラーニング 学習用データセット 信頼度の低いデータは 人間がアノテーション 人がラベル付けしたデータから アクティブラーニングのモデルを学習 © 2019, Amazon Web Services, Inc. or its Affiliates. アクティブラーニングと自動データラベリング 自動で ラベル付け 入力データセット アクティブ 精度の高い ラーニング 学習用データセット 信頼度の低いデータは アノテーターの評価は!? 人間がラベル付け © 2019, Amazon Web Services, Inc. or its Affiliates. ラベルの決定 (Label Consolidation): 多数決の場合 bulldog sharpei bulldog bulldog bulldog © 2019, Amazon Web Services, Inc. or its Affiliates. ラベルの決定 (Label Consolidation): 正答率による評価 (犬に詳しい人) 正しい ラベルを 選ぶ確率 0.7 0.9 0.5 0.3 bulldog sharpei bulldog bulldog bulldog 0.1 sharpei 0.9 • 単純な多数決ではなく、ワーカーの正答率をもとにラベルを決定することで アノテーションの質を担保 • 何人のワーカーの結果をまとめるかはジョブ作成時に指定 © 2019, Amazon Web Services, Inc. or its Affiliates. ラベリングジョブの作り方 © 2019, Amazon Web Services, Inc. or its Affiliates. ラベリングジョブ作成の4ステップ 1. データセットの準備 2. タスクの定義 3. ワーカーの選択 4. ラベリングツールの設定 © 2019, Amazon Web Services, Inc. or its Affiliates. 1. データセットの準備 • データと結果を保存するためのS3バケットを用意 • アノテーション対象の画像群をS3バケットに保存 (テキスト分類の場合は Text/CSV ファイル) • 画像群のパスを記述したマニフェストファイルを作成し、S3バケットに保存 (マニフェストファイルは自動生成も可能) input.manifest Amazon S3 JSON Lines フォーマット © 2019, Amazon Web Services, Inc. or its Affiliates. 1. データセットの準備 (マニフェストファイル) input.manifest {"source-ref": "s3://sagemaker-groundtruth-demo/SSDB00001.JPG"} {"source-ref": "s3://sagemaker-groundtruth-demo/SSDB00002.JPG"} {"source-ref": "s3://sagemaker-groundtruth-demo/SSDB00003.JPG"} {"source-ref": "s3://sagemaker-groundtruth-demo/SSDB00004.JPG"} {"source-ref": "s3://sagemaker-groundtruth-demo/SSDB00005.JPG"} {"source-ref": "s3://sagemaker-groundtruth-demo/SSDB00006.JPG"} {"source-ref": "s3://sagemaker-groundtruth-demo/SSDB00007.JPG"} “source-ref” で S3 のパスを指定。 あるいは “source” に直接テキストを書くこともできる © 2019, Amazon Web Services, Inc. or its Affiliates. 2. タスクの定義 • 画像 • 画像分類 • 物体検出 • セマンティック セグメンテーション • ラベルの検証 • テキスト • テキスト分類 • 固有表現抽出 • あるいはユーザ定義の カスタムタスク © 2019, Amazon Web Services, Inc. or its Affiliates. 3. ワーカーの選択 • Public • Amazon Mechanical Turkを利用 • Private • 社員などワーカーを自ら調達 •ベンダー • 3rd パーティーベンダーに依頼 • 追加オプションも選べる • 自動ラベリング • 複数ワーカーによる ラベル付け © 2019, Amazon Web Services, Inc. or its Affiliates. 4. ラベリングツールの設定 • アノテーションの指示書を書く • 良い例・悪い例を記載 • ラベルを設定 © 2019, Amazon Web Services, Inc. or its Affiliates. 出力 (拡張マニフェストファイル) output.manifest { "source-ref": "s3://sagemaker-groundtruth-demo/SSDB00001.JPG", "GroundTruthDemo": { "annotations": [ {"class_id": 0, "width": 54, "top": 482, "height": 39, "left": 337}, {"class_id": 0, "width": 69, "top": 495, "height": 53, "left": 461}, {"class_id": 0, "width": 52, "top": 482, "height": 41, "left": 523} ], "image_size": [{"width": 1280, "depth": 3, "height": 960} ] }, "GroundTruthDemo-metadata": { "job-name": "labeling-job/groundtruthdemo", "class-map": {"0": "Car"}, "human-annotated": "yes", "objects": [ {"confidence": 0.94}, {"confidence": 0.94}, {"confidence": 0.94},], "creation-date": "2018-11-26T04:01:09.038134", © 2019, Amazon"type": Web Services, "groundtruth/objectInc. or its Affiliates. -detection" } } 拡張マニフェストファイルをトレーニングジョブに渡す notebook.ipynb 詳しくはドキュメント「拡張マニフェストファイルを使用してトレーニングジョブにデータセットメタデータを提供する」 © 2019, Amazon Web Services, Inc. or its Affiliates. Demo © 2019, Amazon Web Services, Inc. or its Affiliates. ジョブ一覧 © 2019, Amazon Web Services, Inc. or its Affiliates. ジョブを作成してみる © 2019, Amazon Web Services, Inc. or its Affiliates. ワーカーのログイン後 © 2019, Amazon Web Services, Inc. or its Affiliates. アノテーション画面 © 2019, Amazon Web Services, Inc. or its Affiliates. 出力プレビュー (Augmented Manifest ファイル) © 2019, Amazon Web Services, Inc. or its Affiliates. アノテーションしたデータをビルトインアルゴリズムに © 2019, Amazon Web Services, Inc. or its Affiliates. Amazon SageMaker Ground Truth お客様事例 https://aws.amazon.com/jp/sagemaker/groundtruth/customers/ © 2019, Amazon Web Services, Inc. or its Affiliates. 価格と提供リージョン 価格 • ラベル付けした対象の数に応じた利⽤料 $0.08 / 個 (5万個以上はより安 価) • Amazon Mechanical Turk および 外部ベンダの利⽤料 • ⾃動ラベリング利⽤時は Amazon SageMaker での学習/推論の利⽤料 • 詳細は「Amazon SageMaker Ground Truth の料⾦」 提供リージョン • アジアパシフィック (東京・ソウル・ムンバイ・シンガポール・シド ニー)・カナダ (中部)・EU (フランクフルト・アイルランド・ロンドン)・ ⽶国東部 (バージニア北部・オハイオ)・⽶国⻄部 (オレゴン) © 2019, Amazon Web Services, Inc. or its Affiliates. SageMaker Ground Truth まとめ • データに正解ラベル (Ground Truth) を付与するのためのサービス • 6種類の組み込みラベリングツール • ラベル付け作業を行うワーカー (Public/Private/3rd party) との連携・管理 • 大規模データセットに対しては自動ラベリング機能で最大70%のコスト削減 • Example • Amazon SageMaker Examples にいくつかあります (英語) • 日本語サンプル「SageMaker Ground Truth カスタムラベリング キーポ イント」 • ブログ(固有表現抽出がサポートされる前)「Amazon SageMaker Ground Truth を使ったカスタムデータラベリングワークフローの構築」 © 2019, Amazon Web Services, Inc. or its Affiliates. Thank you! [email protected] © 2019, Amazon Web Services, Inc. or its Affiliates. .
Details
-
File Typepdf
-
Upload Time-
-
Content LanguagesEnglish
-
Upload UserAnonymous/Not logged-in
-
File Pages36 Page
-
File Size-