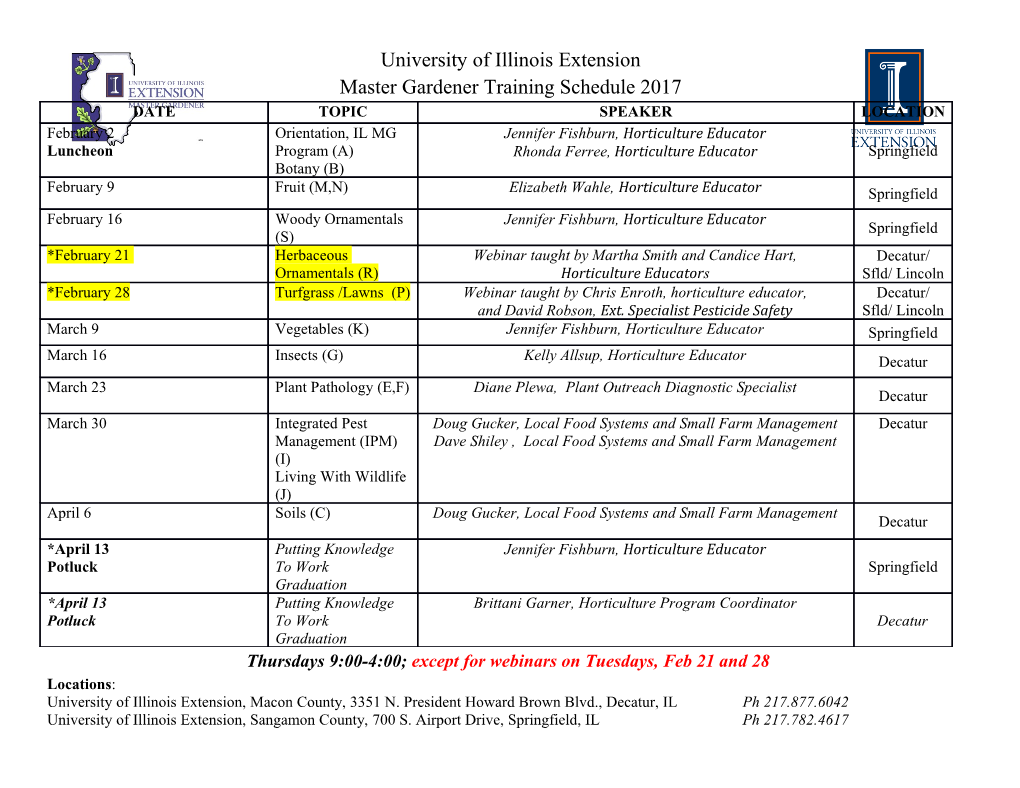
Electrochemical Model-Based State of Charge and State of Health Estimation of Lithium-Ion Batteries Dissertation Presented in Partial Fulfillment of the Requirements for the Degree of Doctor of Philosophy in the Graduate School of The Ohio State University By Alexander Paul Bartlett, M.S. Graduate Program in Mechanical Engineering ***** The Ohio State University 2015 Dissertation Committee Giorgio Rizzoni, Advisor Marcello Canova Terry Conlisk Yann Guezennec © Copyright by Alexander Paul Bartlett 2015 Abstract Vehicle electrification continues to be a key topic of interest for automotive manu- facturers, in an effort to reduce the usage of fossil fuel energy and improve vehicle efficiency. Lithium-ion batteries are currently the technology of choice for hybrid and electric vehicles due to their decreasing cost and improved power and energy density over traditional lead-acid or nickel-metal-hydride batteries. In particular, batteries with composite electrodes have seen increased use in automotive applications due to their ability to balance energy density, power density, and cost by adjusting the amount of each material within the electrode. However, this improved performance introduces new challenges to ensure the battery pack operates safely, reliably, and durably. The vehicle's battery management system (BMS) is designed to meet these challenges, in part, by estimating the battery state of charge (SOC) and state of health (SOH). Knowledge of SOC allows the BMS to predict the available instanta- neous power while ensuring the battery is operating within safe limits. As batteries age, they lose capacity and the ability to deliver power. Therefore, tracking the bat- tery SOH is necessary to maintain accurate estimates of SOC and power throughout the battery life and give an accurate miles-to-empty metric to the driver. Recently, increased attention has been given to electrochemical models for SOC and SOH estimation, over traditional circuit models. Electrochemical models based on first principles have the potential to more accurately predict cell performance, as well as provide more information about the internal battery states. State of health estimation algorithms that do not use electrochemical-based models may have more ii difficulty maintaining an accurate battery model as the cell ages under varying degra- dation modes such as lithium consumption at the solid-electrolyte interface or active material dissolution. However, efforts to validate electrochemical model-based state estimation algorithms with experimental aging data are limited, particularly for com- posite electrode cells. This dissertation focuses on applying electrochemical models towards SOC and SOH estimation problems, specifically on automotive cells with a composite LiMn2O4- LiNi1=3Mn1=3Co1=3O2 (LMO-NMC) cathode. Model order reduction techniques are applied to reduce the computational complexity of the governing electrochemical equations, while still maintaining physically meaningful parameters. The observ- ability of the reduced order model is analyzed in order to facilitate state estimation, while also determining the limitations on the number of capacity and power related parameters that can be estimated simultaneously. Various nonlinear observers are implemented to estimate the model states associated with SOC and SOH, in order to determine their applicability in an onboard battery management system. Finally, SOC and SOH estimates are validated with experimental aging data. iii Acknowledgements I would like to take the time to properly thank everyone that contributed to this work, as I could not have done it alone. I would like to thank my advisor, Dr. Rizzoni, for giving me this incredible opportunity here at CAR, and for all of the advice over the years. A special thanks is due for always providing excellent coffee at our meetings and for the golf tips, including helping me learn not to over-swing. I would also like to thank Dr. Canova, Dr. Conlisk, and Dr. Guezennec for serving on my dissertation committee, and for their assistance with the modeling work and experiments. I am especially grateful to my former fellow grad student Jim Marcicki for being an amazingly patient and helpful mentor, always taking the time to help me under- stand the world of battery modeling, and continuing to offer advice and suggestions throughout my graduate career. I would like to thank my colleges at CAR, especially Guodong, Ke, Samartha, Shrikant, Meng, Chuting, and Dell for all of our enlightening conversations about various battery and non-battery related topics. Also, special thanks to Nick Warner for setting up and running the aging experiments and to Dr. Co and my friend Jon Goiri for patiently trying to explain chemistry to this clueless mechanical engineer. I want to thank Ford Motor Company for their support via the Ford University Research Program (URP), as well as for the opportunities for two summer internships. iv A special thanks to my colleges at Ford for all of their help, including Ted Miller, Xiao Guang Yang, Kevin Rhodes, and OuJung Kwon. Finally, and most importantly, I would like to thank my family for everything they have done for me. A special thanks to my Mom and Dad for their love, support, advice, and constant reminders not to work too hard, my brother Andy and sister- in-law Michele for making these four years a more cromulent experience, and my Grandparents for their constant cheering from the sidelines. Also, thanks to my friend Peter for helping me keep my sanity, and to the friendly staff at Reno's East. Thanks for finally getting some decent darts. v Vita May 20, 1988 ....................................... Born - Columbus, Ohio 2011 ..................................................... B.S. Mechanical Engineering, Summa Cum Laude, University of Michigan 2014 ..................................................... M.S. Mechanical Engineering, The Ohio State University 2014 ..................................................... Graduate Student, The Ohio State University Publications A. Bartlett, J. Marcicki, S. Onori, G. Rizzoni, X. Yang, T. Miller, \Model-Based State of Charge Estimation and Observability Analysis of a Composite Electrode Lithium-Ion Battery", Proceedings of the 52nd IEEE Conference on Decision and Control, Florence, Italy, 2013. A. Bartlett, J. Marcicki, K. Rhodes, G. Rizzoni, \State of Health Estimation in Composite Electrode Lithium-Ion Cells", Journal of Power Sources, 284, p. 642-649, 2015. A. Bartlett, J. Marcicki, S. Onori, G. Rizzoni, X. Yang, T. Miller, \Electrochemi- cal Model-Based State of Charge and Capacity Estimation for a Composite Electrode Lithium-Ion Battery", Submitt. IEEE Transactions on Control Systems Technology, 2014. Fields of Study Major Field: Mechanical Engineering vi Table of Contents Abstract . ii Acknowledgements . iv Vita ....................................... vi Table of Contents . vii List of Tables . .x List of Figures . xi 1 Introduction and Overview 1 1.1 An Introduction to Vehicle Electrification . .2 1.2 Batteries for Energy Storage . .3 1.3 Types of Batteries . .6 1.4 Basic Function of Batteries . .7 1.5 Battery Electrodes . .9 1.6 Aging Mechanisms . 13 1.7 Battery Management System . 15 1.8 Basic Terminology . 17 1.9 Thesis Objectives . 19 2 Battery Modeling 21 2.1 Introduction . 21 2.2 A Hierarchy of Battery Models . 22 2.3 Nomenclature . 24 2.4 The Porous Electrode Model . 26 2.4.1 1+1D porous electrode model . 28 2.4.2 Governing electrochemical equations . 29 2.4.3 The open circuit voltage from thermodynamics . 29 2.4.4 Kinetic overpotential . 33 2.4.5 Mass transport in the spherical electrode particle and the con- centration overpotential . 40 2.4.6 Electrical conduction in the solid . 42 2.4.7 Lithium transport in the electrolyte . 42 2.4.8 Potential in the liquid . 50 2.4.9 Solution method . 52 2.5 The Single Particle Model . 53 2.6 Model Order Reduction . 54 vii 2.6.1 Solid diffusion equation . 56 2.6.2 Liquid diffusion equation . 63 2.7 Reduced Order Model in State Space . 68 2.7.1 Electrode state space dynamics . 70 2.7.2 Electrolyte state space dynamics . 75 2.7.3 Cell voltage output . 77 2.8 Electrochemical Models for State Estimation . 78 2.9 Summary . 82 3 Methods for State Estimation 84 3.1 Introduction to Estimation . 84 3.2 Observability . 86 3.2.1 Observability for linear systems . 87 3.2.2 Observability for nonlinear systems . 91 3.2.3 Observability of the single particle model . 95 3.3 Observer Design . 99 3.3.1 The extended Kalman filter . 101 3.3.2 The particle filter . 108 3.3.3 Other nonlinear observers . 113 3.4 Methods for Smoothing . 115 3.5 Combined State and Parameter Estimation . 121 3.6 Summary . 124 4 State of Charge Estimation 126 4.1 Introduction . 126 4.1.1 Defining state of charge . 127 4.1.2 Methods for state of charge estimation . 129 4.2 SOC Estimation for a Composite Electrode . 130 4.2.1 Experimental methods . 131 4.2.2 Calculating the current split . 133 4.2.3 Calculating a cell level SOC . 143 4.2.4 Initializing the electrodes . 147 4.3 Observability of the Complete Model and Possible Improvements . 148 4.3.1 Approach 1: Open-loop estimation of one electrode . 151 4.3.2 Approach 2: Steady-state approximation for one electrode . 153 4.3.3 Observability of open-loop and steady-state methods . 156 4.4 Validation of SOC Estimates . 162 4.5 Summary and Future Work . 166 5 State of Health Estimation 169 5.1 Introduction . 169 5.1.1 Defining state of health . 170 5.1.2 Methods for state of health estimation . 171 5.2 Estimating Active Material Loss . 172 viii 5.2.1 Observer design . 175 5.2.2 Experimental validation of active material estimates . 179 5.3 Estimating Loss of Cyclable Lithium . 193 5.3.1 Validation with experimental data . 202 5.3.2 Quantifying SOC/SOH Estimate Error . 212 5.4 Simultaneous Estimation of Degradation Mechanisms . 226 5.4.1 Combined active material/cyclable lithium estimation algorithm 228 5.5 Summary and Future Work .
Details
-
File Typepdf
-
Upload Time-
-
Content LanguagesEnglish
-
Upload UserAnonymous/Not logged-in
-
File Pages268 Page
-
File Size-