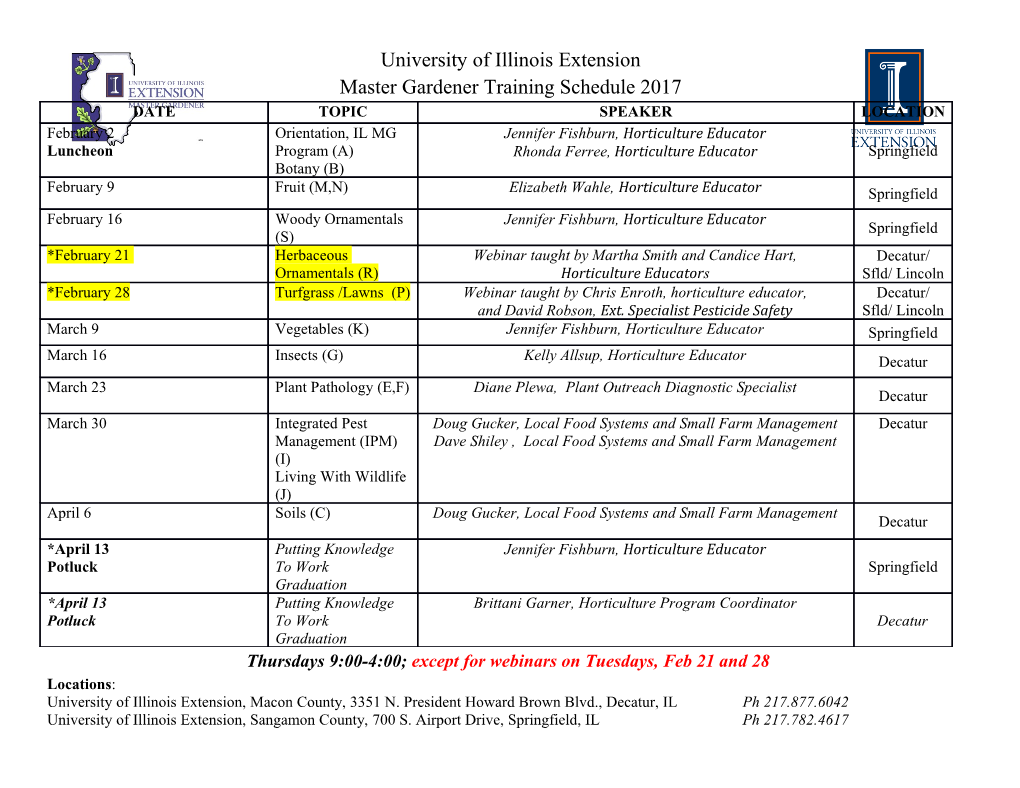
Journal Title 0 (0) 1 1 IOS Press 1 1 2 2 3 3 4 The euBusinessGraph Ontology: a 4 5 5 6 6 7 Lightweight Ontology for Harmonizing 7 8 8 9 Basic Company Information 9 10 10 11 11 a;∗ b c a 12 Dumitru Roman , Vladimir Alexiev , Javier Paniagua , Brian Elvesæter , 12 a a b d 13 Bjørn Marius von Zernichow , Ahmet Soylu , Boyan Simeonov and Chris Taggart 13 14 a SINTEF AS, Norway 14 15 E-mail: {firstname.lastname}@sintef.no 15 16 b Ontotext, Bulgaria 16 17 E-mail: {firstname.lastname}@ontotext.com 17 18 c SpazioDati, Italy 18 19 E-mail: [email protected] 19 20 d OpenCorporates, UK 20 21 E-mail: [email protected] 21 22 22 23 Abstract. Company data, ranging from basic company information such as company name(s) and incorporation date to complex 23 24 balance sheets and personal data about directors and shareholders, are the foundation that many data value chains depend upon 24 in various sectors (e.g., business information, marketing and sales, etc.). Company data becomes a valuable asset when data 25 25 is collected and integrated from a variety of sources, both authoritative (e.g., national business registers) and non-authoritative 26 (e.g., company websites). Company data integration is however a difficult task primarily due to the heterogeneity and complexity 26 27 of company data, and the lack of generally agreed upon semantic descriptions of the concepts in this domain. In this article, 27 28 we introduce the euBusinessGraph ontology as a lightweight mechanism for harmonising company data for the purpose of 28 aggregating, linking, provisioning and analysing basic company data. The article provides an overview of the related work, 29 ontology scope, ontology development process, explanations of core concepts and relationships, and the implementation of 29 30 the ontology. Furthermore, we present scenarios where the ontology was used, among others, for publishing company data 30 31 (business knowledge graph) and for comparing data from various company data providers. The euBusinessGraph ontology 31 serves as an asset not only for enabling various tasks related to company data but also on which various extensions can be built 32 upon. 32 33 33 Keywords: Company data, Open data, Linked data, Ontology, Business knowledge graph 34 34 35 35 36 36 37 37 38 1. Introduction 38 39 39 Corporate information, including basic company information (e.g., name(s), incorporation data, reg- 40 40 istered addresses, ownership and related entities, etc.), financials (e.g., balance sheets, ratings, etc.) as 41 41 well as contextual data (e.g., cadastral data on corporate properties, geo data, personal data about direc- 42 42 tors and shareholders, public tenders data, etc.) are the foundation that many data value chains depend 43 43 upon in different sectors. The most evident examples of sectors are the business information sector, the 44 44 45 *Corresponding author. E-mail: [email protected]. 45 46 46 0000-0000/0-1900/$00.00 c 0 – IOS Press and the authors. All rights reserved 2 D. Roman et al. / euBusinessGraph ontology 1 marketing and sales sector and the business publishing industry. At the same time, the use of company 1 2 data is extremely significant in many other business sectors and societal activities including transparency 2 3 and accountability [1]. 3 4 Recently, a number of initiatives have been established to harmonise and increase the interoperability 4 5 of corporate and financial data across national borders, including public initiatives such as the Global 5 6 Legal Entity Identification System—GLEIS1, Bloomberg’s open FIGI system for securities2, as well 6 7 as long-established proprietary initiatives such as the Dun & Bradstreet DUNS number3. Other notable 7 8 initiatives include the European Business Register (EBR)4, which aims to federate several national busi- 8 9 ness registers in order to offer a unique point of access, and BREX5, which “wraps” the EBR, extends its 9 10 country coverage and offers a pricing model to access the underlying data. Additionally there are estab- 10 11 lished and widespread adopted standardisation systems in the area of company financials (e.g., official 11 12 deposited and public balance sheets data, which is in most cases exchanged in the XBRL format6). How- 12 13 ever, due to various reasons including technical, operational and organizational limitations, the systems 13 14 and data sources mentioned above are mostly fragmented across borders, limited in scope and size7, and 14 15 siloed within specific business communities with limited accessibility from outside their originating sec- 15 16 tors. For example, register exchanges only offer access to official national registry data, not linked to any 16 17 other contextual datasets (i.e., there is no obvious way of following a link from a company’s registered 17 18 data to a tender it has won in another country), nor among themselves across countries (which means 18 19 that there is no “machine-readable” and easy way to follow, for example, a shareholding relationship 19 20 from an individual to companies in two different countries). 20 21 As a result, collecting and aggregating information about a business entity from several public sources 21 22 (official and non-official ones, such as public tender registries, press mentions of companies and related 22 23 entities, cadastral records, etc.), and especially across borders and languages is a tedious and very ex- 23 24 24 pensive task which renders many potential business models non-feasible. As a step in addressing this 25 25 challenge, governments and other public bodies are increasingly publishing open data about firmograph- 26 26 ics and contextual databases, which reference companies. For example, the UK, Norway, France, and 27 27 Denmark make the public records about companies available as open data, and other countries have dif- 28 28 ferent degrees of openness for their company registries8. Examples of contextual databases include the 29 29 EU TED (Tenders Electronic Daily) public procurement notices9, gazette notices, Horizon 2020 project 30 30 data10, and Structural Funds11. Unfortunately, firmographics datasets are not yet fully harmonised and 31 31 32 interoperable because data differs widely in semantics from one source to the other, and due to data 32 33 formats ranging from UK’s five star Linked Data [2] to poorly accessible and poorly documented ones. 33 34 Furthermore, contextual databases are not linked to the company registries and they still use different 34 35 35 1 36 https://www.gleif.org 36 2https://en.wikipedia.org/wiki/Financial_Instrument_Global_Identifier 37 37 3http://www.dnb.com/duns-number.html 38 4http://www.ebr.org 38 39 5https://brex.io 39 40 6https://www.xbrl.org 40 41 7Less than 1.6M companies worldwide were assigned a Legal Entity Identifier (LEI) number as of December 2019 (https: 41 42 //search.gleif.org) and these are only used in financial transactions of certain kind. 42 8 43 https://index.okfn.org/dataset/companies and http://registries.opencorporates.com 43 9https://ted.europa.eu 44 44 10https://data.europa.eu/euodp/en/data/dataset/cordisH2020projects 45 11https://cohesiondata.ec.europa.eu 45 46 46 D. Roman et al. / euBusinessGraph ontology 3 1 identifier systems or, in some cases, no identifiers at all. Private businesses are also producers of valu- 1 2 able company-related data, which is seldom linked to the public sources mentioned above. For example, 2 3 media publishers often reference businesses and legal entities by name (hence ambiguously) even within 3 4 their digital publications (with the exception of traded company tickers, which are sometimes used by 4 5 specialised financial publishers), because there isn’t any widespread markup schema to annotate a digital 5 6 reference to a company, nor a standardised way of accessing its information once it is unambiguously 6 7 identified. As a result, it is extremely expensive, time consuming and error prone to find, interpret and 7 8 reconcile these data from private sector sources. One of the immediate consequences is that the busi- 8 9 ness information sector is very cost-inefficient in itself, which is reflected in a lack of transparency and 9 10 efficiency of the markets. Nevertheless, the most relevant consequence in this context is that these inef- 10 11 ficiencies severely harm digital innovation across sectors, which is often introduced by small and agile 11 12 actors (e.g., startups, civil society organizations) who lack the capacity to invest time and resources in 12 13 overcoming these problems. 13 14 In this article, we follow the established approach for harmonizing and integrating data based on 14 15 ontologies (e.g., [3, 4]). In particular, we develop an ontology—the euBusinessGraph ontology—for 15 16 harmonising and integrating basic company information12. The ontology is meant to be used as a key 16 17 mechanism for aggregating, linking, provisioning and analysing company-related data. In this paper we 17 18 provide an overview of the related work, ontology scope, ontology development process, explanations 18 19 of core concepts and relationships, implementation of the ontology, and examples of scenarios where 19 20 the ontology was used, among others, for publishing company data (business knowledge graph) and 20 21 for comparing data from various company data providers. The main challenge this paper addresses is 21 22 related to striking the right balance between a semantic model for basic company information that is too 22 23 complex and hard to understand and a simplistic least common denominator model, while at the same 23 24 time exploiting proper mechanisms to reuse numerous related ontologies. 24 25 The remainder of the article is organised as follows.
Details
-
File Typepdf
-
Upload Time-
-
Content LanguagesEnglish
-
Upload UserAnonymous/Not logged-in
-
File Pages34 Page
-
File Size-