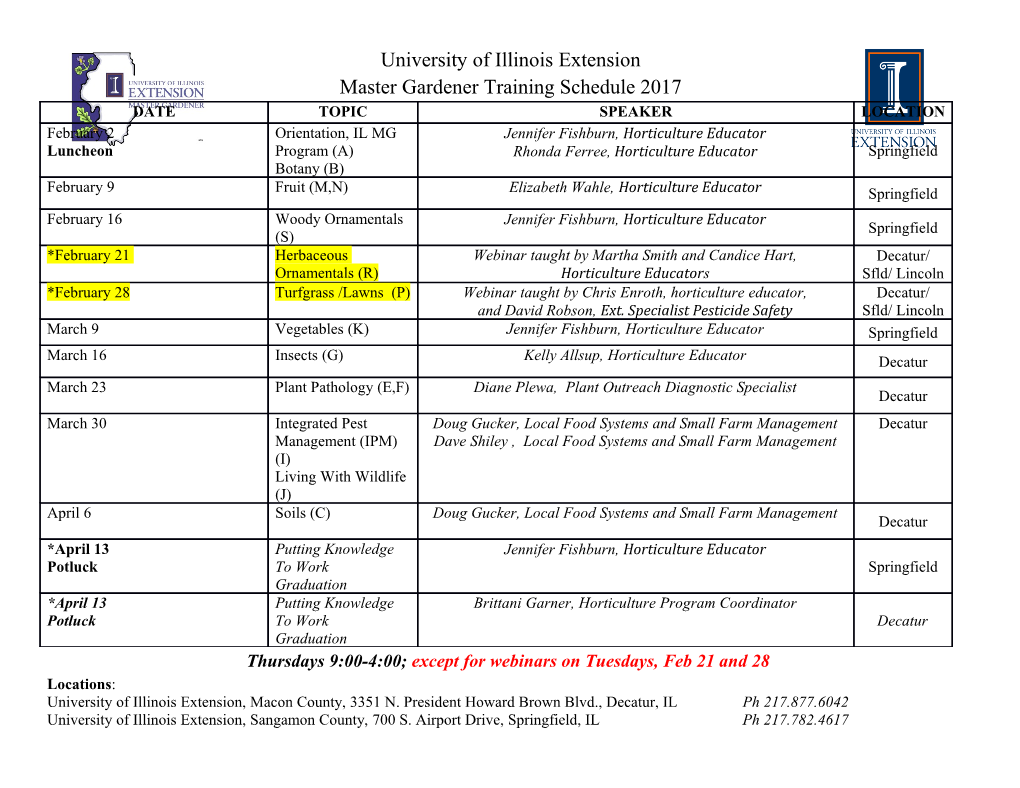
Sequence Analysis Introduction to Bioinformatics BIMMS December 2015 Gabriel Teku Department of Experimental Medical Science Faculty of Medicine Lund University Sequence analysis Part 1 • Sequence analysis: general introduction • Sequence features • Motifs and Domains Part 2 • Gala y • !MB"SS • Bioinformatics soft#are for sequence analysis Sequence analysis Part 1 • Sequence analysis: general introduction • Sequence features • Motifs and Domains Sequence analysis: definition $ refers to t%e &rocess of subjecting a DNA, RNA or peptide sequence to any of a #ide range of analytical met%ods to understand its features( function, structure, or evolution*** +%tt&://en.wi-i&edia.org,#iki/Sequence_analysis/ Quick sequence analysis example 1* "btain t%e &rotein sequence encoded by 0uman elastase gene from Uni&rot, P02234 2* "btain t%e 5DS sequence for t%e &rotein* %tt&:/,###.ebi.ac.u-,6ools/st 1* 6ranslate t%e 5DS sequence obtained above %tt&:/,###.ebi.ac.u-,6ools/st Quick sequence analysis example 3* 5om&are t%e translated 5DS to t%e &rotein sequence obtained from 1 above. %tt&:/,###.ebi.ac.u-,6ools/msa,clustalo, Quick sequence analysis example 3* 5om&are t%e translated 5DS to t%e &rotein sequence obtained from 1 above. %tt&:/,###.ebi.ac.u-,6ools/msa,clustalo, Types of sequence analysis Searching databases Sequence alignments Feature analyses Feature analysis Part 1 • General introduction • Feature analyses • Motifs and Domains What is a feature Sequence features are groups of nucleotides or amino acids that confer certain characteristics upon a gene or protein, and may be important for its overall function. %tt&://###.ebi.ac.u-,6ools,st Protein features Gene features Exercise on features 1* ! &lore t%e features along t%e &rotein P02234 #it%in UniProt 2* 8ie# the &rotein9s structure from &db by follo#ing t%e :D structure lin- for 1%1b. Quick exercise on motifs and domains 1* Identify t%e functional motif;s< of the &rotein P02234 Use P="SI6! lin- from Uni&rot → Family & Domains 2* ?%at is t%e motif as represented by t%e database entry Sequence analysis Part 1 • General introduction • Features • Motifs and Domains Motifs • Short, conserved sequence patterns • Associated to specific function(s) Binding site Active site • ~ 10 - 30 amino acids • Prosite Motifs Motifs: prosite From 5DS to &rotein sequence Statistically signi@cant motifs Functional motifs Protein family by virtue of similar functional sites Quick exercise on scanning for motifs 1* Use t%e &rotein sequence of t%e gene !ABC! to scan &rosite for motifs and domains. 2* 5om&are the results #it% that of t%e &revious exercise* Motifs: prosite Met%odology • Pattern development Pattern from literature Profiles Pattern development • Based on signature &atterns • Sensiti)ity • S&eci@city Pattern development • Aiterature curated &atterns &ublished curated tested against Swiss-Prot for specificity Pattern development • Cew &atterns start wit% review article alignment of proteins from article focus on biologically im&ortant regions create core pattern Pattern development • Cew &atterns ;contd< Search Swiss-Prot using core sites Retain/discard core &attern Refine core pattern and repeat searc% Patterns • Prosite syntax for &atterns: • one-letter codes for amino acids, e.g* GEGly • elements se&arated by a hy&hen, “DG • FHG used where any amino acid is accepted( Patterns Prosite syntax for &atterns contd: • Bmbiguities indicated by [ ]( e.g. +BG/ means Bla or Gly( • Bmino acids that are not accepted at a given position are listed between curly braces, “I JG( e.g. IBGJ means any amino acid e ce&t Bla and Gly( Patterns Prosite syntax for &atterns contd: • repetitions are &laced between braces,F( )G( e.g. +BG/;2(3< means Bla or Gly bet#een 2 and 3 times( • a &attern is anc%ored to the N-terminal or CDterminal by FKF and “LG( respectively* G H E G V G K V V K L G A G A G H E K K G Y F E D R G P S A G H E G Y G G R S R G G G Y S G H E F E G P K G C G A L Y I G H E L R G T T F M P A L E C G H E G V G K V V K L G A G A K K Y F E D R A P S S F Y G R S R G G Y I L E P K G C P L E C R T T F M G-H-E-X(2)-G –X(5)-[GA]-X(3) Quick exercise Interpret t%e motif you obtained from t%e &revious exercise. Motifs: prosite Met%odology • Pattern development Pattern from literature Ce# &atterns • Profiles Profiles Po&ular ap&roac%es • &osition #eig%t matrix • 0MM Position weight matrix 1 2 3 4 5 6 1 A T G T C G 2 A A G A C T 3 T A C T C A 1 2 3 4 5 6 Overall 4 C G G A G Gfreq. Pos. 5 A A C C T G A 0.6 0.6 - 0.4 - 0.2 0.30 T 0.2 0.2 - 0.4 0.2 0.2 0.20 G - 0.2 0.6 - 0.2 0.6 0.27 C 0.2 - 0.4 0.2 0.6 - 0.23 1 2 3 4 5 6 Overall freq. Pos. A 0.6 0.6 - 0.4 - 0.2 0.30 T 0.2 0.2 - 0.4 0.2 0.2 0.20 G - 0.2 0.6 - 0.2 0.6 0.27 C 0.2 - 0.4 0.2 0.6 - 0.23 1 2 3 4 5 6 Overall freq. Pos. A 2.0 2.0 - 1.33 - 0.67 0.30 T 1.0 1.0 - 2.0 1.0 1.0 0.20 G - 0.74 2.22 - 0.74 2.22 0.27 C 0.87 - 1.74 0.87 2.61 - 0.23 1 2 3 4 5 6 Overall freq. Pos. A 2.0 2.0 - 1.33 - 0.67 0.30 T 1.0 1.0 - 2.0 1.0 1.0 0.20 G - 0.74 2.22 - 0.74 2.22 0.27 C 0.87 - 1.74 0.87 2.61 - 0.23 1 2 3 4 5 6 Pos. A 1.0 1.0 - 0.41 - -0.58 T 0.0 0.0 - 1.0 0.0 0.0 G - -0.43 1.15 - -0.43 1.15 C -0.2 - 0.8 -0.2 1.38 - 1 2 3 4 5 6 Pos. A 1.0 1.0 - 0.41 - -0.58 T 0.0 0.0 - 1.0 0.0 0.0 G - -0.43 1.15 - -0.43 1.15 B B 5 6 5 G Sum of logs = 6.33 C -0.2 - 0.8 -0.2 1.38 - 1 2 3 4 5 6 Pos. A 1.0 1.0 - 0.41 - -0.58 T 0.0 0.0 - 1.0 0.0 0.0 G - -0.43 1.15 - -0.43 1.15 C -0.2 - 0.8 -0.2 1.38 - Profile • Multi&le sequence alignments #it% gaps • Gap &enalties • Pro@le E PSSM that includes gap &enalties • Fine tuning gap &arameters to achieve good &ro@les Building a profile: PSI-BLAST Query sequence BLAST MSA Profile A C B E 1 2 3 ... BLAST Additional homologs Iterate process Incorporated profile A C B E New profile 1 2 3 ... MEME Suite Example Quick exercise 1. BLAST the &rotein P02234 against the Uni&rot &roteins. 2. Select t%e first 5 hits and do#nload t%e sequences in fasta format :* Aaunch the MEME &rogram at %tt&:/,meme-suite.org, 3* Using the do#nloaded sequence file above, search for &ossible motifs using t%e M!M! &rogram* 5. Com&are t%e results to t%at from Prosite. 4* Aea)e the results o&en for later. Profiles from Hidden Markov Models • More efficient • From s&eech recognition • Based on Marko) Models • Statistical ap&roac% Some motif resources • P="SI6! • P=IC6S • SMB=6 • InterPro %tt&:,,###*ebi.ac*uk/inter&ro/about.%tml Domains • Aonger t%an motifs • conser)ed sequence &atterns • Inde&endent structural and functional unit • Bverage lengt%, 100 aa • May ;not< include motifs along boundries Domains • 0MM ap&lied in domain identi@cation due to its robustness* • Some domain databases include • PfamDB • PfamDB • Prodom • MEM! suite Quick exercise 1* Identify t%e domain;s< of t%e P02234 &rotein. 2* ! &lain %o# you accom&lished the tas-. PART 2 • Galaxy • !MB"SS • Bioinformatics soft#are for sequence analysis • "&en source tools • 5ommercial softwares Galaxy • %tt&s:/,usegala y*org, • OneDsto& sho& • from single sequence to CGS • Open source Sequence Analysis Introduction Introduction to galaxy %ttp:/,galaxy.bmc*lu.se, Introduction to galaxy Introduction to galaxy ?%ic% coding exon %as the %ig%est number of single nucleotide &olymor&%isms ;SCPs< on c%romosome 22N Introduction to galaxy Galaxy tutorial =egister Aogin Familiarize Galaxy tutorial https://github.com/nekrut/galaxy/wiki/Galaxy101-1 Galaxy tutorial: demo and exercise 1. 5om&lete t%e galaxy 101 tutorial* 2. Share the @nal #or-Po#* :. Briefly describe the #or-Pow in your o#n #ords* 3. =e-use t%e #or-flo#, but t%is time; c%oose Bll SCPs dataset as feature* Galaxy Demo & Exercise EMBOSS 6%e European Molecular Biology O&en Soft#are Suite Large user community Bvailable on the web, for many OS, servers and stand-alone If you know ho# to use one( t%en you kno# %o# to use all Mature and stable Sequence Analysis Introduction EMBOSS ?%at is it good forN Sequence alignment Database search with sequence patterns Motif identification and domain analysis Cucleotide sequence pattern analysis Sequence Analysis Introduction EMBOSS FROM SOURCEFORGE %ttp:/,emboss*sourceforge.net/ EMBOSS programs within galaxy Many other portals http://www.ebi.ac.uk/Tools/emboss/ http://emboss.bioinformatics.nl/ http://imed.med.ucm.es/EMBOSS/ http://www.bioinformatics2.wsu.edu/emboss/ http://pro.genomics.purdue.edu/emboss/ Quick CpG islands background for next exercise =egion of high density CG dinucleotides along t%e DNB 200 – 500 nucleotides( enric%ed #ith 5G Enriched C&G nucleotides The & in 5&G islands represent t%e phos&hodiester bond bet#een the C and G nucleotides Mostly occur #ithin the promoter of eukaryotic genes Loc- gene in an inactive state 0elps identify the transcri&tion start site of a gene Exercise From galaxy, emboss toolshed: List all tools that analyze CpG islands On the search field, type in “cpg” Access the documentation for two of these tools, Preferably cpgplot and newcpgreport Exercise • Write down t%e e &ected result • =un the tools on t%e %uman gene !ABC! • Inter&ret t%e results* Software for sequence analysis • "&en source tools • 5ommercial tools Software for sequence analysis • Websites with lin-s to o&en source tools and ser)ices http://www.ebi.ac.uk/services http://www.ncbi.nlm.nih.gov/guide/sequence-analysis/ http://bioinformatics.ca/links_directory/ http://bioinformatics.ca/links_directory/category/dna/structure-and-sequence- feature-detection Software for sequence analysis "&en source GC1 general public licenses (GC1 GPA< • 5ontinuous evolution of code • 5ommunity su&&orted
Details
-
File Typepdf
-
Upload Time-
-
Content LanguagesEnglish
-
Upload UserAnonymous/Not logged-in
-
File Pages69 Page
-
File Size-