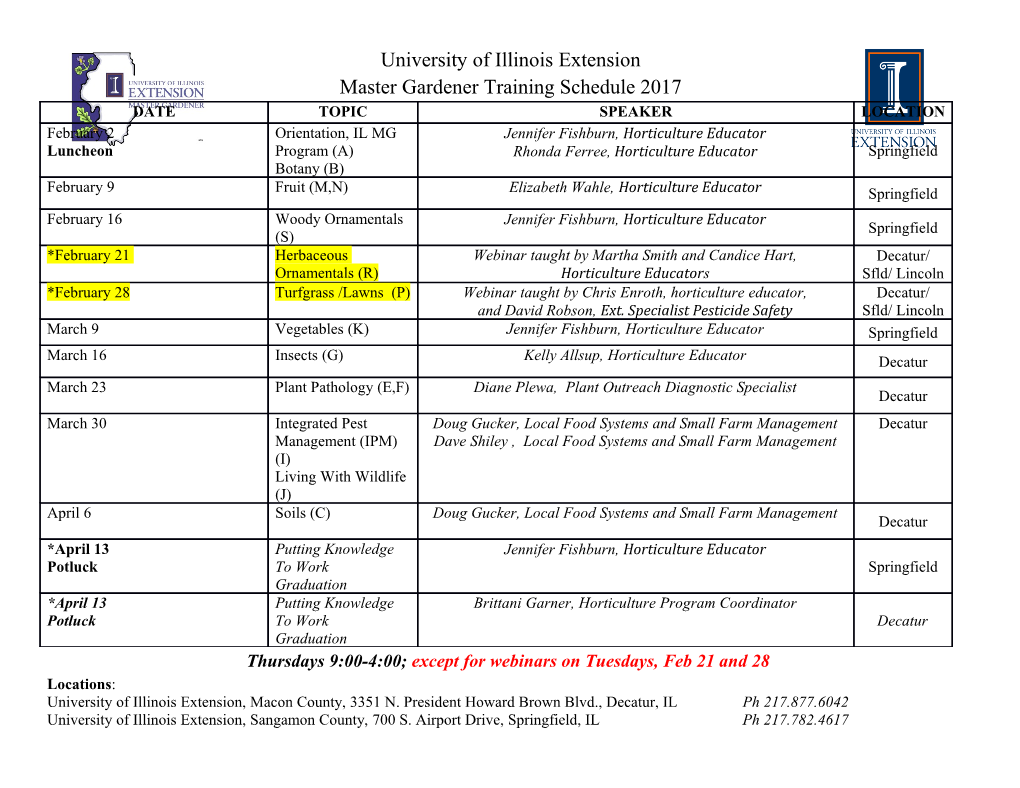
Journal of Spatial Hydrology Volume 13 Number 1 Article 2 2017 Determination of curve number and estimation of runoff using Indian experimental rainfall and runoff data Follow this and additional works at: https://scholarsarchive.byu.edu/josh BYU ScholarsArchive Citation (2017) "Determination of curve number and estimation of runoff using Indian experimental rainfall and runoff data," Journal of Spatial Hydrology: Vol. 13 : No. 1 , Article 2. Available at: https://scholarsarchive.byu.edu/josh/vol13/iss1/2 This Article is brought to you for free and open access by the Journals at BYU ScholarsArchive. It has been accepted for inclusion in Journal of Spatial Hydrology by an authorized editor of BYU ScholarsArchive. For more information, please contact [email protected], [email protected]. Journal of Spatial Hydrology Vol.13, No.1 Fall 2017 Determination of curve number and estimation of runoff using Indian experimental rainfall and runoff data Pushpendra Singh*, National Institute of Hydrology, Roorkee, Uttarakhand, India; email: [email protected] S. K. Mishra, Dept. of Water Resources Development & Management, IIT Roorkee, Uttarakhand; email: [email protected] *Corresponding author Abstract The Curve Number (CN) method has been widely used to estimate runoff from rainfall runoff events. In this study, experimental plots in Roorkee, India have been used to measure natural rainfall-driven rates of runoff under the main crops found in the region and derive associated CN values from the measured data using five different statistical methods. CNs obtained from the standard United States Department of Agriculture - Natural Resources Conservation Service (USDA-NRCS) table were suitable to estimate runoff for bare soil, soybeans and sugarcane. However, we propose a new method to estimate CN values from measured rainfall-runoff data (CN calibrated) from fallow lands, finger millet and maize. The new CN values provide better runoff estimates than the values from the standard USDA-NRCS table. The results of this study can be used in hydrologic models to evaluate impacts of land use and land cover (LULC) changes on water quality and water quantity over the years in the region. Keywords Curve number, runoff, hydrologic models, land use and land cover, water quality, water quantity Journal of Spatial Hydrology Vol.13, No.1 Fall 2017 Introduction Models have been developed to evaluate changes in hydrological processes. The Curve Number (CN) method developed in 1954 by the USDA Soil Conservation Service (SCS), currently the USDA-NRCS, is one of the methods most often used to estimate direct surface runoff from a given rainfall event (Hawkins et al. 2009). Because of the simplicity, versatility, and availability of necessary data, this method has been quite popular within the United States and other countries (Ponce and Hawkins 1996; Sartori et al. 2011; Hawkins et al. 2009). Several hydrologic, soil erosion, and water quality models have used the CN method, such as Chemicals, Runoff and Erosion from Agricultural Management Systems (CREAMS) (Knisel 1980), Simulator for Water Resources in Rural Basins (SWRRB) (Williams et al. 1985), Agricultural Nonpoint Source pollution model (AGNPS) (Young et al. 1989), Erosion Productivity Impact Calculator (EPIC) (Sharpley and Williams 1990), Soil and Water Assessment Tool (SWAT) (Arnold et al. 1998), CN-based modeling of sediment yield (Mishra et al. 2006; Tyagi et al. 2008), and the CN method coupled with the Revised Universal Soil Loss Equation (RUSLE) model (Gao et al. 2012). CN estimations by in situ data from plots or watersheds are preferable. Errors in the tabulated CN can result in large errors in surface runoff estimation (Hawkins et al. 2009; Soulis et al. 2009). Many authors have reported better runoff estimates from the in- situ data than from using tabulated CN (Elhakeem and Papanicolaou 2009; Shi et al. 2009; Tedela et al. 2012; D’Asaro et al. 2014; Ajmal and Kim 2014; Lal et al. 2015). Tabulated CN derived for rainfall-runoff data were originally computed from a graphical method, whereas annual runoff and rainfall volumes were plotted to obtain the curve that divides the plotted points into two equal groups, thus corresponding the median CN. In this study, only one peak flow events for each year is considered (USDA NRCS 2004). Other methods for calculating the CN from rainfall-runoff data include the geometric mean (USDA NRCS 2004); arithmetic mean (Bonta 1997; Tedela et al. 2012); nonlinear, least squares fit (Hawkins 1993); standard asymptotic fit (Hawkins 1993); and lognormal frequency (Schneider and McCuen 2005). However, a consensus is lacking for the best method that might be used as a standard for CN estimation. Most investigations use Journal of Spatial Hydrology Vol.13, No.1 Fall 2017 several methods to estimate the CN and then choose the best method for each condition (Tedela et al. 2012). In addition, there are several sources of uncertainty in the use of the CN method for estimating surface runoff from regions with undisturbed cover (Tedela et al. 2012). Thus, the objectives of this study were to measure natural rainfall-driven rates of runoff under undisturbed vegetation and under the main crops found in this region, and to derive associated CN values from the five more frequently used statistical methods. We also evaluated the use of the CN method to estimate runoff in this region and suggested CN values and ranges for the land covers studied. Materials and Methods The experimental catchments are in Village Todakalyanpur (Latitude: 290 50’ 9” N, Longitude: 770 55’ 21” E) in Roorkee, District of Haridwar, Uttarakhand State, India (Figure 1). The experimental watersheds have been developed by the Department of Water Resources Development and Management, IIT Roorkee to study the impacts of land use/landcover, soil type and watersheds slope on the runoff generation process. In this study, three different types of land use such as Maize, Finger Millet and Fallow have been considered. Further each type of land use is having three different slopes (8%, 12% and 16%). Thus, total nine plots are there having V-notches for measuring runoff for each rainfall event. The experimental watershed is equipped with recording and non-recording rain-gauges for measuring rainfall events. The average Reduced Level of the site is 266 m. Average precipitation of the study site varies from 1200 mm to 1500 mm mostly (70- 80%) occurring during the months of June to September. Journal of Spatial Hydrology Vol.13, No.1 Fall 2017 Figure 1: Location map of the Study Area The methodologies used in this study includes collection of rainfall-runoff data and its processing, mathematical formulations for the CN estimation with varying watershed slopes as well as initial abstraction ratio (λ). The generated CNs are being used henceforth for runoff estimation using NRCS-CN model. During the study period starting from May to November, data for 26 natural P-Q storm events have been carefully collected from nine experimental plots for evaluating the performance of the proposed NRCS-CN mathematical models considering watershed slope and initial abstraction in comparison to the existing slope based NRCS-CN models. Journal of Spatial Hydrology Vol.13, No.1 Fall 2017 Worth mentioning here, there are few models available in hydrologic literature dealing with watershed slope based on CN concept for runoff estimation. Estimation of Curve Number from Rainfall-Runoff Data The CN method is based on the following water budget equation to estimate the storm runoff: Q = P – Ia – F, (1) where, Q is total runoff (mm); P is total rainfall (mm) (P>Q and P>Ia); Ia is initial abstraction (mm); and F is the amount of surface retention (mm). Runoff depth (Q) is estimated in the original SCS-CN model for rainfall depth (P) and the initial abstraction (Ia) as 2 (P − I a ) Q = P ≥ Ia; else Q= 0 (2) (P − I a + S) I a = λS (3) S = 2λP + Q(1− λ)− {[Q(1− λ)]2 + 4λQP} /(2λ2 ) (4) 25400 CN = 0 ≤ CN ≤ 100 (5) obs 254 + S where, λ= 0.05 (Woodward et al (2003) or 0.1 or 0.2 (Hawkins 1993) or 0.3 (NEH) and S = Maximum Potential Retention (mm). The CNobs is a dimensionless index ranging from 0 (no runoff, S = ∞) to 100 (all rainfall becomes runoff, S = 0). For the available rainfall and runoff events, the values of ‘S’ can be obtained from equation (4) and the value is then transformed to the CN scale using equation (5). These numbers were used to obtain the median and mean for each individual plot. For the geometric mean, we first calculated the logarithm of the event maximum potential retention S derived using equation (4), determined the arithmetic mean of the series for each experimental plot, log S; and then calculated the geometric Journal of Spatial Hydrology Vol.13, No.1 Fall 2017 mean of maximum potential retention,10logS (Tedela et al. 2012). Thus, the CN was computed as the following: = (6) 100 10 1+ 254 The nonlinear, least squares fit method was used by minimizing the sum of squared differences between observed and calculated CN runoff using equation (2) for each rainfall-runoff event for a given experimental plot. For this method, we used only large storms (P> 25.4 mm [1 in]) to avoid bias towards larger CN found with small rainfall events (Hawkins et al. 2009). For the standard asymptotic fit method, we first rank-ordered both the rainfall and runoff time series separately, matching them in pairs from a decreasing order, and then computing the CN values from equations 7 and 8 using the rank-matched pairs (Hawkins 1993). The CN values were evaluated according to three types of behavior identified by Hawkins (1993): standard, complacent, and violent. The standard behavior occurs when the CN values decrease with the total rainfall and tend to approach a near-constant CN (called CNb) with rainfall increase.
Details
-
File Typepdf
-
Upload Time-
-
Content LanguagesEnglish
-
Upload UserAnonymous/Not logged-in
-
File Pages26 Page
-
File Size-