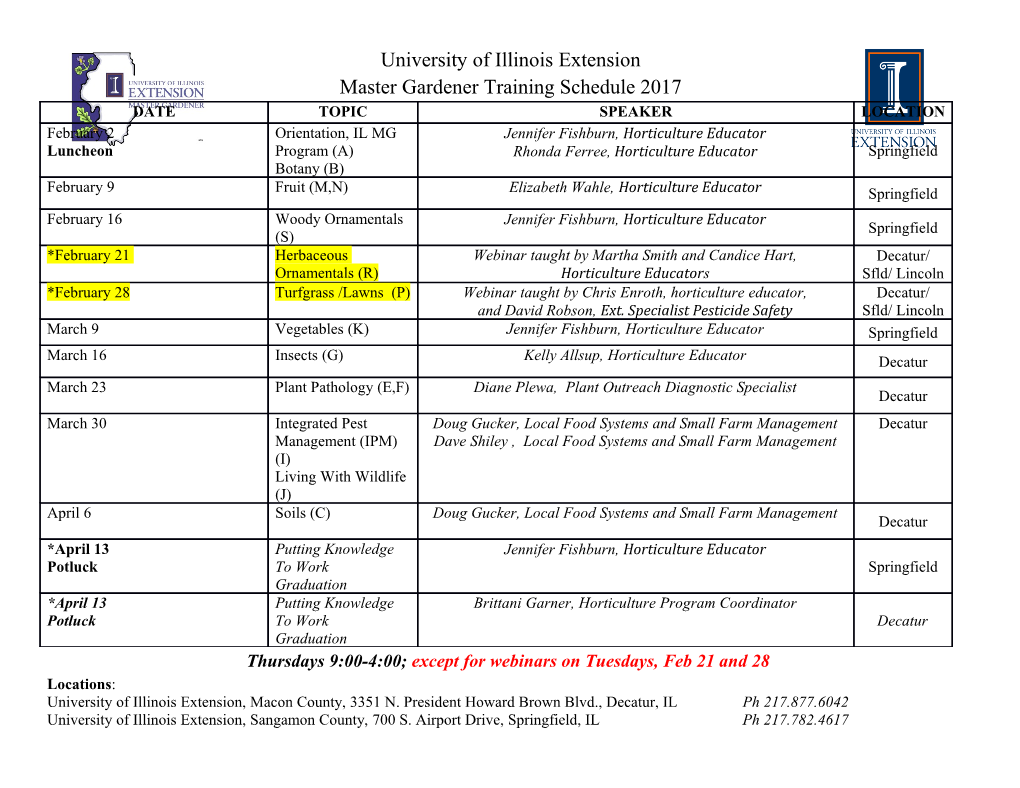
Essays in Revealed Preference Theory and Behavioral Economics Thesis by Taisuke Imai In Partial Fulfillment of the Requirements for the Degree of Doctor of Philosophy California Institute of Technology Pasadena, California 2016 (Defended December 11, 2015) ii c 2016 Taisuke Imai All Rights Reserved iii To my family. iv Acknowledgements First and foremost, I would like to express my sincere thanks to my advisor, Colin Camerer, for his support, patience, and encouragement. I first met him in the hot summer of 2007 at the University of Tokyo. He gave a talk on then-emerging field of neuroeconomics. The day after his seminar, I had an opportunity to show him around several sightseeing places in Tokyo. During this tour, he enthusiastically talked about the history, challenges, and future directions of behavioral, experi- mental, and neuro-economics. I was astonished by his encyclopedic knowledge and was deeply inspired by his passion for pioneering new fields of researches. That was the moment I decided to come to Caltech to conduct researches in those fields. My research interest drifted during the course, but he always supported me and gave the best guidance at every stage of my doctoral work. I benefited greatly from Federico Echenique and Kota Saito. Keeping up with their speed of discussion was sometimes challenging to me, but at the same time, working with them has always been enjoyable. Conversations with Ming Hsu, Pietro Ortoleva, Antonio Rangel, and Matthew Shum have also been immensely helpful. I would also like to thank my friends who gave me constructive feed- backs and thoughtful questions: Ryo Adachi, Rahul Bhui, Ben Bushong, Kyle Carlson, Matthew Chao, John Clithero, Cary Frydman, Keise Izuma, Andrea Kanady Bui, Yutaka Kayaba, Jackie Kimble, Ian Krajbich, Gidi Nave, Euncheol Shin, Alec Smith, Shinsuke Suzuki, Federico Tadei, and Romann Weber. Laurel Auchampaugh and Barbara Estrada have provided me with amazing administra- tive support. I would also like to acknowledge the Nakajima Foundation for their financial support throughout my Ph.D. research. Last but not least, I thank my wife Noriko who always believed in me. Without her love and support, I could not have achieved this long academic journey. v Abstract Time, risk, and attention are all integral to economic decision making. The aim of this work is to understand those key components of decision making using a variety of approaches: providing axiomatic characterizations to investigate time discounting, generating measures of visual attention to infer consumers’ inten- tions, and examining data from unique field settings. Chapter2, co-authored with Federico Echenique and Kota Saito, presents the first revealed-preference characterizations of exponentially-discounted util- ity model and its generalizations. My characterizations provide non-parametric revealed-preference tests. I apply the tests to data from a recent experiment, and find that the axiomatization delivers new insights on a dataset that had been an- alyzed by traditional parametric methods. Chapter3, co-authored with Min Jeong Kang and Colin Camerer, investigates whether “pre-choice” measures of visual attention improve in prediction of con- sumers’ purchase intentions. We measure participants’ visual attention using eye- tracking or mousetracking while they make hypothetical as well as real purchase decisions. I find that different patterns of visual attention are associated with hypothetical and real decisions. I then demonstrate that including information on visual attention improves prediction of purchase decisions when attention is measured with mousetracking. Chapter4 investigates individuals’ attitudes towards risk in a high-stakes en- vironment using data from a TV game show, Jeopardy!. I first quantify players’ subjective beliefs about answering questions correctly. Using those beliefs in esti- mation, I find that the representative player is risk averse. I then find that trailing players tend to wager more than “folk” strategies that are known among the com- munity of contestants and fans, and this tendency is related to their confidence. I also find gender differences: male players take more risk than female players, and even more so when they are competing against two other male players. Chapter5, co-authored with Colin Camerer, investigates the dynamics of the favorite-longshot bias (FLB) using data on horse race betting from an online ex- vi change that allows bettors to trade “in-play.” I find that probabilistic forecasts implied by market prices before start of the races are well-calibrated, but the de- gree of FLB increases significantly as the events approach toward the end. vii Contents Acknowledgements iv Abstractv 1 Introduction1 2 Testable Implications of Models of Intertemporal Choice: Exponential Discounting and Its Generalizations3 2.1 Introduction.................................3 2.2 Exponentially Discounted Utility.....................7 2.3 A Characterization of EDU Rational Data................8 2.3.1 No Discounting and One Observation: d = 1 and K = 1...9 2.3.2 No Discounting: d = 1....................... 10 2.3.3 General K and d ........................... 11 2.4 More General Models........................... 12 2.4.1 Quasi-Hyperbolic Discounted Utility.............. 12 2.4.2 More General Models of Time Discounting........... 15 2.5 Empirical Application........................... 17 2.5.1 Description of the Data...................... 17 2.5.2 Results................................ 19 2.6 Proof of Theorem1............................. 29 2.6.1 Necessity.............................. 31 2.6.2 Theorem of the Alternative.................... 31 2.6.3 Sufficiency.............................. 32 2.6.4 Proof of Lemma5......................... 33 2.6.5 Proof of Lemma6......................... 39 2.6.6 Proof of Lemma7......................... 40 2.7 Proof of Theorem2............................. 41 2.7.1 Necessity.............................. 41 viii 2.7.2 Sufficiency.............................. 42 2.7.3 Proof of Lemma 10......................... 43 3 When the Eyes Say Buy: Visual Fixations during Hypothetical Consumer Choice Improve Prediction of Actual Purchases 47 3.1 Introduction................................. 47 3.2 Experimental Design............................ 51 3.2.1 Experiment I: Mousetracking Study............... 51 3.2.2 Experiment II: Eyetracking Study................ 55 3.3 Results.................................... 56 3.4 Discussion.................................. 66 4 Risk Taking in a Natural High-Stakes Setting: Wagering Decisions in Jeopardy! 71 4.1 Introduction................................. 71 4.2 Description of Jeopardy! and Data..................... 74 4.2.1 Rules of the Game......................... 74 4.2.2 Source and Description of the Dataset.............. 75 4.3 Preliminary Analyses............................ 76 4.3.1 Setup and Notations........................ 76 4.3.2 Descriptive Statistics........................ 76 4.4 Metrick(1995) Revisited.......................... 78 4.5 Estimating the Representative Player’s Risk Preferences....... 82 4.5.1 Quantifying Subjective Beliefs.................. 82 4.5.2 Estimation.............................. 85 4.6 Folk Wagering Strategies in Non-Runaway Games........... 87 4.7 Gender Differences............................. 90 4.8 Conclusion.................................. 95 5 Dynamics of Forecast Miscalibration in the U.K. Horse Racing In-Play Betting Data 97 5.1 Introduction................................. 97 5.2 Data...................................... 99 5.2.1 Background on Betting...................... 99 5.2.2 Description of Data......................... 101 5.2.3 Descriptive Statistics........................ 102 5.3 Empirical Tests of the Favorite-Longshot Bias.............. 105 5.3.1 Bunching Horses with Similar Odds............... 105 ix 5.3.2 Linear Regression.......................... 107 5.3.3 Nonparametric Estimation of Calibration Curves....... 107 5.3.4 Parametric Estimation of Calibration Curves.......... 111 5.4 Discussion.................................. 116 5.4.1 Magnitude of Estimated Curvature............... 116 5.4.2 Explanations for the Favorite-Longshot Bias.......... 118 5.5 Conclusion.................................. 122 A Appendix to Chapter 2 124 A.1 Proof of Theorem3............................. 124 A.1.1 MTD................................. 124 A.1.2 TSU.................................. 126 A.1.3 Proof of Lemma 14......................... 127 A.2 Implementing Revealed Preference Tests................ 129 A.3 Ground Truth Analysis: Test Performance and Parameter Recovery. 133 A.4 Additional Results from Empirical Application............. 138 A.4.1 Estimated Daily Discount Factors................ 140 A.4.2 Parameter Recovery........................ 141 A.5 Implementing Minimum Price Perturbation Test............ 144 A.6 Distance Measure Based on Maximal Subset: A Robustness Check. 146 A.7 Jittering: Perturbing Choices....................... 147 B Appendix to Chapter 3 151 B.1 Gaze Data Processing............................ 151 B.2 Additional Results............................. 152 B.2.1 Monotonicity of Preferences................... 152 B.2.2 Estimating the Size of Hypothetical Bias............ 153 B.3 Supplementary Figures and Tables.................... 153 B.4 Instructions................................. 165 B.4.1 Experiment I: Mousetracking................... 165 B.4.2 Experiment II: Eyetracking.................... 173 C Appendix to Chapter 4 180 C.1 Behavioral Responses
Details
-
File Typepdf
-
Upload Time-
-
Content LanguagesEnglish
-
Upload UserAnonymous/Not logged-in
-
File Pages230 Page
-
File Size-