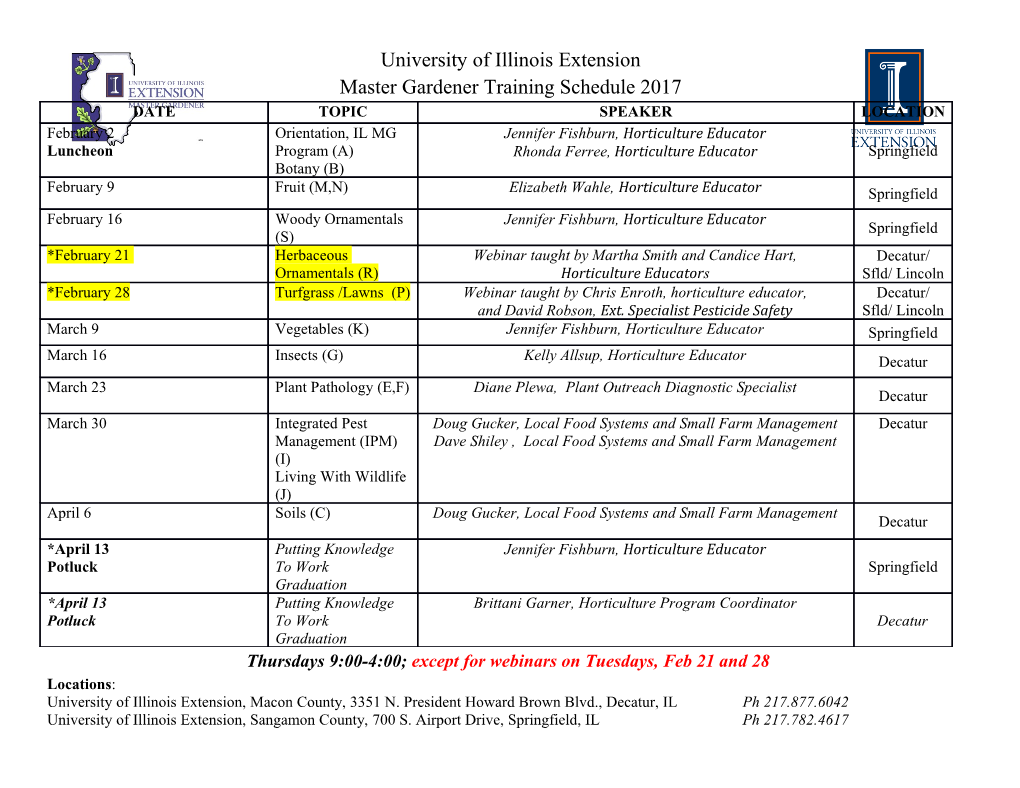
Pros and Cons of ABMs for Epidemic Modeling Virtual Workshop June 21-22, 2021 SPEAKER TITLES/ABSTRACTS David Banks Duke University/SAMSI “Inference Without Likelihood” In most ABM applications, one cannot write out the likelihood function. In those cases, the only two recourses that statisticians have are emulators and approximate Bayesian computation. This talk describes the strengths and weaknesses fo those methods in the context of epidemiologic modeling. Paul Birrell Public Health England “Real-time Challenges in Modelling a Real-life Pandemic” In England policy decisions during the SARS-COV-2 pandemic have relied on prompt scientific evidence on the state of the pandemic. Since March 2020, through weekly contributions to governmental advisory groups, the "PHE/Cambridge" real-time model has contributed to real time pandemic monitoring and assessment, from an early exponential phase, to the current embryonic third wave. Mostly, these contributions have been in the form of estimation of key epidemic quantities and short-to-medium-term projections of severe disease. In this talk I describe the statistical transmission modelling framework we have used throughout and its continual adaptation throughout the pandemic to tackle the introduction of vaccinations, the ecological effects of new variants, the changing relationship between available data streams and many other challenges posed by the ever-evolving epidemiology. As datasets get longer and models become more complex, more attention must be paid to the computational aspects of fitting such a model to ensure that model developments do not jeopardise the timely provision of relevant, converged, outputs to policymakers. Georgiy Bobashev RTI “Hybrid System Dynamics and Agent-Based Models” Predictive models range in levels of complexity, applicability, and the tasks they can perform. Agent-based models are most complex, but also most computationally intensive and require extensive parameterization. Simpler models can often do a similar job with less resources. I will present examples of epidemic models that could be considered as hybrid, i.e. start as ABMs, but eventually becoming simpler system dynamic models. Another type of hybrid models is models where one component is system dynamics and another is ABM. I will discuss model comparisons to evaluate similarity in performances of complex and simplified models. Formal hypothesis testing could be used in model evaluation but requires specific considerations of minimal effects. I will provide examples of epidemic models from flu, covid and substance use. Sara Del Valle LANL “Modeling COVID-19 with Agent-based Simulations: Challenges & Opportunities” In this talk, I will describe how agent-based simulations were critical in understanding and assessing the impact of different pharmaceutical and non-pharmaceutical interventions on COVID- 19 spread due to their ability to capture complex demographics and population characteristics. I will also describe some of the challenges and opportunities in parametrizing large-scale agent-based simulations. M. Elizabeth Halloran Fred Hutchinson Cancer Center “Simulations for Designing and Interpreting Vaccine Studies” Interventions in infectious diseases can have both direct effects on individuals who receive the intervention as well as indirect effects in the population. In addition, intervention combinations can have complex interactions at the population level, which are often difficult to adequately assess with standard study designs and analytical methods. Here we discuss a paradigm for the design and interpretation of vaccine trials in infectious diseases, particularly with regard to emerging infectious diseases, using simulations that reflects the dynamics of the transmission process. In an increasingly complex world, simulations can explicitly represent transmission dynamics, which are critical for proper trial design and interpretation Phyllis Ju Harvard University “Scalable Inference in Agent-based Epidemic Models using Sequential Monte Carlo” Agent-based models have been used to characterize the complex dynamics of epidemics because they describe how individuals interact in a network and with the environment. Despite many attractive features of agent-based models, parameter estimation remains a challenge due to intractable computations. In this talk, we present an agent-based susceptible-infectious-removed hidden Markov model that allows heterogeneity among individuals, a social network structure, and observation noises. Next, we develop sequential Monte Carlo methods that enable scalable and efficient statistical inference in agent-based models. The guiding principle is to design informed proposals that take the observations and model structure into account by leveraging knowledge from signal processing and optimal control. Finally, our methodology is illustrated on the celebrated Abakaliki smallpox dataset. Chris Krapu ORNL “Making the Most of Recent Advances in Statistical Programming for ABM Emulation” Gaussian process models are finding widespread usage in statistical software packages such as Stan and PyMC3, leading to a wealth of advances in open-source tools for flexible and scalable nonparametric modeling. In this work, we review some of the most useful of these features in PyMC3 and employ a spatial susceptible-infected-removed agent-based model to show how the construction of ABM emulators can benefit from their usage. Jessica Ginepro Notestine NCSU “Sensitivity and Active Subspace Analysis for Agent-Based Models” Agent-based models can have several parameters, ranging up to 15 or more. For many problems, sensitivity or active subspace analysis is critical to determine subsets or subspaces of parameters, which strongly inuence model responses and hence can be inferred from data. We discuss current sensitivity analysis methods for agent-based models and propose active subspace techniques for agent-based models. We first discuss one-at-a-time sensitivity analysis, correlation coefficients, and Sobol' sensitivity analysis. We then demonstrate the use of active subspaces to compute activity scores as an alternative to current sensitivity analysis methods. We implement this for a small-scale agent-based model and compare results of active subspace analysis to those of current sensitivity analysis methods for agent-based models. We conclude by showing that we obtain similar results with all analysis methods. Further, computing activity scores will require significantly less model evaluations than Sobol' sensitivity analysis. Bruce Rogers Formerly Duke University “How Much Testing is Enough?” The classic epidemic containment strategy of surveillance and quarantine can be highly effective. Using a network based SEIR ABM, we consider the policy question, "How much surveillance testing is necessary to quell an epidemic outbreak?" That is, agent based models are used to study surveillance testing from a control theory perspective. This particular ABM is adapted from Paul Romer, who was advocating 25 million COVID tests per day in the United States during the spring of 2020. Here, we focus on more modestly sized institutions, say 5000 to 10000 individuals, with an interest in parameter calibration and outbreak detection. Toryn Schafer Cornell University “Inverse Reinforcement Learning for Agent Based Models” Agent-based methods allow for defining simple rules that generate complex group behaviors. The governing rules of such models are typically set a priori and parameters are learned from observed behavior trajectories. Instead of making simplifying assumptions across all anticipated scenarios, inverse reinforcement learning provides inference on the short-term (local) rules governing long term behavior policies by using properties of a Markov decision process. In large state spaces, computational feasibility is achieved by the use of basis functions and the linearly solvable Markov decision process. Simon Spencer University of Warwick “Scalable Bayesian Inference and Model Selection for Individual-based Epidemic Models” People (and chickens) are not all the same! Whilst it is quite straight-forward to design individual heterogeneities and covariate information into simulation models for infectious disease transmission, it is definitely not as easy to perform parameter inference for the resulting models, especially if the data available are limited. Such models often quickly become over-parameterised and at the same time remain limited in the way in which heterogeneity is captured, for example assuming that individuals in the same age group all behave the same. To solve these issues we need to fit epidemic models to individual level data, and to perform model selection to identify the heterogeneities that are necessary to explain the observed data. In this work, I will describe how we can view an individual-based epidemic model as a coupled hidden Markov model and fit it to individual level data using an efficient and computationally scalable Markov chain Monte Carlo algorithm. Furthermore, I will describe how to utilize this algorithm to obtain accurate estimates of the model evidence – the key statistic needed to compare models within a Bayesian framework. I will illustrate these novel methods on an application to highly pathogenic avian influenza in chickens. Our approach allows us to identify the specific mechanisms by which genetically modified chickens can prevent the spread of infection and, for the first time, we were able to perform exact Bayesian model averaging for
Details
-
File Typepdf
-
Upload Time-
-
Content LanguagesEnglish
-
Upload UserAnonymous/Not logged-in
-
File Pages5 Page
-
File Size-