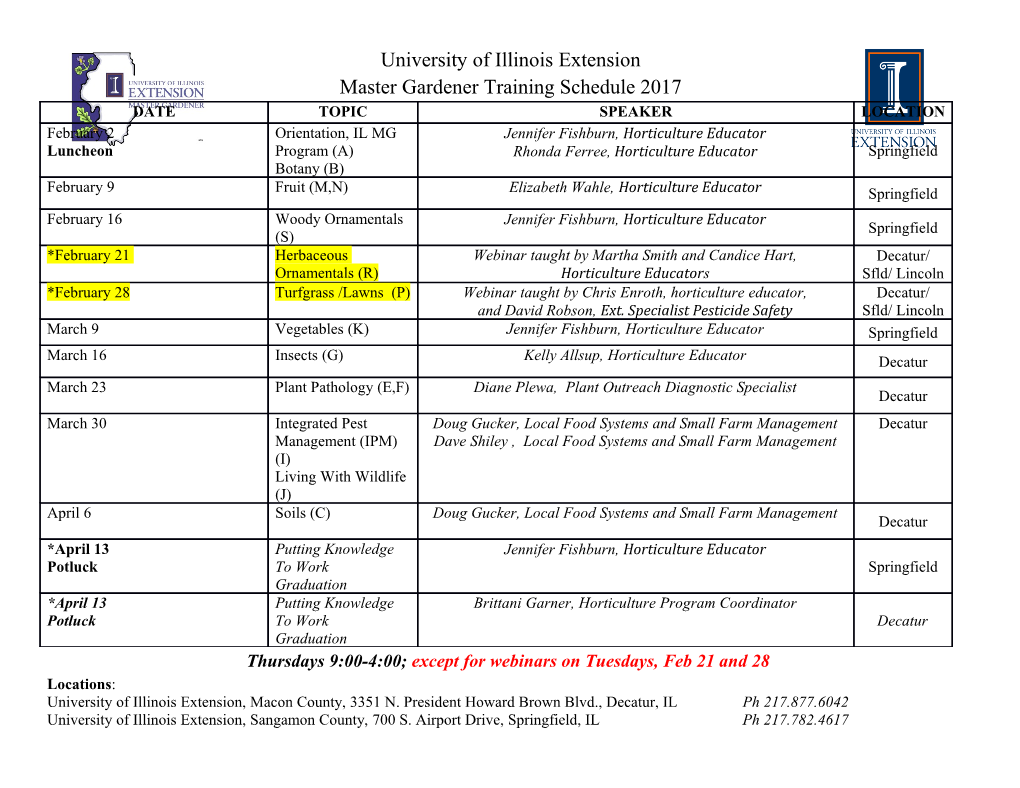
Stochastic Dependence in Wireless Channel Capacity: A Hidden Resource Fengyou Sun and Yuming Jiang NTNU, Norwegian University of Science and Technology, Trondheim, Norway [email protected], [email protected] Abstract—This paper dedicates to exploring and exploiting way to achieve dependence control. We classify the de- the hidden resource in wireless channel. We discover that pendence into three categories, i.e., positive dependence, the stochastic dependence in wireless channel capacity is a independence, and negative dependence, and we identify hidden resource, specifically, if the wireless channel capacity bears negative dependence, the wireless channel attains a better the dependence as a resource, because when the wireless performance with a smaller capacity. We find that the dependence channel capacity bears negative dependence relative to in wireless channel is determined by both uncontrollable and positive dependence, the wireless channel attains a better controllable parameters in the wireless system, by inducing performance with a smaller average capacity with respect negative dependence through the controllable parameters, we to independence. Being exploitable is a further require- achieve dependence control. We model the wireless channel capacity as a Markov additive process, i.e., an additive process ment of a benign resource, specifically, the dependence defined on a Markov process, and we use copula to represent in wireless channel is induced both by the uncontrol- the dependence structure of the underlying Markov process. lable parameters, e.g., fading, and by the controllable Based on a priori information of the temporal dependence of the parameters, e.g., power, we model the dependence caused uncontrollable parameters and the spatial dependence between by these random parameters with multivariate copula, the uncontrollable and controllable parameters, we construct a sequence of temporal copulas of the Markov process, given the and we propose to use the controllable dimensions to initial distributions, we obtain a sequence of transition matrices transform the dependence in whole capacity process. of the controllable parameters satisfying the expected dependence While the multivariate dependence nature of wireless properties of the wireless channel capacity. The goal of this paper channel capacity is complex, the diversity of dependence is to show the improvement of wireless channel performance from structures in different dimensions provides an opportu- transforming the dependence structures of the capacity. Index Terms—Wireless channel capacity; Dependence model; nity to achieve dependence control and improve channel Dependence control; Markov process; Copula. performance. I. INTRODUCTION The copula property of Markov process is investigated in [5] Wireless communication has been around for over a hundred and extended to high order case in [6] and multivariate case years, starting in 1896 with Marconi’s successful demon- in [7]. No-Granger causality is a concept in econometrics and stration of wireless telegraphy and transmission of the first its relation with Markov process is investigated in [8]. We wireless signals across the Atlantic in 1901 [1]. For cellular model the random parameters in wireless channel capacity as systems, the first generation is deployed in around 1980s, i.e., a multivariate Markov process, the Markov family copula in 1G, then 2G in 1990s, 3G in 2000s, and 4G in 2010s [1]. [5], [7] are used not only as a mechanism for dependence It has become a pattern that a new generation of wireless modelling, i.e., the copula is an expression of dependence system is deployed every a decade and the theme of each structure, but also as a tool for dependence controlling, i.e., arXiv:1711.10363v2 [cs.IT] 11 Nov 2019 generation is to increase the capacity and spectral efficiency the copula function provides a solution to the controllable of wireless channels. The trend is driven by the explosion of parameter configuration. We apply the no-Granger causality wireless traffic that is a rough reflection of people’s demand to model the relationship between the controllable and uncon- on wireless communication, and the paradox of supply and trollable parameters, and the sufficient and necessary condition demand [2] is kept relieving generation by generation through for Markov process is extended from the bivariate case in [8] exploiting the physical resources, i.e., power, diversity, and to the multivariate case in this paper. degree of freedom [3]. The remainder of this paper is structured as follows. Sec. Considering trillions of devices to be connected to the II dedicates to modelling dependence in wireless channel wireless network, high capacity demand, and stringent latency capacity. First, basic capacity concepts are introduced, includ- requirement in the coming 5G [4], it’s imperative to rethink ing instantaneous capacity, cumulative capacity, and transient the wireless channel resources. We present a perspective on the capacity; second, the capacity is modeled as a Markov additive challenge by asking and answering a question in this paper. process and the Markov property is expressed by copula; third, • What’s the hidden resource and how to use it? the distribution function of the Markov additive capacity is We discover that the stochastic dependence in wireless investigated and lower and upper bounds are derived. Sec. channel capacity is the hidden resource and we find a III proposes an approach to control dependence. First, perfor- mance measures of the wireless channel are derived, namely B. Markov Dependence delay, backlog, and delay-constrained capacity; second, the Let (Ω, F , (F t)t∈N , P ) be a filtered probability space and dependence is classified into three types, i.e., positive de- (Xt)t∈N be an adapted stochastic process. X is a Markov pendence, independence, and negative dependence, according process if and only if to the performance measure, it’s proved that the negative dependence is good to the channel performance, while the P (Xt ≤ x|Xt−1, Xt−2,..., X0)= P (Xt ≤ x|Xt−1) . (7) positive dependence does the opposite thing; last, the cause The Markov property is solely a dependence property that can of dependence in capacity is distinguished as uncontrollable be modeled exclusively in terms of copulas [5], [7]. (Copula parameters and controllable parameters, it’s elaborated how to is introduced in Appendix A.) use the controllable parameters to induce negative dependence The n-dimensional process X is a Markov process, if and to the capacity, and an example of using power as a control- only if, for all t < t <...<t , the copula C of lable parameter is illustrated. Finally, the paper is concluded 1 2 p t1,...,tp (X ,..., X ) satisfies [7] in Sec. IV. t1 tp Ct (.) Ct2 (.) Ct3 (.) p−1 EPENDENCE ODEL II. D M Ct1,...,tp = Ct1,t2 ⋆ Ct2,t3 ⋆ . ⋆ Ctp−1,tp . (8) A. Channel Capacity Provided that the integral exists for all x, y, z, the operator Consider a flat fading channel with input x(t), output y(t), C(.) ⋆ is defined by fading process h(t), and additive white Gaussian noise process z C(z) n(t) ∼ CN (0,N0), the complex baseband representation is (A ⋆ B)(x, y)= A,C(x, r) · BC,(r, y)C(dr), (9) expressed as [3], [9] Z0 y(t)= h(t)x(t)+ n(t), (1) where A is a (k + n)-dimensional copula, B is a (n + l)-dimensional copula, C is a n-dimensional cop- conditional on a realization of h(t), the mutual information is ula, and A(x, dy) = A,C (x, y)C(dy) and B(dx, y) = expressed as [9] BC,(x, y)C(dx) are respectively the derivative of the copula P (x, y|ht) A(x,.) and B(., y) with respect to the copula C. A,C and I(X; Y |h(t)) = P (x, y|ht) log2 , (2) P (x|ht)P (y|ht) BC, are well-defined. Specifically, for 1-dimensional Markov x∈XX,y∈Y process, the copula is expressed by [5] where X and Y are respectively the input and output alphabets C = C ⋆ C ⋆...⋆C , (10) of the channel. For multiple input and multiple output channel, t1...tn t1t2 t2t3 tn−1tn the generalized formula is available in [10], [11]. where Ct1...tn is the copula of (Xt1 ,...,Xtn ), Ctk−1tk is the The maximum mutual information over input distribution at copula of Xtk−1 ,Xtk , and A⋆B is defined as t, denoted as C(t), is defined as instantaneous capacity [12]: A⋆B (x ,...,x )= C(t) = max I(X; Y |h(t)). (3) 1 m+n−1 P (x) xm ∂A (x ,...,x , ξ) ∂B (ξ, x ,...,x ) ,m 1 m−1 1, m+1 m+n−1 dξ, The sum of instantaneous capacity in discrete time (s,t] or Z ∂ξ ∂ξ the integral of instantaneous capacity in continuous time [s,t), 0 denoted as S(s,t), is defined as cumulative capacity: (11) t t for m-dimensional copula A and n-dimensional copula B. S(s,t)= C(i) or S(s,t)= C(τ)dτ . (4) Z Proposition 1. If the dependence in capacity is driven by a i=Xs+1 s Markov process and the instantaneous capacity has a specific Denote S(t) ≡ S(0,t). The time average of the cumulative distribution with respect to a specific state transition, the capacity through [0,t) is defined as transient capacity: additive capacity together with the underlying Markov process, S(t) i.e., cumulative capacity, form a Markov additive process, C(t)= . (5) t which is a bivariate process with strong Markov property and the increments are conditionally independent given a Example 1. For a single input single output channel, if the realization of the underlying Markov process. channel side information is only known at the receiver, the instantaneous capacity is expressed as [3] In other words, a Markov additive process is a non- stationary additive process defined on a Markov process [13], C(t)= W log 1+ γ|h(t)|2 , (6) 2 [14], if the Markov process has only one state, it reduces where |h(t)| denotes the envelope of h(t), γ = P/N0W to a Markov process with additive increments.
Details
-
File Typepdf
-
Upload Time-
-
Content LanguagesEnglish
-
Upload UserAnonymous/Not logged-in
-
File Pages9 Page
-
File Size-