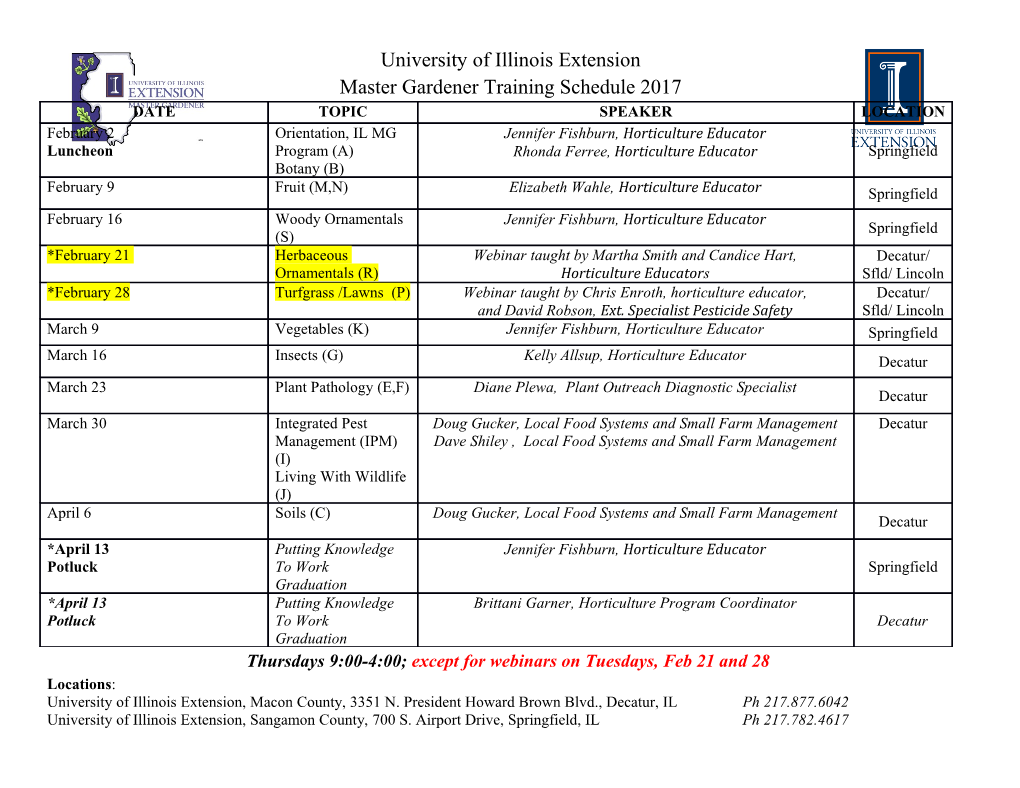
IMAGE DEMOSAICING BY USING ITERATIVE RESIDUAL INTERPOLATION Wei Ye and Kai-Kuang Ma School of Electrical and Electronic Engineering Nanyang Technological University, Singapore 639798 [email protected], [email protected] ABSTRACT A new demosaicing approach has been introduced recently, which is based on conducting interpolation on the generated residual fields rather than on the color-component difference fields as commonly practiced in most demosaicing methods. In view of its attractive performance delivered by such resid- ual interpolation (RI) strategy, a new RI-based demosaicing (a) (b) (c) method is proposed in this paper that has shown much im- Fig. 1. Comparison of color-component differences and proved performance. The key success of our approach lies in residuals: (a) a full color image, “Kodak 3”; (b) the color- that the RI process is iteratively deployed to all the three chan- component difference field, R-G; (c) the residual field, R-R.¯ nels for generating a more accurately reconstructed G chan- nel, from which the R channel and the B channel can be better Recently, a new demosaicing approach is proposed in [8], reconstructed as well. Extensive simulations conducted on which is based on the so-called residual interpolation (RI). two commonly-used test datasets have clearly demonstrated Instead of conducting interpolation on the color-component that our algorithm is superior to the existing state-of-the-art difference fields, the demosaicing algorithm as described demosaicing methods, both on objective performance evalua- in [8] performs interpolation over the residual fields. Note tion and on subjective perceptual quality. that a residual incurred at each pixel is the difference yielded Index Terms— Image demosaicing, regression filter, it- between a known color value (i.e., ground truth) and its es- erative residual interpolation. timated value. If the estimated values are sufficiently close to the ground truth, the resulted residual field will be much smoother than the color-component difference field, espe- 1. INTRODUCTION cially when there is a sharp color transition (e.g., Fig. 1). This indicates that interpolation conducted on the residual A color image captured by a single image sensor is denoted fields has a potential to yield a better reconstructed image. as a mosaiced image. This is commonly achieved by using a However, it has been observed that the RI-based demo- color filter array (CFA), on which the pixels are arranged in saicing method as described in [8] did not fully explore the the Bayer pattern [1], such that only one of the three primary RI-based strategy, since the reconstruction of the G channel is color components (i.e., R, G, or B) is recorded at each pixel mainly based on another approach, called the gradient-based location. Demosaicing is the reverse process of mosaicing, threshold free (GBTF) [5]. In this paper, a new demosaicing which restores an approximate full color image based on the algorithm is developed by exploiting the RI-based strategy to acquired mosaiced image. One strategy commonly practiced the G channel, using a new reconstruction scheme that itera- in the majority of demosaicing methods [2–7] is to interpo- tively employs the RI process to all the three channels. Simu- late those missing pixels on the G channel first, for this chan- lation results obtained from extensive experiments conducted nel has the highest sampling density among all three channels on the Kodak and the IMAX datasets have clearly shown that in the Bayer pattern; to be exact, the number of pixels with the proposed iterative RI-based demosaicing algorithm out- known values are extactly twice that of the R channel and performs all existing state-of-the-art demosaicing methods. of the B channel, respectively. The missing pixels on the R The rest of this paper is organized as follows. A succinct and B channels are then restored by interpolating the color- review of the RI process employed by [8] is given in Section component difference fields R-G and B-G, respectively. This 2. Section 3 describes the proposed demosaicing algorithm in is based on the observation that the color-component differ- detail. Section 4 conducts performance evaluation of several ences are usually locally smooth. comparable methods. Section 5 concludes the paper. 978-1-4799-5751-4/14/$31.00 ©2014 IEEE 1862 ICIP 2014 is immaterial). Consequently, two G channel images will be Guidance Stage 1. Generating the estimated image Image Estimated generated and are subject to be linearly combined together 푑 = 퐺 Image 푝 = 푅 to produce the final reconstructed G channel. The horizontal Input Regression processing flow is depicted in Fig. 3. For the vertical direc- Image 푝 = 푅 Filtering tion, the entire operations will be performed in the same way, except along the vertical direction. - Interpolated Reconstructed Stage 2. Interpolating the residuals Residuals Image 3.1. Iterative Residual Interpolation ∆푝 = ∆푅 푞 = 푅 Residuals Bilinear Given a Bayer mosaiced image (Fig. 3 (a)), a simple fi- ∆푝 = ∆푅 Interpolation + nite impulse response (FIR) filter, with three coefficient taps [1=2; 1; 1=2], is first applied to each channel for conduct- - denotes that the subtraction operation is only applied to pixels with known R values ing linear interpolation to produce three interpolated images, ~(1) ~(1) ~(1) Fig. 2. The RI-based reconstruction scheme for the R channel R , G , and B (Fig. 3 (b)). The RI process is then and for the B channel (not shown). employed to refine each interpolated channel image (as the input image, p) under the guidance of another interpolated 2. RESIDUAL INTERPOLATION channel image (as the guidance image, d). In our approach, the RI process is used as a refining process, rather than a Residual interpolation (RI) is introduced in [8] for conduct- reconstruction process as employed in [8]; the RI is exploited ing image demosaicing to restore those missing pixel values not only to the initially interpolated pixel values but also to on an incomplete image p under the guidance of a complete the ones in the follow-up iterations. image d. Fig. 2 shows its RI-based reconstruction scheme for Note that all the three channels should help each other restoring the R channel (likewise, for the B channel). during the refining process, since the known values of the For each color channel under reconstruction, the RI pro- three channels are non-overlapped on the Bayer mosaiced im- cess consists of two stages: (1) generate the estimated channel age. Therefore, each image in Fig. 3(b) can serve both roles image and compute the corresponding residual field, and (2) — i.e., as the input image p and as the guidance image d. By interpolate the residual field for compensating it back to the using the regression filter, three estimated images, R¯(1), G¯(1), estimated channel image. In Stage (1), the estimated image p¯ and B¯(1), are generated (Fig. 3 (c)). That is, is obtained by using the regression filter (also known as the R¯(1) = < R~(1)jG~(1) and B¯(1) = < B~(1)jG~(1) ; (2) guided filter) [9] as R~ B~ P P a(u; v) b(u; v) (1) (1) (1) (1) (u;v)2wi;j (u;v)2wi;j where G~ [ G~ = G~ , and the subscript R~ in G~ de- p¯(i; j) = · d(i; j) + ; R~ B~ R~ MN MN ~(1) (1) notes that only those rows in G that correspond to the rows in R~(1) that have values will be involved in the regression where wi;j is the M ×N local window centered at pixel (i; j). filtering process. (The same interpretation goes to G~(1), like- The coefficients a(u; v) and b(u; v) are first computed at each B~ pixel location (u; v) by performing linear regression between wise). As discussed previously, the initially interpolated im- ages G~(1) and G~(1) can be also refined under the guidance of p and d over the M ×N local window wu;v centered at (u; v). R~ B~ For ease of presentation, the above-described regression fil- R~(1) and of B~(1), separately. Therefore, tering process is denoted as: p¯ = <(pjd). Then, the residuals can be computed as ∆p = p − p¯, involving only those known G¯(1) = < G~(1)jR~(1) and G¯(1) = < G~(1)jB~(1) : (3) R~ R~ B~ B~ values. In Stage (2), the bilinear interpolation is exploited to ∆p to obtain the estimated ∆p for being compensated back to The estimated images as shown in Fig. 3(c) are then sub- the estimated image p¯ to generate the reconstructed image q. tracted from their corresponding Bayer mosaiced channel im- ages in Fig. 3(a), respectively, to generate the residual fields (1) (1) (1) 3. PROPOSED DEMOSAICING ALGORITHM WITH ∆R , ∆G , and ∆B as shown in Fig. 3(d). The 3- ITERATIVE RESIDUAL INTERPOLATION tap FIR filter used previously is exploited here again to con- duct linear interpolation for each residual field along the hor- A new RI-based demosaicing algorithm is proposed, in which izontal direction to generate the interpolated residual fields (1) (1) (1) the RI process is deployed through a unified and iterative way ∆R , ∆G , and ∆B as shown in Fig. 3(e). These to all the three channels to reconstruct a much more accurate fields are then added back to the estimated channel images G channel, from which the R and B channels can be more to give the refined images R^(1), G^(1), and B^(1)as illustrated accurately restored as well. The proposed iterative RI-based in Fig. 3(f). The above-described refining process will be re- reconstruction scheme will be conducted along the horizon- peated for further refining the channel images through itera- tal direction and the vertical direction individually (the order tions (i.e., k = 2; 3;:::;K).
Details
-
File Typepdf
-
Upload Time-
-
Content LanguagesEnglish
-
Upload UserAnonymous/Not logged-in
-
File Pages5 Page
-
File Size-