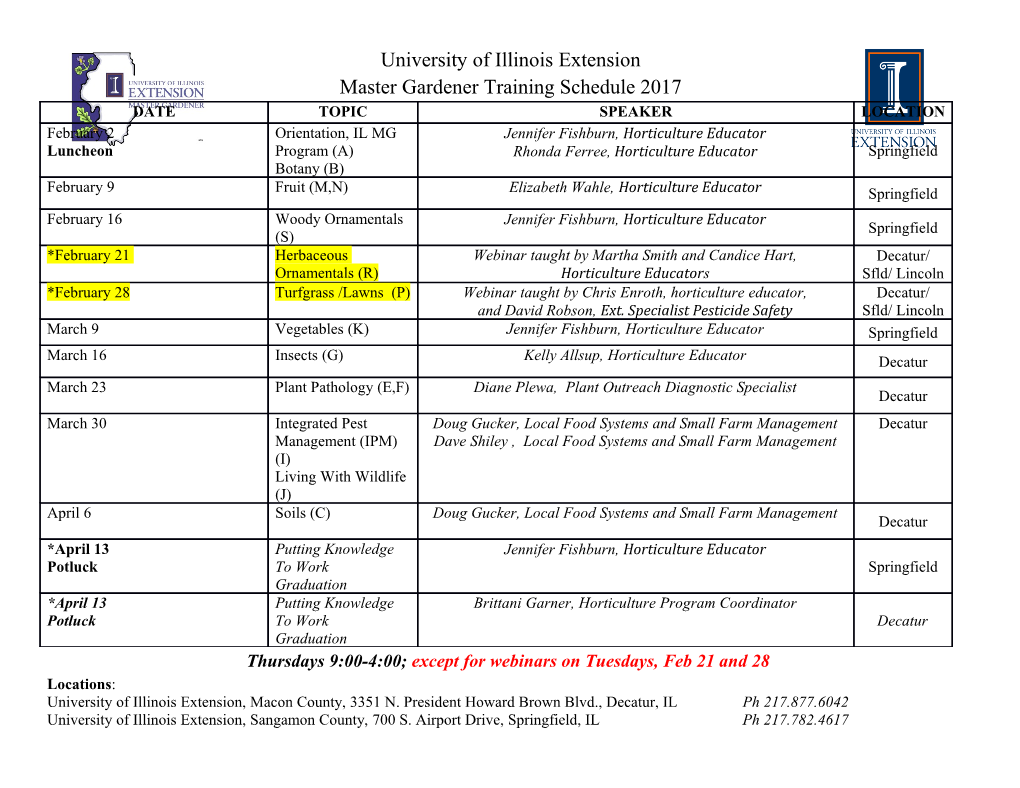
DISSERTATION GPU-based Multi-Volume Rendering of Complex Data in Neuroscience and Neurosurgery ausgeführt zum Zwecke der Erlangung des akademischen Grades eines Doktors der technischen Wissenschaften unter der Leitung von Ao.Univ.Prof. Dipl.-Ing. Dr.techn. Eduard Gröller, Institut E186 für Computergraphik und Algorithmen, und Dipl.-Ing. Dr.techn. Markus Hadwiger, VRVis Zentrum für Virtual Reality und Visualisierung, und Dipl.-Math. Dr.techn. Katja Bühler, VRVis Zentrum für Virtual Reality und Visualisierung, eingereicht an der Technischen Universität Wien, bei der Fakultät für Informatik, von Dipl.-Ing. (FH) Johanna Beyer, Matrikelnummer 0426197, Gablenzgasse 99/24 1150 Wien Wien, im Oktober 2009 DISSERTATION GPU-based Multi-Volume Rendering of Complex Data in Neuroscience and Neurosurgery Abstract Recent advances in image acquisition technology and its availability in the medical and bio-medical fields have lead to an unprecedented amount of high-resolution imaging data. However, the inherent complexity of this data, caused by its tremendous size, complex structure or multi-modality poses several challenges for current visualization tools. Recent developments in graphics hardware archi- tecture have increased the versatility and processing power of today’s GPUs to the point where GPUs can be considered parallel scientific computing devices. The work in this thesis builds on the current progress in image acquisition techniques and graphics hardware architecture to develop novel 3D visualization methods for the fields of neurosurgery and neuroscience. The first part of this thesis presents an application and framework for plan- ning of neurosurgical interventions. Concurrent GPU-based multi-volume ren- dering is used to visualize multiple radiological imaging modalities, delineating the patient’s anatomy, neurological function, and metabolic processes. Addition- ally, novel interaction metaphors are introduced, allowing the surgeon to plan and simulate the surgial approach to the brain based on the individual patient anatomy. The second part of this thesis focuses on GPU-based volume rendering tech- niques for large and complex EM data, as required in the field of neuroscience. A new mixed-resolution volume ray-casting approach is presented, which circum- vents artifacts at block boundaries of different resolutions. NeuroTrace is intro- duced, an application for interactive segmentation and visualization of neural processes in EM data. EM data is extremely dense, heavily textured and exhibits a complex structure of interconnected nerve cells, making it difficult to achieve high-quality volume renderings. Therefore, this thesis presents a novel on-demand nonlinear noise removal and edge detection method which allows to enhance im- portant structures (e.g., myelinated axons) while de-emphasizing less important regions of the data. In addition to the methods and concepts described above, this thesis tries to bridge the gap between state-of-the-art visualization research and the use of those visualization methods in actual medical and bio-medical applications. iii Kurzfassung Technische Fortschritte in bildgebenden Verfahren und deren weite Verfügbarkeit im medizinischen und bio-medizinischen Bereich haben zu einer noch nie dagewe- senen Menge an hochaufgelösten Bilddaten geführt. Für die meisten Visualisie- rungs-Werkzeuge stellt jedoch die Komplexität dieser Daten, hervorgerufen durch ihre enorme Größe, Auflösung, komplexe Struktur oder Multi-Modalität große Herausforderungen dar. Die vorliegende Arbeit baut auf den aktuellen Entwick- lungen im Bereich der bildgebenden Verfahren und der hohen Performanz und Flexibilität heutiger Grafikkarten auf, um neue Methoden zur 3D Visualisierung in der Neurochirurgie und Neurobiologie zu entwickeln. Der erste Teil der Arbeit beschreibt eine Anwendung für die Planung von neu- rochirurgischen Eingriffen. Es werden GPU-basierte Multi-Volume-Rendering- Methoden entwickelt, um die Volumendaten mehrerer unterschiedlicher Bild-Mo- dalitäten gleichzeitig darstellen zu können. Diese kombinierte Visualisierung er- laubt die genaue Darstellung der individuellen Anatomie, neurologischen Funk- tionen und Stoffwechselvorgänge der Patienten. Darüber hinaus werden neue Interaktions-Metaphern eingeführt, die es Chirurgen ermöglichen, den operativen Zugang zum Gehirn patientenindividuell zu planen und zu simulieren. Der zweite Teil der Arbeit konzentriert sich auf GPU-basierte Volume-Ren- dering-Techniken für die Darstellung großer und komplexer EM Daten, wie sie im Bereich der Neurowissenschaften vorhanden sind. Ein neuer auf unterschiedliche Auflösungsstufen beruhender Volume-Ray-Casting-Ansatz ermöglicht die artefak- tfreie Darstellung von Blöcken mit unterschiedlichen Auflösungsstufen. Schließlich wird NeuroTrace vorgestellt, ein Programm zur interaktiven Segmentierung und Visualisierung neuronaler Prozesse in EM-Daten. Um die Qualität von abge- bildeten EM-Daten zu verbessern, wird eine neue bedarfsorientierte und nicht- lineare Rauschunterdrückung und Kantendetektierungsmethode beschrieben, die wichtige Strukturen in EM-Daten (z.B. myelinisierte Axone) hervorhebt, während weniger wichtige Regionen des Volumens ausgeblendet werden. Zusätzlich zu den oben beschriebenen Methoden und Konzepten, versucht diese Dissertation eine Brücke zwischen dem momentanen Stand der Forschung im Bereich der Volu- menvisualisierung und deren Integration in tatsächliche medizinische und bio- medizinische Anwendungen zu schlagen. v Contents Abstract iii Related Publications xi 1 Introduction 1 1.1 Contribution . 2 1.2 Organization . 3 Multi-Volume Rendering for Neurosurgery 7 2 Introduction 7 2.1 Medical Image Data . 8 2.1.1 X-Rays . 9 2.1.2 Computed Tomography . 9 2.1.3 Magnetic Resonance Imaging . 9 2.1.4 Functional Magnetic Resonance Imaging . 10 2.1.5 Digital Subtraction Angiography . 10 2.1.6 Positron Emission Tomography . 10 2.1.7 Imaging Artifacts . 11 2.2 Image Processing for Medical Data . 12 2.2.1 Filtering and De-noising . 12 2.2.2 Segmentation . 13 2.2.3 Registration and Resampling . 13 2.3 Visualization of Medical Data . 14 2.3.1 Volume Rendering . 16 2.3.2 Volume Visualization for Neurosurgery . 18 vii 3 Skull Peeling 23 3.1 Introduction . 23 3.2 Related Work . 25 3.3 Skull Peeling . 26 3.4 Neurosurgical Applications . 27 3.4.1 Surgical Approach to the Brain . 28 3.4.2 Epilepsy Surgery Planning . 31 3.5 Results and Evaluation . 31 3.6 Summary and Conlusion . 31 4 Preoperative Planning of Neurosurgical Interventions 33 4.1 Introduction . 33 4.2 Related Work . 37 4.3 Workflow . 38 4.4 Preprocessing Stage . 39 4.5 Visualization Modules . 40 4.5.1 Multi-Volume Rendering . 40 4.5.2 Skull Peeling - Surgical Approach to the Brain . 43 4.5.3 Multi-Volume Blending – Brain Surface Visualization . 43 4.5.4 Segmented Multi-Volume Rendering – Deep Lesions . 44 4.5.5 Smooth Rendering of Segmented Multi-Volume Data . 46 4.5.6 Interaction Aides . 49 4.6 Results and Evaluation . 49 4.7 Summary and Conclusion . 54 Volume Rendering of Large Complex Biological Data 59 5 Introduction 59 5.1 Connectomics . 60 5.1.1 Brain Anatomy . 60 5.1.2 Electron Microscopy Data . 61 5.1.3 Processing Pipeline . 62 5.2 Large Data Volume Rendering . 63 5.2.1 Multi-Resolution Techniques . 64 5.2.2 Compression and Packing Techniques . 66 6 Smooth Mixed-Resolution GPU Volume Rendering 69 6.1 Introduction . 69 6.2 Related Work . 71 6.3 Mixed-Resolution Volume Rendering . 72 6.3.1 Volume Subdivision for Texture Packing . 73 6.3.2 Mixed-Resolution Texture Packing . 74 6.3.3 Address Translation . 75 6.4 Smooth Mixed-Resolution Interpolation . 76 6.4.1 Smooth Transition Interpolation . 76 6.4.2 Volume Rendering Fragment Shader Modification . 82 6.4.3 Brick Cache Fixup . 83 6.5 Results and Evaluation . 84 6.6 Summary and Conclusion . 86 7 Visualization of Neural Processes in EM Datasets 87 7.1 Introduction . 87 7.2 Previous Work . 89 7.3 NeuroTrace . 89 7.3.1 Pre-Processing . 91 7.3.2 NeuroTrace Workflow . 91 7.3.3 NeuroTrace Framework . 92 7.4 Volume Visualization . 93 7.4.1 On-demand Filtering . 94 7.4.2 Noise Removal . 95 7.4.3 Local Histogram-based Edge Detection . 95 7.4.4 Dynamic Caching . 96 7.4.5 GPU Implementation . 97 7.5 Results and Evaluation . 98 7.6 Summary and Conclusion . 99 8 Summary and Conclusions 101 Acknowledgments 103 Bibliography 105 Curriculum Vitae 115 Related Publications This thesis is based on the following publications: J. Beyer, M. Hadwiger, S. Wolfsberger, C. Rezk-Salama, and K. Bühler. Seg- mentierungsfreie Visualisierung des Gehirns für Direktes Volume Ren- dering . In Proceedings of Bildverarbeitung für die Medizin 2007 , pages 333–337, 2007. J. Beyer, M. Hadwiger, S. Wolfsberger, and K. Bühler. High-Quality Multi- modal Volume Rendering for Preoperative Planning of Neurosurgical Interventions . IEEE Transactions on Visualization and Computer Graphics (Proceedings of IEEE Visualization 2007) , 13(6), pages 1696–1703, 2007. J. Beyer, M. Hadwiger, T. Möller, and L. Fritz. Smooth Mixed-Resolution GPU Volume Rendering. In Proceedings of IEEE International Symposium on Volume and Point-Based Graphics (VG 2008) , pages 163–170, 2008. W.-K. Jeong, J. Beyer, M. Hadwiger, A. Vasquez, H. Pfister, and R. Whitaker. Scalable and Interactive Segmentation and Visualization of Neural Pro- cesses in EM Datasets . IEEE Transactions on Visualization and Computer Graphics (Proceedings of IEEE Visualization 2009) , 15(6), pages 1505–1514, 2009. Other publications: J. Beyer, C. Langer, L. Fritz, M. Hadwiger, S. Wolfsberger, and K. Bühler. In- teractive Diffusion
Details
-
File Typepdf
-
Upload Time-
-
Content LanguagesEnglish
-
Upload UserAnonymous/Not logged-in
-
File Pages131 Page
-
File Size-