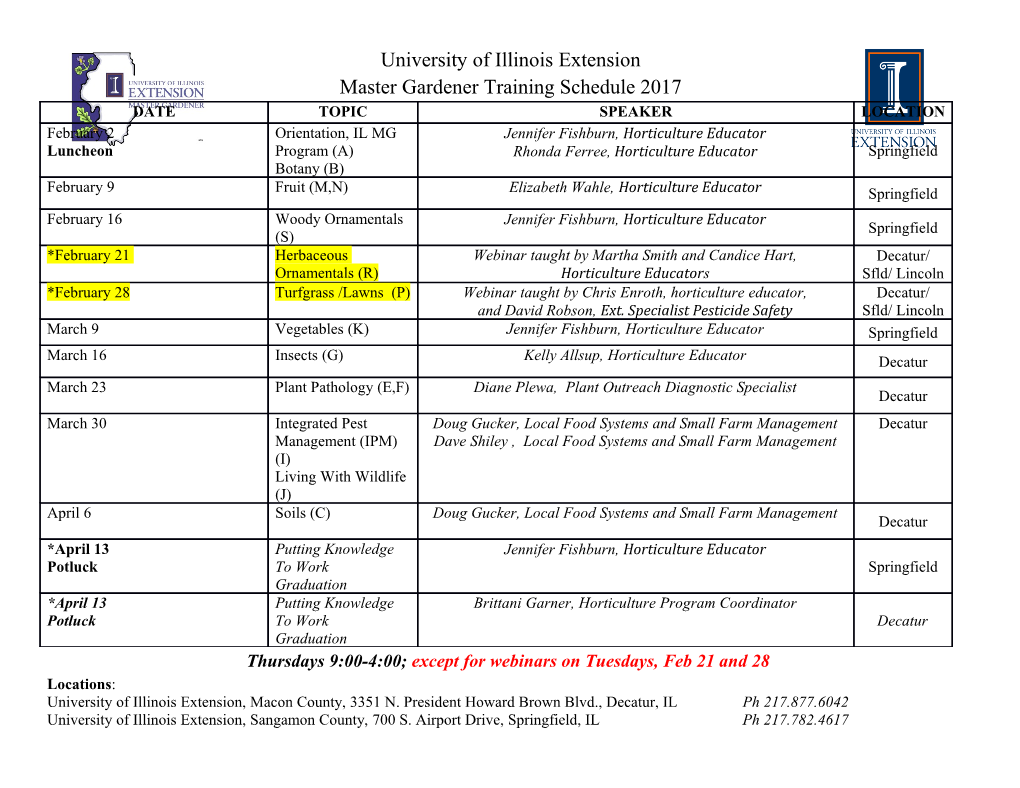
Laws of Total Expectation and Total Variance Definition of conditional density. Assume and arbitrary random variable X with density fX . Take an event A with P (A) > 0. Then the conditional density fXjA is defined as follows: 8 < f(x) 2 P (A) x A fXjA(x) = : 0 x2 = A Note that the support of fXjA is supported only in A. Definition of conditional expectation conditioned on an event. Z Z 1 E(h(X)jA) = h(x) fXjA(x) dx = h(x) fX (x) dx A P (A) A Example. For the random variable X with density function 8 < 1 3 64 x 0 < x < 4 f(x) = : 0 otherwise calculate E(X2 j X ≥ 1). Solution. Step 1. Z h i 4 1 1 1 x=4 255 P (X ≥ 1) = x3 dx = x4 = 1 64 256 4 x=1 256 Step 2. Z Z ( ) 1 256 4 1 E(X2 j X ≥ 1) = x2 f(x) dx = x2 x3 dx P (X ≥ 1) fx≥1g 255 1 64 Z ( ) ( )( ) h i 256 4 1 256 1 1 x=4 8192 = x5 dx = x6 = 255 1 64 255 64 6 x=1 765 Definition of conditional variance conditioned on an event. Var(XjA) = E(X2jA) − E(XjA)2 1 Example. For the previous example , calculate the conditional variance Var(XjX ≥ 1) Solution. We already calculated E(X2 j X ≥ 1). We only need to calculate E(X j X ≥ 1). Z Z Z ( ) 1 256 4 1 E(X j X ≥ 1) = x f(x) dx = x x3 dx P (X ≥ 1) fx≥1g 255 1 64 Z ( ) ( )( ) h i 256 4 1 256 1 1 x=4 4096 = x4 dx = x5 = 255 1 64 255 64 5 x=1 1275 Finally: ( ) 8192 4096 2 630784 Var(XjX ≥ 1) = E(X2jX ≥ 1) − E(XjX ≥ 1)2 = − = 765 1275 1625625 Definition of conditional expectation conditioned on a random variable. Suppose two random variables X and Y . To define E(XjY ) we need the conditional density function. For this , let fX;Y (x; y) be the joint density of the pair fX; Y g. Thsn the conditional density fXjY is defined 8 > fX;Y (x;y) > fY (y) > 0 < fY (y) f j (xjy) = X Y > :> 0 fY (y) = 0 R 1 Then E(h(X) j Y ) is defined to be the random variable that assigns the value −∞ h(x) fXjY (xjy) dx P to y in the continuous case, and assigns the value h(x) fXjY (xjy) in the discrete case. x Example. Take the following joint density: X=1 X=2 X=3 fY (y) Y = 1 0.07 0.1 0.23 0.4 Y = 2 0.25 0.08 0.07 0.4 Y = 3 0.05 0.04 0.11 0.2 fX (x) 0.37 0.22 0.41 1 Describe the random variable E(Y 2jX) 2 P P 2j 2 j 2 f(X=1 ;Y =y) Solution. E(Y X = 1) = y fY jX (y 1) = y f (1) y y X n o 1 X 1 1:52 = y2 f(X = 1 ;Y = y) = (1)2(0:07) + (2)2(0:25) + (3)2(0:05) = f (1) 0:37 0:37 X y Similarly: 0:78 E(Y 2jX = 2) = 0:32 1:5 E(Y 2jX = 3) = 0:41 So then: 8 > 1:52 <> 0:37 with probability P (X = 1) = 0:37 E(Y 2jX) = 0:78 > 0:32 with probability P (X = 2) = 0:22 > : 1:5 0:41 with probability P (X = 3) = 0:41 Law of Total Expectation. E(X) = E(E[XjY ]) Law of Total Variance. ( ) ( ) Var(X) = E Var[X j Y ] + Var E[X j Y ] Proof. By definition we have Var(XjY ) = E(X2jY ) − fE(XjY )g2 3 BX taking E of both sides we get: E[Var(XjY )] = E[E[X2jY ]] − E[fE(XjY )g2] = E(X2) − E[fE(XjY )g2] law of iterated expectations n o n o = E(X2) − fE(X)g2 − E[fE(XjY )g2] − fE(X)g2 n o = Var(X) − E[fE(XjY )g2] − fE[E(XjY )]g2 = Var(X) − Var[E(XjY )] By moving terms around , the claim follows. Note: Using similar arguments , one can prove the following: Example (from the Dean's note): Two urns contain a large number of balls with each ball marked with one number from the set f0; 2; 4g. The proportion of each type of ball in each urn is displayed in the table below: Number on Ball 0 2 4 A 0.6 0.3 0.1 B 0.1 0.3 0.6 An urn is randomly selected and then a ball is drawn at random from the urn. The number on the ball is represented by the random variable X. (a) Calculate the hypothetical means (or conditional means) E[Xjθ = A] and E[Xjθ = B] (b) Calculate the variance of the hypothetical means: Var[E[Xjθ]] . 4 (c) Calculate the process variances (or conditional variances) Var[Xjθ = A] and Var[Xjθ = B] (d) Calculate the expected value of the process variance: E[Var[Xjθ]] . (e) Calculate the total variance (or unconditional variance) Var[X] and show that it equals the sum of the quantities calculated in (b) and (d) . Solution: Part (a) E[Xjθ = A] = (0:6)(0) + (0:3)(2) + (0:1)(4) = 1:0 E[Xjθ = B] = (0:1)(0) + (0:3)(2) + (0:6)(4) = 3:0 Part (b) 1 1 E[X] = E[E[Xjθ]] = ( )(1:0) + ( )(3:0) = 2:0 2 2 1 1 Var[E[Xjθ]] = ( )(1:0 − 2:0)2 + ( )(3:0 − 2:0)2 = 1:0 2 2 Part (c) Var[Xjθ = A] = (0:6)(0 − 1:0)2 + (0:3)(2 − 1:0)2 + (0:1)(4 − 1:0)2 = 1:8 Var[Xjθ = B] = (0:1)(0 − 3:0)2 + (0:3)(2 − 3:0)2 + (0:6)(4 − 3:0)2 = 1:8 Part (d) 1 1 E[Var[Xjθ]] = ( )(1:8) + ( )(1:8) = 1:8 2 2 Part (e) h i Var[X] = 1 (0:6)(0 − 2:0)2 + (0:3)(2 − 2:0)2 + (0:1)(4 − 2:0)2 2 h i 1 − 2 − 2 − 2 + 2 (0:1)(0 2:0) + (0:3)(2 2:0) + (0:6)(4 2:0) = 2:8 ) Var(X) = Var[E[Xjθ]] + E[Var[Xjθ]] 5.
Details
-
File Typepdf
-
Upload Time-
-
Content LanguagesEnglish
-
Upload UserAnonymous/Not logged-in
-
File Pages5 Page
-
File Size-