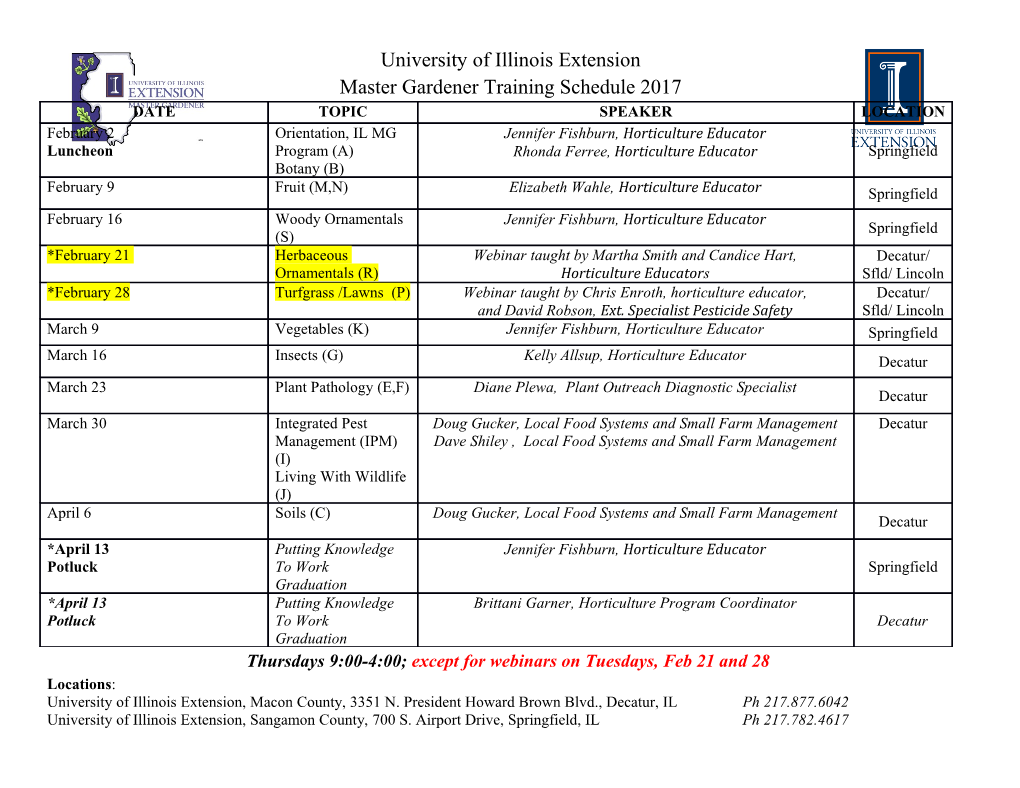
Stat 6550 (Spring 2016) – Peter F. Craigmile A time series model Introduction to time series models and stationarity Remember that a time series model is a specification of the Reading: Brockwell and Davis [2002, Chapter 1, Appendix A] • probabilistic distribution of a sequence of random variables (RVs) A time series model Xt : t T0 . • { ∈ } – Example: IID noise (The observed time series x is a realization of the RVs). { t} – Mean function The simplest time series model is IID noise: • – Autocovariance and autocorrelation functions – Here X is a sequence of independent and identically dis- { t} Strictly stationary processes • tributed (IID) RVs with (typically) zero mean. (Weakly) stationary processes – There is no dependence in this model • – Autocovariance (ACVF) and autocorrelation function (ACF). (but this model can be used as a building block for construct- ing more complicated models) – The white noise process – We cannot forecast future values from IID noise – First order moving average process, MA(1) (There are no dependencies between the random variables at – First order autoregressive process, AR(1) different time points). The random walk process • Gaussian processes • 1 2 Example realizations of IID noise Means and autocovariances 1. Gaussian (normal) IID noise with mean 0, variance σ2 > 0. A time series model could also be a specification of the means 2 1 xt • pdf is fXt(xt)= exp √ 2 −2σ2 and autocovariances of the RVs. 2πσ The mean function of X is • { t} µX(t) = E(Xt). Think of µX(t) are being the theoretical mean at time t, taken IID Normal(0, 0.25) noise −3 −2 −1 0 1 2 3 over the possible values that could have generated Xt. 0 20 40 60 80 100 120 time When X is a continuous RV, • t 2. Bernoulli IID noise, with success probability p. ∞ µX(t) = xfXt(x) dx, Z−∞ P (Xt =1)= p =1 P (Xt = 0). − where f ( ) is the probability density function (pdf) for X . Xt · t When X is a discrete RV, • t ∞ µX(t) = xfXt(x), X−∞ IID Bernoulli(p=0.5) noise −3 −2 −1 0 1 2 3 where fXt( ) is the probability mass function (pmf) for Xt. 0 20 40 60 80 100 120 · time Note: Variances are equal in both plots (but means are not!) 3 4 Examples of mean functions Review: The covariance between two RVs Example 1: What is µ (t) for η an IID N(0, σ2) process? The covariance between the RVs X and Y is • η { t} • cov(X,Y ) = E (X µ )(Y µ ) { − X − Y } = E(XY ) µ µ . − X Y It is a measure of linear dependence between the two RVs. Example 2 (Straight line trend plus IID noise model): • When X = Y we have • For each t, let Xt = α + βt + ηt, where α and β are constants, cov(X,X) = var(X). and ηt is defined above. What is µX(t)? For constants a, b, c, and RVs X, Y , Z: • cov(aX + bY + c,Z) = cov(aX, Z) + cov(bY,Z) = a cov(X,Z)+ b cov(Y,Z). Thus • var(X + Y ) = cov(X,X) + cov(X,Y ) + cov(Y,X) + cov(Y,Y ) = var(X) + var(Y )+2 cov(X,Y ). 5 6 Autocovariance function Why non-negative definite functions? The autocovariance function of X is A function f( , ) is non-negative definite if • { t} • · · n n γX(s,t) = cov(Xs,Xt) = E[(Xs µX(s))(Xt µX(t))]. a f(s,t) a 0, − − s t ≥ s=1 t=1 X X Measures the strength of linear dependence between the two RVs for all positive integers n and real-valued constants a , . , a . • 1 n X and X . s t So, why must γ be a non-negative definite function? • X Properties: • Consider the following: – For any integer n, and real constants a1, . , an, let 1. γX(s,t)= γX(t,s) for each s and t. n 2. When s = t we obtain Y = atXt. t=1 2 X γX(t,t) = cov(Xt,Xt) = var(Xt) = σX(t), – Then the variance of Y is equal to n n the variance function of Xt . { } var(Y ) = cov(Y,Y ) = cov asXs, atXt s=1 t=1 ! 3. γX(s,t)isa non-negative definite function n Xn X = asatcov(Xs,Xt) s=1 t=1 Xn Xn = asatγX(s,t). s=1 t=1 X X – Thus if γX is a non-negative definite function: 7 8 Autocorrelation function Strictly stationary processes The autocorrelation function of X is In strict stationarity the joint distribution of a set of RVs are • { t} • γX(s,t) unaffected by time shifts. ρX(s,t) = corr(Xs,Xt) = . γX(s,s)γX(t,t) A time series, Xt , is strictly stationary if Measures the strength of linear associationp between the two RVs • { } • (Xt1,...,Xtn) =d (Xt1+h,...,Xtn+h) Xs and Xt. Properties: for all integers h and n 1, and time points tk . • ≥ { } (Here =d means “equal in distribution to”.) 1. 1 ρ (s,t) 1 for each s and t. − ≤ X ≤ Then: 2. ρX(s,t)= ρX(t,s) for each s and t. • 3. ρ (t,t) = 1 for each t. 1. Xt is identically distributed X { } 4. ρX(s,t)isa non-negative definite function. – Not necessarily independent! 2. (Xt,Xt+h)=d (X1,X1+h) for all t and h; 3. When µX is finite, µX(t)= µX is independent of time t. 4. When the variance function exists, γX(s,t)= γX(s + h,t + h) for any s, t and h. 9 10 (Weakly) stationary processes Autocovariance function of a stationary process X is (weakly) stationary if The autocovariance function of a stationary process •{ t} • Xt (ACVF) is defined to be 1. E(Xt) = µX(t) = µX for some constant µX which does not { } depend on t. γX(h) = cov(Xt,Xt+h) 2. cov(X ,X )= γ (t,t + h)= γ (h), a finite constant that = E[(X µ )(X + µ )] . t t+h X X t − X t h − X can depend on h but not on t. Measures the amount of dependence between X and X + . • t t h The quantity h is called the lag. • Properties of the ACVF: • Other terms for this type of stationarity include second-order, • 1. γ (0) = var(X ). covariance, wide sense. X t 2. γ ( h)= γ (h) for each h. X − X Relating weak and strict stationarity: 3. γ (s t) as a function of s and t is non-negative definite. • X − 1. A strictly stationary process X is also weakly stationary { t} as long as µX(t) is finite for all t. 2. Weak stationarity does not imply strict stationarity! 11 12 Autocorrelation function of a stationary process The white noise process The autocorrelation function of a stationary process Assume E(X )= µ, var(X )= σ2 < . • • t t ∞ Xt (ACF) is defined to be 2 { } Xt is a white noise or WN(µ,σ ) process if •{ } γX(h) ρX(h) = . γX(0) γX(h)=0, Exercise: prove that this it true from previous definitions. for h = 0. • 6 Properties of the ACF: X is clearly stationary. • •{ t} 1. 1 ρ (h) 1 for each h. − ≤ X ≤ But, distributions of X and X +1 can be different! 2. ρ(0) = 1. • t t 3. ρ ( h)= ρ (h) for each h. All IID noise with finite variance is white noise (but, the X − X • 4. ρ (s t) as a function of s and t is non-negative definite. converse need not be true). X − 13 14 Examples realizations of white noise processes The moving average process of first order, MA(1) IID N(2,4) noise Let Z be a WN(0,σ2) process and θ be some real-valued con- • { t} stant. For each integer t let, ACF realization Xt = Zt + θZt 1. −2 0 2 4 6 8 − −1.0 0.0 0.5 1.0 0 20 40 60 80 100 120 0 2 4 6 8 10 The sequence of RVs X is called the moving average pro- time lag • { t} IID chisq(2) noise cess of order 1 or MA(1) process. Extends naturally to the MA(q) process). ACF realization By calculating µX(t) and γX(t,t + h) show that the MA(1) pro- −2 0 2 4 6 8 • −1.0 0.0 0.5 1.0 cess X is stationary. Also calculate the ACF. 0 20 40 60 80 100 120 0 2 4 6 8 10 { t} time lag 15 16 MA(1) process (cont.) MA(1) process (cont.) 17 18 Examples realizations of MA(1) processes First order autoregressive process, AR(1) The same Z sequence is used in each case. Let Z be a WN(0,σ2) process, and 1 <φ< 1 be a constant. • { t} • { t} − theta=0.25 Assume: • 1. Xt is a stationary process with ACF { } realization Xt = φXt 1 + Zt, −4 −2 0 2 4 − −1.0 0.0 0.5 1.0 0 20 40 60 80 100 120 0 2 4 6 8 10 time lag for each integer t. theta=0.75 2. Xs and Zt are uncorrelated for each s<t (future noise in uncorrelated with the current time point). ACF realization We will see later there is only one unique solution to this equation. −4 −2 0 2 4 −1.0 0.0 0.5 1.0 • 0 20 40 60 80 100 120 0 2 4 6 8 10 Such a sequence Xt of RVs is called an AR(1) process.
Details
-
File Typepdf
-
Upload Time-
-
Content LanguagesEnglish
-
Upload UserAnonymous/Not logged-in
-
File Pages14 Page
-
File Size-