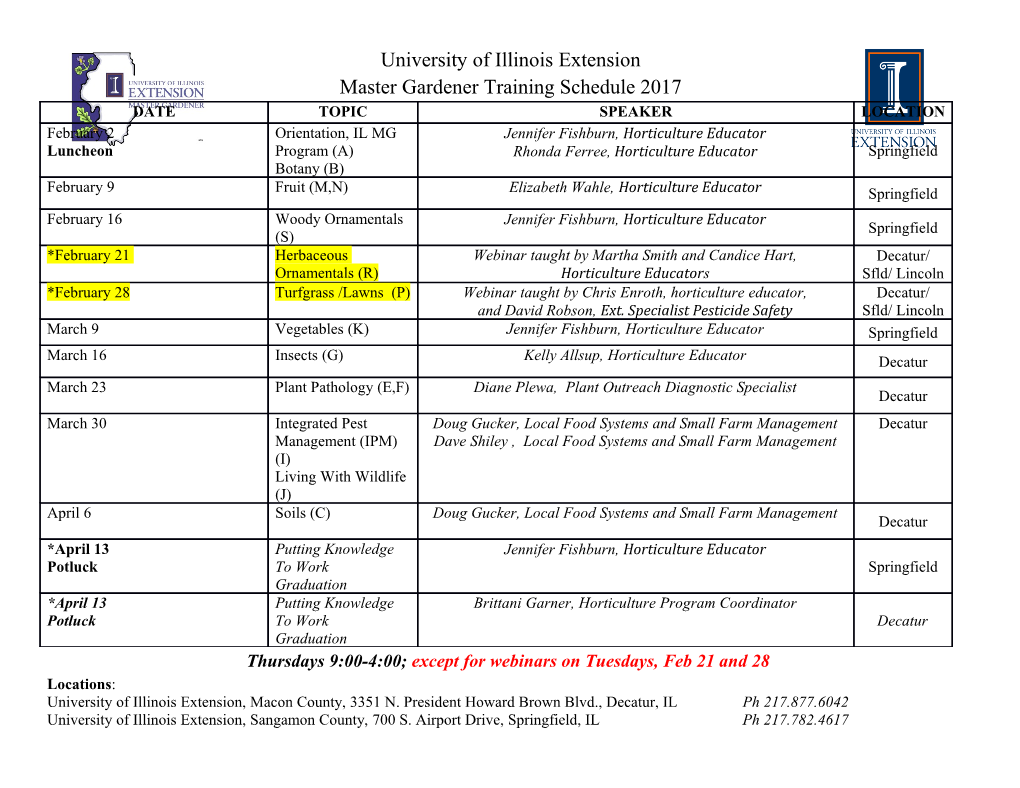
Short Paper CIKM’18, October 22-26, 2018, Torino, Italy An Adversarial Approach to Improve Long-Tail Performance in Neural Collaborative Filtering Adit Krishnany, Ashish Sharma∗, Aravind Sankary, Hari Sundaramy yUniversity of Illinois at Urbana-Champaign, IL, USA ∗Microsoft Research, Bangalore, India y{aditk2, asankar3, hs1}@illinois.edu ∗[email protected] ABSTRACT Figure 1: CDAE[15] and VAE-CF[9] recall for item-groups In recent times, deep neural networks have found success in Collabo- (decreasing frequency) in MovieLens (ml-20m). CDAE over- rative Filtering (CF) based recommendation tasks. By parametrizing fits to popular item-groups, falls very rapidly. VAE-CF has latent factor interactions of users and items with neural architec- better long-tail recall due to representational stochasticity. tures, they achieve significant gains in scalability and performance 1.0 over matrix factorization. However, the long-tail phenomenon in 0.9 VAE-CF recommender performance persists on the massive inventories of 0.8 CDAE online media or retail platforms. Given the diversity of neural archi- 0.7 tectures and applications, there is a need to develop a generalizable 0.6 and principled strategy to enhance long-tail item coverage. 0.5 In this paper, we propose a novel adversarial training strategy 0.4 to enhance long-tail recommendations for users with Neural CF (NCF) models. The adversary network learns the implicit associa- 0.3 tion structure of entities in the feedback data while the NCF model 0.2 0.1 is simultaneously trained to reproduce these associations and avoid Item Recall @ 50 (Mean over users) the adversarial penalty, resulting in enhanced long-tail performance. 0.0 Experimental results show that even without auxiliary data, ad- Item Groups Sorted by Decreasing Frequency versarial training can boost long-tail recall of state-of-the-art NCF 1 INTRODUCTION models by up to 25%, without trading-off overall performance. We Recommender systems play a pivotal role in sustaining massive evaluate our approach on two diverse platforms, content tag rec- product inventories on online media and retail platforms, and re- ommendation in Q&A forums and movie recommendation. duce information overload on users. Collaborative filtering methods personalize item recommendations based on historic interaction CCS CONCEPTS data (implicit feedback setting), with matrix-factorization being the • Information systems → Collaborative filtering; Recommender most popular approach [5]. In recent times, NCF methods [3, 9, 15] systems; • Computing methodologies → Neural networks; have transformed simplistic inner-product representations with non-linear interactions, parametrized by deep neural networks. KEYWORDS Although performance gains over conventional approaches are sig- nificant, a closer analysis indicates skew towards popular items Recommender Systems; Neural Collaborative Filtering; Adversarial (Figure 3) with ample evidence in the feedback (overfit to popular Learning; Long-Tail Phenomenon items), resulting in poor niche (long-tail) item recommendations to ACM Reference Format: users (see fig. 1). This stifles user experience and reduces platform Adit Krishnan, Ashish Sharma, Aravind Sankar, Hari Sundaram. 2018. An revenue from niche products with high profit margins. Adversarial Approach to Improve Long-Tail Performance in Neural Col- Conventional effort to challenge the long-tail in recommendation laborative Filtering. In Proceedings of The 27th ACM International Conference has been two-fold [16]. First, integration with neighbor-based mod- on Information and Knowledge Management (CIKM ’18). ACM, New York, els [10] to capture inter-item, inter-user and cross associations in NY, USA, 4 pages. https://doi.org/10.1145/3269206.3269264 the latent representations and second, incorporating auxiliary data (e.g. item descriptions) to overcome limited feedback [13] or hybrid methods [6, 11]. While neural models readily adapt auxiliary data Permission to make digital or hard copies of all or part of this work for personal or classroom use is granted without fee provided that copies are not made or distributed [8], the association/neighbor-based path is relatively unexplored for profit or commercial advantage and that copies bear this notice and the full citation due to the heterogeneity of representations and architectures. on the first page. Copyrights for components of this work owned by others than ACM Given the diversity of NCF architectures and applications [3, 8, 9], must be honored. Abstracting with credit is permitted. To copy otherwise, or republish, to post on servers or to redistribute to lists, requires prior specific permission and/or a architectural solutions may not generalize well. Instead we propose fee. Request permissions from [email protected]. to augment NCF training to levy penalties when the recommender CIKM ’18, October 22–26, 2018, Torino, Italy fails to identify suitable niche items for users, given their history © 2018 Association for Computing Machinery. ACM ISBN 978-1-4503-6014-2/18/10...$15.00 and global item co-occurrence. To achieve this, conventional neigh- https://doi.org/10.1145/3269206.3269264 bor models employ static pre-computed links between entities [10] 1491 Short Paper CIKM’18, October 22-26, 2018, Torino, Italy to regularize the learned representations. While it is possible to add we seek to balance the tradeoff between the popular item biased a similar term to the NCF objective, we aim to learn the associa- reconstruction objective against the recall and accuracy of long-tail tion structure rather than imposing it on the model. Towards this item recommendations. goal, we introduce an adversary network to infer the inter-item Towards the above objective, we introduce the adversary model association structures unlike link-based models, guided by item co- D in our learning framework to learn the inter-item association occurrences in the feedback data. The adversary network is trained structure in the feedback data and correlate G’s niche item rec- P in tandem with the recommender. It can readily integrate auxiliary ommendations with popular items in the user’s history, Xu . We data and be extended to model inter-user or cross associations. associate G’s niche item recommendations with u’s popular item For each user, a penalty is imposed on the recommender if the history since niche-popular pairings are the most informative (inter- suggested niche items do not correlate with the user’s history. The popular pairs are redundant, inter-niche pairs are noisy). The ad- adversary is trained to distinguish the recommender’s niche item versary D is trained to distinguish “fake" or synthetic pairings of P suggestions against actual item pairings sampled from the data. The popular and niche items sampled from Xu and fG(i j u; X) respec- more confident this distinction, the higher the penalty imposed. As tively, against “real" popular-niche pairs sampled from the global training proceeds, the adversary learns the inter-item association co-occurrence counts in X. The more confident this distinction by D, structure guided by the item pairs sampled from user records while the stronger the penalty on G. To overcome the applied penalty, G the recommender incorporates these associations, until mutual must produce niche item recommendations that are correlated with convergence. In summary, we make the following contributions: the user’s history. The model converges when both the synthetic • Unlike conventional neighbor models, our adversary model and true niche-popular pairs align with the association structure learns the association structure of entities rather than im- learned by D. We now formalize the strategy. posing pre-defined links on the recommender model. True & Synthetic Pair Sampling. • Our approach is architecture and application agnostic. • True Pairs : “True" popular-niche pairs (ip ;in ) 2 I P × • Experimental results on two diverse platforms show substan- IN are sampled from their global co-occurrence counts in tial gains (by upto 25%) in long-tail item recall for state-of- X. To achieve efficiency, we use the alias table method [7] the-art NCF models while not degrading overall results. which has O(1) amortized cost when repeatedly drawing We now present our problem formulation, model details (sec. 2, 3) samples from the same discrete distribution, compared to experimental results (sec. 4), and conclude in sec. 5. O(I P × IN ) for standard sampling. We will denote the true p n distribution of pairs from X as ptrue (i ;i ). 2 PROBLEM DEFINITION • Synthetic Pairs : Synthetic pairs (i˜p ;i˜n ) 2 I P × IN are n n ˜p We consider the implicit feedback setting with binary interaction drawn on a per-user basis with i˜ / fG(i˜ j u; X), and i × P X 2 MU MI f g U f g randomly drawn from Xu . The number of synthetic pairs matrix Z2 ; Z2 = 0; 1 given users = u1;:::;uMU , drawn for each user u is in proportion to jX P j. We denote items I = fi1;:::;iMI g. Items I are partitioned apriori into two u ˜p ˜n disjoint sets, I = I P (popular items) [IN (niche/long-tail items) the resulting synthetic pair distribution pθ (i ;i j u), condi- tioned on u and parameters θ of the recommender G. based on their frequency in X. We use the notation Xu to denote the set of items interacted by u 2 U, further split into popular and Discriminative Adversary Training. The adversary D takes P N niche subsets Xu , Xu respectively. as input the synthetically generated item pairs (i˜p ;i˜n ) across all The base neural recommender model G learns a scoring function users, and an equal number of true pairs (ip ;in ) sampled as de- fG(i j u; X);i 2 I;u 2 U to rank items given u’s history Xu and scribed above. It performs two tasks: global feedback X, by minimizing CF objective function O over G • D learns latent representations V = [v ;i 2 I] for the set of recommender G’s parameters θ via stochastic gradient methods.
Details
-
File Typepdf
-
Upload Time-
-
Content LanguagesEnglish
-
Upload UserAnonymous/Not logged-in
-
File Pages4 Page
-
File Size-