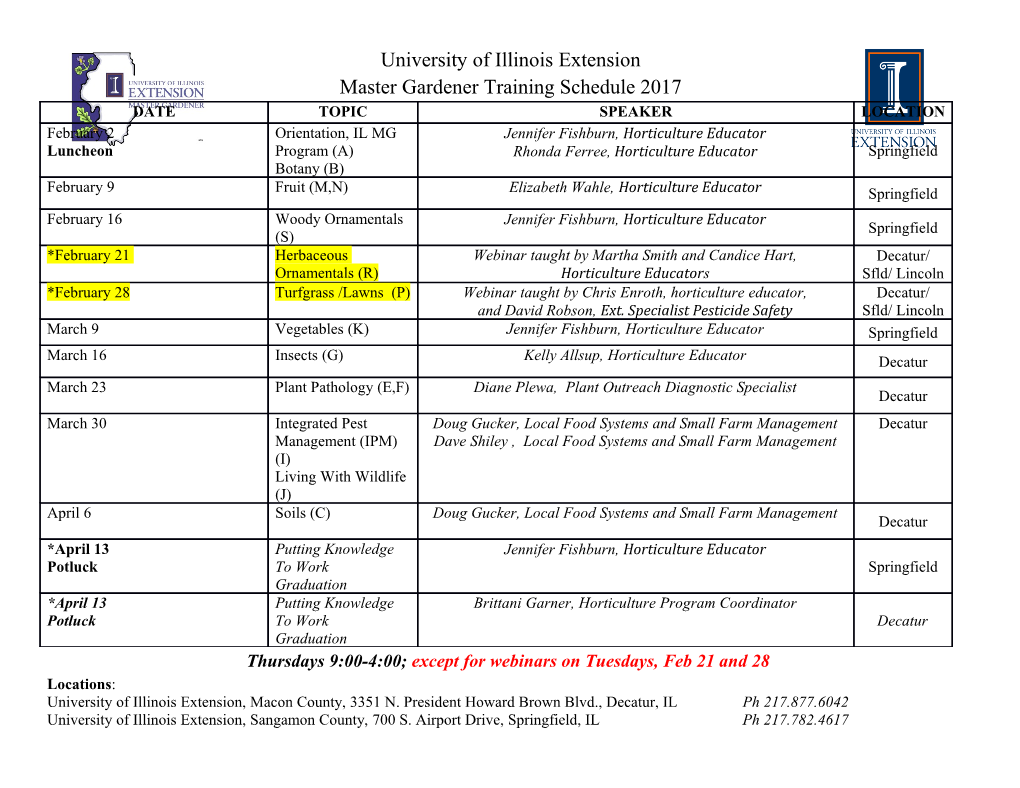
ANALYSIS Identification of significantly mutated regions across cancer types highlights a rich landscape of functional molecular alterations Carlos L Araya1,4, Can Cenik1,4, Jason A Reuter1, Gert Kiss2, Vijay S Pande2, Michael P Snyder1 & William J Greenleaf1,3 Cancer sequencing studies have primarily identified cancer Algorithms to identify cancer driver genes often examine non- driver genes by the accumulation of protein-altering mutations. synonymous-to-synonymous mutation rates across the gene body An improved method would be annotation independent, or recurrently mutated amino acids called mutation hotspots5, as sensitive to unknown distributions of functions within proteins observed in BRAF7, IDH1 (ref. 8) and DNA polymerase ε (encoded and inclusive of noncoding drivers. We employed density-based by POLE)9. Yet, these analyses ignore recurrent alterations in the clustering methods in 21 tumor types to detect variably sized vast intermediate scale of functional coding elements, such as significantly mutated regions (SMRs). SMRs reveal recurrent those affecting protein subunits or interfaces. Moreover, where alterations across a spectrum of coding and noncoding elements, mutation clustering within genes has been examined10–12, analyses including transcription factor binding sites and untranslated have employed windows of fixed length or identified clusters of non­ regions mutated in up to ~15% of specific tumor types. SMRs synonymous mutations, assuming that driver mutations exclusively demonstrate spatial clustering of alterations in molecular influence protein sequence and ignoring the importance of exon- domains and at interfaces, often with associated changes in embedded regulatory elements13–18. signaling. Mutation frequencies in SMRs demonstrate that A significant proportion of regulatory elements in the genome distinct protein regions are differentially mutated across tumor are located proximal to or even in exons15,19, suggesting that many Nature America, Inc. All rights reserved. Inc. Nature America, types, as exemplified by a linker region of PIK3CA in which may be captured by whole-exome sequencing. Efforts to characterize 5 biophysical simulations suggest that mutations affect regulatory noncoding regulatory variation in cancer genomes have primarily interactions. The functional diversity of SMRs underscores both examined either (i) pan-cancer whole-genome sequencing data or © 201 the varied mechanisms of oncogenic misregulation and the (ii) predefined regions—such as ETS-binding sites, splicing signals, advantage of functionally agnostic driver identification. promoters and UTRs—or mutation types20–23. These approaches either presume the relevant targets of disruption or disregard the In cancer, driver mutations alter functional elements of diverse established heterogeneity among cancer types at the level of driver nature and size. For example, melanoma drivers include hyperacti- genes and pathways5,24,25 as well as in nucleotide-specific mutation vating mutations mapping to single amino acid residues (for example, probabilities3,4. Yet, systematic analyses of genomic regulatory activity BRAF Val600; ref. 1), inactivating mutations along tumor-suppressor in animals have identified substantial tissue and developmental stage exons (for example, in PTEN1) and regulatory mutations (for example, specificity26–28, suggesting that mutations in cancer type–specific in the TERT promoter2). Cancer genomics projects such as The regulatory features may be significant noncoding drivers of cancer. Cancer Genome Atlas (TCGA) and the International Cancer Genome To address these diverse limitations, we employed density-based Consortium (ICGC) have substantially expanded our understand- clustering techniques using cancer-, mutation type– and gene- ing of the landscape of somatic alterations by identifying frequently specific mutation models to identify regions of recurrent mutation mutated protein-coding genes3–5. However, these studies have focused in 21 cancer types. This approach permitted the unbiased identifica- little attention on systematically analyzing the positional distribution tion of variably sized genomic regions recurrently altered by somatic of coding mutations or characterizing noncoding alterations6. mutation, which we term significantly mutated regions (SMRs). We identified SMRs in numerous well-established cancer drivers as 1Department of Genetics, Stanford University School of Medicine, Stanford, well as in new genes and functional elements. Moreover, SMRs were California, USA. 2Department of Chemistry, Stanford University, Stanford, associated with noncoding elements, protein structures, molecular California, USA. 3Department of Applied Physics, Stanford University, Stanford, California, USA. 4These authors contributed equally to this work. interfaces, and transcriptional and signaling profiles, thereby provid- Correspondence should be addressed to C.L.A. ([email protected]), ing insight into the molecular consequences of accumulating somatic M.P.S. ([email protected]) or W.J.G. ([email protected]). mutations in these regions. Overall, SMRs identified a rich spectrum Received 17 June; accepted 20 November; published online 21 December of coding and noncoding elements recurrently targeted by somatic 2015; doi:10.1038/ng.3471 alterations that complement gene- and pathway-centric analyses. NATURE GEnETICS ADVANCE ONLINE PUBLICATION 1 ANALYSIS RESULTS composition (GC content) as well as differences in local gene expres- Multiscale detection of SMRs sion and replication timing, which have been shown to correlate We examined ~3 million previously identified5 somatic single-nucleotide with somatic mutation rate4. To avoid skewed mutation probability variants (SNVs) from 4,735 tumors of 21 cancer types, recording29 estimates due to selection pressure on exons, we applied a Bayesian their impact on protein-coding sequences, transcripts and adjacent framework to derive gene-specific mutation probabilities given regulatory regions (Supplementary Fig. 1). We note that 79.0% intronic mutation probabilities in cancer whole-genome sequencing (n = 2,431,360) of these somatic mutations do not alter protein-coding data3,20 while controlling for differences in sensitivity in whole-exome sequences or their splicing and thus were not previously considered and whole-genome sequencing (Online Methods). in the analysis of cancer driver mutations5 (Fig. 1a). Although many known cancer-related genes did not display signals of To discover both coding and noncoding cancer drivers, we applied an high mutation density, increasing density scores correlated with stronger annotation-independent density-based clustering technique30 to iden- enrichments (up to 120×) for somatic SNV–driven cancer genes (n = 158), tify 198,247 variably sized clusters of somatic mutations within exon- as determined by the Cancer Gene Census (CGC; Supplementary proximal domains of the human genome (Fig. 1b and Online Methods). Fig. 3b,c)31,32. Moreover, ~10% of genes associated with SMRs in the We included synonymous mutations because functionally important quintile with the top density scores were not found previously in a gene- noncoding features can be embedded within coding regions13–18. level analysis5 or in the CGC. Thus, high density scores are enriched for Mutation density scores within each identified cluster were derived known cancer genes but also nominate potentially new drivers. as the Fisher’s combined P value of the individual binomial probabilities We applied Monte Carlo simulations to select density score thresh- of observing k or more mutations for each mutation type within the olds controlling the false-discovery rate (FDR) to ≤5% (Supplementary region in each cancer type (Online Methods). We evaluated mutation Fig. 4 and Supplementary Table 1). We identified 872 significantly density for each cluster using gene-specific and genome-wide models mutated regions (SMRs; Fig. 1c) that were altered in ≥2% of patients of mutation probability (Supplementary Fig. 2), which were well in 20 cancer types for further characterization (Fig. 1d). SMRs correlated (Supplementary Fig. 3a), selecting the more conservative spanned 735 genomic regions, which were assigned unique SMR codes estimate for each cluster as the final density score (Online Methods). (for example, TP53.1). Note that some SMRs (n = 120) appeared in Gene-specific mutation probability models accounted for sequence more than one cancer type. a c BRAF 5 kb) DNMT3A KRAS 5 kb) KRAS ≥ ≤ ( ( 5 kb) TBC1D12 NRAS ≤ PTEN ( PIK3CA d 200 Exon* 5′ UTR 500 FLT3 TP53 Intron 3′ UTR EGFR CTNNB1 Splice Downstream Intron Intergenic 6 Nonsynonymous AKT1 IDH2 100 Synonymous UTR Upstream Noncoding exon Other Upstream ′ Downstream UTR Stop gain ′ 5 3 Splice-site region 5 Splice site acceptor IDH1 Start gain 4 Splice site donor 100 20 Start lost Intragenic 10 SMRs Stop lost 3 Synonymous stop (count) 8 ) APC Nonsynonymous start POLE 10 2 CDKN2A BCL2 6 5 4 log Unknown 1 Rare amino acid MYD88, SF3B1 density 2 n = 1 0 P U2AF1 PPP2R1A 1 ( 50 Mutation type FBXW7 0 10 Regions (×1,000) 0 5 10 15 20 25 30 KIAA0907 KIRC CLLX BLCA LAML LUAD LUSC DLBC MELA COLR BRCA PRAD RHAB ESOP UCEC HNSC CARC GLBM OVAR MEDU MUMY (1) Define exon-proximal domains –log YAE1D1 –log10 (density score) Nature America, Inc. All rights reserved. Inc. Nature America, b Exon Exon Exon TMEM208 1 2 3 FBXO24 Exon* 5 Domains 20 n = 191,669 PCDHB8 Other NDUFB9 (2) Discover mutation regions (DBSCAN) Downstream g * * RPS27 3′ UTR 2 * * MALAT1
Details
-
File Typepdf
-
Upload Time-
-
Content LanguagesEnglish
-
Upload UserAnonymous/Not logged-in
-
File Pages12 Page
-
File Size-