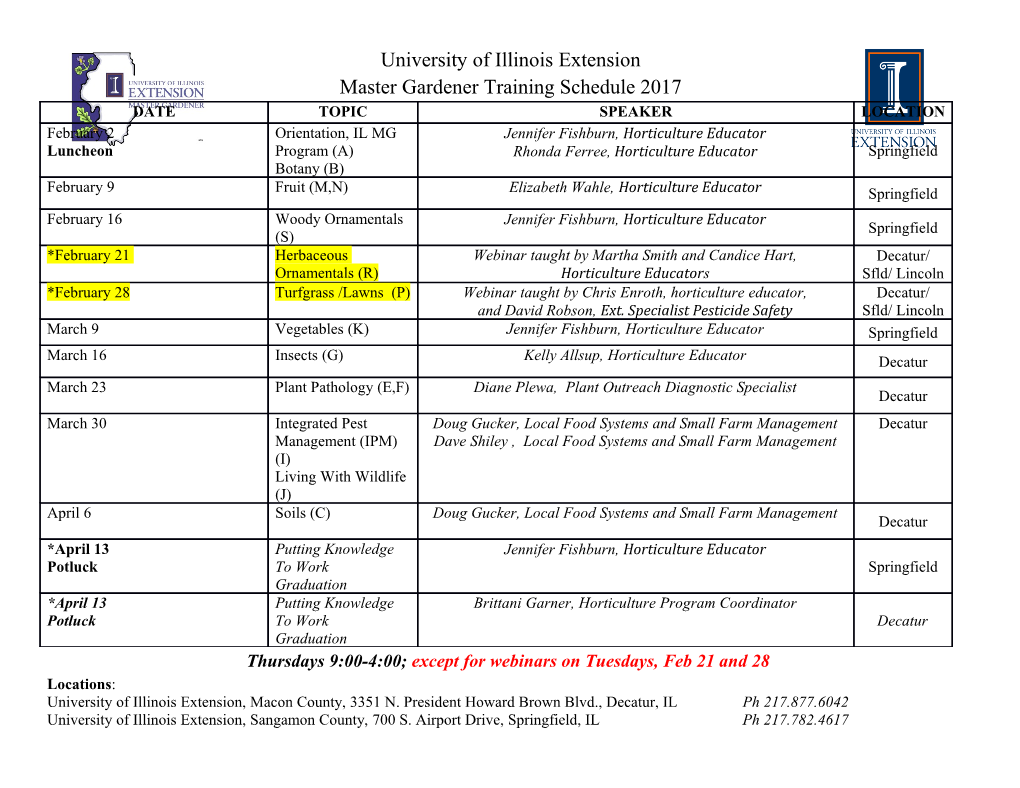
Investigation of a Localized Approach to Shift-Variant Image Restoration and Robust Autofocusing A Dissertation Presented by Younsik Kang to The Graduate School in Partial Fulfillment of the Requirements for the Degree of Doctor of Philosophy in Electrical Engineering Stony Brook University State University of New York at Stony Brook 2011 Copyright by Younsik Kang 2011 Stony Brook University The Graduate School Younsik Kang We, the dissertation committee for the above candidate for the Doctor of Philosophy degree, hereby recommend acceptance of this report. Muralidhara Subbarao, Preliminary Examination Advisor Professor, Department of Electrical & Computer Engineering Sangjin Hong Associate Professor, Department of Electrical & Computer Engineering Monica Fernandez-Bugallo Assistant Professor, Department of Electrical & Computer Engineering Jeffrey Ge Professor, Department of Mechanical Engineering This dissertation is accepted by the Graduate School Dean of the Graduate School iii Stony Brook University The Graduate School Younsik Kang We, the Dissertation committee for the above candidate for the Doctor of Philosophy degree, hereby recommend acceptance of this report. Muralidhara Subbarao, Dissertation Advisor Professor, Department of Electrical & Computer Engineering Sangjin Hong Associate Professor, Department of Electrical & Computer Engineering Monica Fernandez-Bugallo Assistant Professor, Department of Electrical & Computer Engineering Jeffrey Ge Professor, Department of Mechanical Engineering This dissertation is accepted by the Graduate School Lawrence Martin Dean of the Graduate School iv Abstract of the Dissertation Investigation of a Localized Approach to Shift-Variant Image Restoration and Robust Autofocusing by Younsik Kang Doctor of Philosophy in Electrical Engineering Stony Brook University 2011 Images of three-dimensional (3D) scenes or dynamic scenes captured by a digital camera are in general blurred by different degrees at different points in the image. The blur level at a pixel depends on the point spread function (PSF) of the camera near that pixel. The blur may be due to both defocus and motion blur effects. The PSF itself depends on the shape, distance, and motion of objects in the scene as well as imaging parameters of the camera such as focal length, position of image sensor, and camera aperture. Images where the blur PSF changes with spatial position of pixels are said to be shift-variant blurred images. In some special cases such as a planar object perpendicular to the direction of view, the PSF does not change but remains the same at all pixels. In this case the image is said to be a shift-invariant v or convolution blurred image. Image restoration or deblurring is the problem of recovering the focused image from blurred images in the presence of noise. This thesis addresses two closely related problems. One is determining the distance of objects through image defocus analysis for rapid autofocusing of digital cameras. Another is image restoration or recovering the focused image from shift-variant blurred images. Shift-invariant deblurring or deconvolution is a special case of the shift-variant deblurring. Application of this special case to autofocusing of digital cameras is pre- sented in this thesis. In particular, we present a new Robust Depth-from-Defocus (RDFD) method for autofocusing that combines the advantages of both Depth-from- Focus (DFF) and Depth-from-Defocus (DFD). RDFD does not require pixel corre- spondence between samples and determines object depth by analyzing local energy of image. RDFD is shown to be computationally faster and robust in the presence of small image shifts due to camera motion and hand-shake during image capture. A new polynomial model for Focus Measure (FM) is proposed. This new model ap- proximates actual focus measure as a polynomial of a blur parameter σ. This model can be used to predict the variation of focus measure with camera parameters near the focal position where the blur level is small. Many methods have been proposed to deblur or restore shift-variant blurred im- ages. These methods typically suffer from exorbitant computational cost and mem- ory requirement. Matrix approach is a widely used method for shift-variant image restoration. It formulates shift-variant system as a matrix equation and solves it by inverting a large matrix. Both computational cost and noise sensitivity are serious problems. An N ×N image requires inverting an N 2 ×N 2 matrix, which is an O(N 6) vi operation. Therefore computational cost becomes very large even for small images. This method is highly noise sensitive and mere quantiztion noise alone can result in large errors in image restoration. Regularization is needed to reduce noise sensitiv- ity which requires additional computation and complication in the form of selecting optimal regularization technique and parameter. In order to reduce computational cost, some methods use local piecewise shift- invariance approximation in small image blocks to model shift-variant blurring. Each image block is restored separately through deconvolution in the Fourier domain using the Fast Fourier Transform. The resulting restored image blocks are synthesized to reconstruct the whole image. Due to the inherent approximation used, the error in restoration could be large. In addition, this method could result in highly visible blocking artifacts or discontinuities near the boundary between image blocks. This thesis investigates a new approach to shift-variant image restoration based on a complete localization of the blurring process using Rao Transform (RT). This approach is fully localized, non-iterative, and effective. It assumes a local polynomial model for the focused image, e.g. a bicubic model in a small image region such as 9 × 9. The blurred image intensity at each pixel is expressed in terms of the deriva- tives of the focused image at the same pixel and moments of the derivatives of the shift-variant PSF at the same pixel. This differential equation is inverted locally by considering the spatial derivatives of the blurred image. The resulting solution pro- vides a computationally efficient, parallel, and closed-form solution to the shift-variant image restoration problem. This method is extended by incorporating regularization techniques to derive robust image restoration techniques. In comparison with com- vii peting techniques, this new method is fully localized and it involves non-iterative computation in the spatial domain. It enables deblurring to be carried out in the im- age space and to restrict computations to use local image data. Therefore, this new method permits very fine-grain parallel implementation and is suitable for real-time applications. In this thesis, the RT theory underlying the image restoration method is reviewed, tested, and evaluated in the case of shift-variant blur. The performance of RT for var- ious shift-variant systems including one dimensional blur, two dimensional blur, and multiple blur are investigated. Analysis of noise sensitivity of spatial differentiation filters is presented. A unified expression is derived for multiple blur that includes mo- tion blur and shift-variant defocus. Results of both simulation and real experiments are presented for digital still cameras and cell-phone cameras. Performance of the new localized approach is compared with matrix approaches such as Singular Value Decomposition (SVD) and Conjugate Gradient method. The results indicate that the new approach offers significant computational speed up and improved accuracy in comparison with general Singular Value Decomposition (SVD) and Conjugate Gradient method. In particular, when combined with regularization, the new approach provides computationally efficient and more accurate results. viii Contents List of Figures xii List of Tables xxi 1 Introduction 1 1.1 Literature Review . 3 1.1.1 Autofocusing Techniques . 3 1.1.2 Inverse Filtering of Shift-Variant Blur Kernels . 8 1.2 Dissertation Organization . 12 2 Robust Depth-from-Defocus 15 2.1 Introduction . 16 2.2 Camera Model . 18 2.2.1 Image formation . 19 2.3 S-Transform . 22 2.4 Robust Depth from Defocus (RDFD) . 24 2.4.1 RDFD based on Green's Identity . 28 2.4.2 Experiments . 30 2.5 Two Variations of DFD: FET and BET . 34 2.6 Relation between Depth from Focus (DFF) and the Blur Parameter σ of Depth from Defocus (DFD) . 35 ix 2.7 Summary . 38 3 Restoration of One-Dimensional Shift-Variant Blur 41 3.1 Problem of Modeling of Shift Variant System . 42 3.1.1 Matrix Form of RT for One-Dimension . 46 3.1.2 Example . 48 3.2 Advantage of RT . 50 3.3 Noise Sensitivity Analysis of Inverse RT Filter . 51 3.4 Experiment . 57 3.4.1 Restoration of Sinusoidal Pattern . 58 3.4.2 Experiment on Barcode Image with Gaussian SV-PSF . 61 3.5 Summary . 64 4 Shift-Variant Image Deblurring: Two-dimensional Case 65 4.1 Brief Review of Prior Art . 66 4.2 Theory of RT for Two-dimension . 68 4.2.1 Example . 74 4.2.2 Computational Complexity . 75 4.3 Experiments . 76 4.3.1 Simulation Results . 76 4.3.2 Results on Real Images . 80 4.3.3 Experiment on a Cylindrical Object . 84 4.3.4 Experiment on Spatial Differential windows derived by Polyno- mial Fitting . 89 4.4 Summary . 94 5 Motion and Defocus Blur 95 5.0.1 Shift-Invariant Motion Blur . 95 5.0.2 Experiments on Shift-invariant Motion Deblur . 98 5.0.3 Multiple Blur Model . 103 5.0.4 Experiments on Multiple Blur Model . 106 6 Comparison of Matrix Approaches and RT for Image Deblurring 110 6.1 Introduction . 110 6.2 Matrix approach to shift-variant imaging system . 111 6.2.1 Matrix formation . 111 6.3 Kernel Matrix Inversion . 113 6.3.1 Singular Value Decomposition . 113 6.3.2 Conjugate Gradient Method . 116 6.3.3 Complexity of SVD . 117 6.4 Image Restoration based on RT and SVD . 118 6.5 Image Restoration based on Conjugate Gradient Method . 124 6.6 Regularization techniques . 125 6.6.1 Regularization of SVD .
Details
-
File Typepdf
-
Upload Time-
-
Content LanguagesEnglish
-
Upload UserAnonymous/Not logged-in
-
File Pages181 Page
-
File Size-