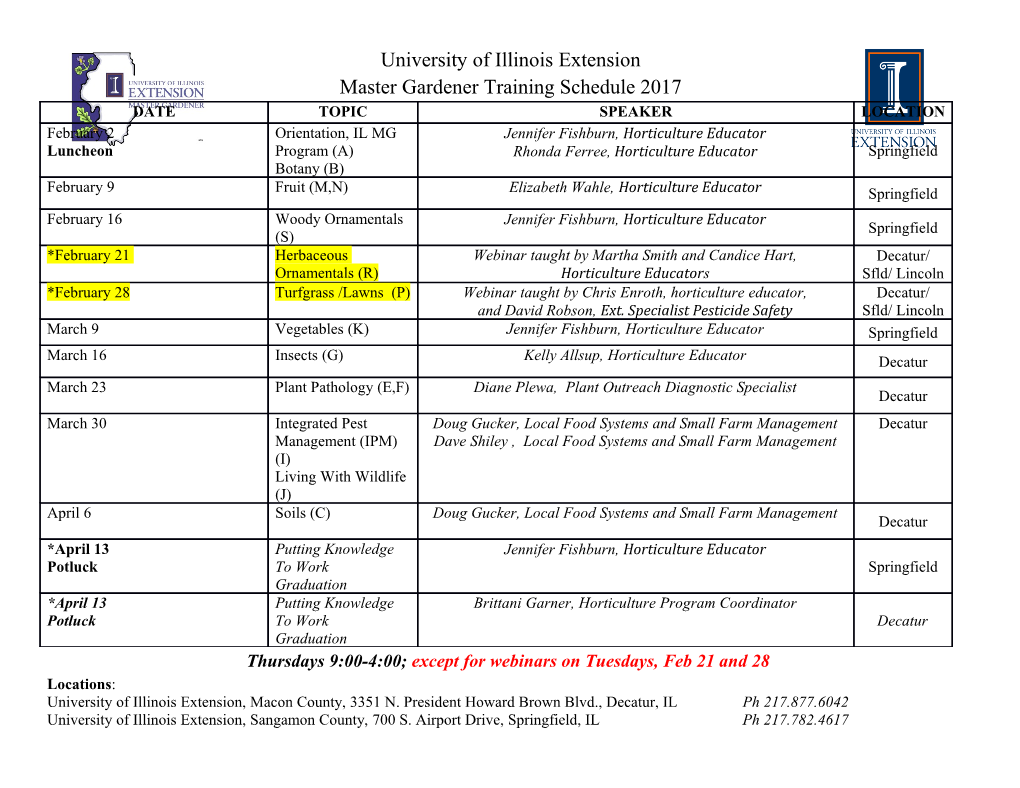
Florida State University Libraries Electronic Theses, Treatises and Dissertations The Graduate School 2013 Effects of Time-off on Performance in the National Football League Jeremy J. Foreman Follow this and additional works at the FSU Digital Library. For more information, please contact [email protected] THE FLORIDA STATE UNIVERSITY COLLEGE OF EDUCATION EFFECTS OF TIME-OFF ON PERFORMANCE IN THE NATIONAL FOOTBALL LEAGUE By JEREMY J. FOREMAN A Thesis submitted to the Department of Sport Management in partial fulfillment of the requirements for the degree of Master of Science Degree Awarded: Summer Semester, 2013 Jeremy J. Foreman defended this thesis on June 17, 2013. The members of the supervisory committee were: Ryan Rodenberg Professor Directing Thesis Joshua Newman Committee Member Yu Kyoum Kim Committee Member The Graduate School has verified and approved the above-named committee members, and certifies that the thesis has been approved in accordance with university requirements. ii TABLE OF CONTENTS List of Tables v List of Figures vii Abstract x CHAPTER ONE: INTRODUCTION 1 Scheduling Background 1 Controversy Surrounding Schedules 2 Brief History of NFL Bye Weeks 5 Purpose of Study 7 Significance of Study 7 Limitations 10 Layout of Thesis 11 CHAPTER TWO: LITERATURE REVIEW 12 Analysts’ Perceptions of Bye Weeks and Team Performance 13 Learning as a Function of Time 16 Respite as a Function of Time 19 Physiology, Performance, and Time 26 Summary of Previous Research Methods and Findings 30 CHAPTER THREE: METHODOLOGY 33 Model One: Bye Week Impact 34 Model Two: Time-Off Impact, Ceteris Paribus 35 Dichotomizing Model Two 37 Model Three: Optimal Bye Week for Uninterrupted Final Stretch 39 CHAPTER FOUR: RESULTS 42 Analysis of Model One: Bye Week Impact 42 Analysis of Model Two: Time-Off Impact, Ceteris Paribus 43 Analysis of Dichotomized Model Two 48 Analysis of Model Three: Optimal Bye Week for Uninterrupted Final Stretch 61 CHAPTER FIVE: DISCUSSION 66 Conclusions 66 Implications 75 Limitations 77 Future Research 80 iii APPENDICES 85 A MODEL TWO 85 B REVISED MODEL TWO 87 C MODEL TWO FOR YOUNGER TEAMS 89 D MODEL TWO FOR OLDER TEAMS 91 E REVISED MODEL TWO FOR YOUNGER TEAMS 93 F REVISED MODEL TWO FOR OLDER TEAMS 95 G MODEL TWO FOR GOOD TEAMS 97 H MODEL TWO FOR BAD TEAMS 99 I REVISED MODEL TWO FOR GOOD TEAMS 101 J REVISED MODEL TWO FOR BAD TEAMS 103 K MODEL TWO FOR DIVISION GAMES 105 L MODEL TWO FOR NON-DIVISION GAMES 107 M REVISED MODEL TWO FOR DIVISION GAMES 109 N REVISED MODEL TWO FOR NON-DIVISION GAMES 111 O MODEL THREE 113 REFERENCES 115 BIOGRAPHICAL SKETCH 123 iv LIST OF TABLES 4.1 Regression output for Model Two with DAYDIFSQ 45 4.2 Regression output for Model Two 45 4.3 Regression output for revised Model Two 46 4.4 Regression output for Model Two with younger teams 48 4.5 Regression output for revised Model Two with younger teams 49 4.6 Regression output for Model Two with older teams 50 4.7 Regression output for revised Model Two with older teams 51 4.8 Regression output for Model Two with good teams 52 4.9 Regression output for revised Model Two with good teams 52 4.10 Regression output for Model Two with bad teams 53 4.11 Regression output for revised Model Two with bad teams 54 4.12 Regression output for Model Two with division teams 55 4.13 Regression output for Model Two with non-division teams 56 4.14 Regression output for revised Model Two with division teams 56 4.15 Regression output for revised Model Two with non-division teams 57 4.16 Model Two with sample from Method One 61 4.17 Model Three regression output 62 4.18 Model Three multicollinearity output 63 A.1 Multicollinearity output for Model Two 86 B.1 Multicollinearity output for revised Model Two 88 C.1 Multicollinearity output for Model Two with only younger teams 90 v D.1 Multicollinearity output for Model Two with only older teams 92 E.1 Multicollinearity output for revised Model Two with younger teams 94 F.1 Multicollinearity output for revised Model Two with older teams 96 G.1 Multicollinearity output for Model Two with only good teams 98 H.1 Multicollinearity output for Model Two with only bad teams 100 I.1 Multicollinearity output for revised Model Two with good teams 102 J.1 Multicollinearity output for revised Model Two with bad teams 104 K.1 Multicollinearity output for Model Two with only division games 106 L.1 Multicollinearity output for Model Two with only non-division games 108 M.1 Multicollinearity output for revised Model Two with division games 110 N.1 Multicollinearity output for revised Model Two with non-division games 112 vi LIST OF FIGURES 4.1 Linearity of BYESQUAR 64 4.2 Linearity of BYESQUAR with trendlines 65 5.1 Sum of coefficients by bye week 73 A.1 Normality of residuals for Model Two 85 A.2 Homoscedasticity of residuals for Model Two 85 A.3 Linearity of regressors for Model Two 86 B.1 Normality of residuals for revised Model Two 87 B.2 Homoscedasticity of residuals for revised Model Two 87 B.3 Linearity of regressors for revised Model Two 88 C.1 Normality of residuals for Model Two with only younger teams 89 C.2 Homoscedasticity of residuals for Model Two with only younger teams 89 C.3 Linearity of regressors for Model Two with only younger teams 90 D.1 Normality of residuals for Model Two with only older teams 91 D.2 Homoscedasticity of residuals for Model Two with only older teams 91 D.3 Linearity of regressors for Model Two with only older teams 92 E.1 Normality of residuals for revised Model Two with younger teams 93 E.2 Homoscedasticity of residuals for revised Model Two with younger teams 93 E.3 Linearity of regressors for revised Model Two with younger teams 94 F.1 Normality of residuals for revised Model Two with older teams 95 F.2 Homoscedasticity of residuals for revised Model Two with older teams 95 F.3 Linearity of regressors for revised Model Two with older teams 96 G.1 Normality of residuals for Model Two with only good teams 97 vii G.2 Homoscedasticity of residuals for Model Two with only good teams 97 G.3 Linearity of regressors for Model Two with only good teams 98 H.1 Normality of residuals for Model Two with only bad teams 99 H.2 Homoscedasticity of residuals for Model Two with only bad teams 99 H.3 Linearity of regressors for Model Two with only bad teams 100 I.1 Normality of residuals for revised Model Two with good teams 101 I.2 Homoscedasticity of residuals for revised Model Two with good teams 101 I.3 Linearity of regressors for revised Model Two with good teams 102 J.1 Normality of residuals for revised Model Two with bad teams 103 J.2 Homoscedasticity of residuals for revised Model Two with bad teams 103 J.3 Linearity of regressors for revised Model Two with bad teams 104 K.1 Normality of residuals for Model Two with only division games 105 K.2 Homoscedasticity of residuals for Model Two with only division games 105 K.3 Linearity of regressors for Model Two with only division games 106 L.1 Normality of residuals for Model Two with only non-division games 107 L.2 Homoscedasticity of residuals for Model Two with only non-division games 107 L.3 Linearity of regressors for Model Two with only non-division games 108 M.1 Normality of residuals for revised Model Two with division games 109 M.2 Homoscedasticity of residuals for revised Model Two of division games 109 M.3 Linearity of regressors for revised Model Two with division games 110 N.1 Normality of residuals for revised Model Two with non-division games 111 N.2 Homoscedasticity of residuals for revised Model Two with non-division games 111 N.3 Linearity of regressors for revised Model Two with non-division games 112 O.1 Model Three normality of residuals 113 O.2 Model Three homoscedasticity of residuals 113 viii O.3 Model Three linearity of regressors 114 ix ABSTRACT Among National Football League (NFL) fans, coaches, and analysts, there are many different views on how time-off effects team performance. Differences in how time-off is allocated to teams has also become a source of controversy as debates continue regarding which teams received the least favorable schedules. This investigation was concerned with identifying how time-off prior to a game affects the final score, when is the best time to have a bye week, and why some teams may benefit more from time-off than other teams. Twenty-one seasons were examined using ordinary least squares regressions to determine that there is at least about a .21 point advantage for each extra day of preparation time that a team receives prior to a game, relative to the time received by an opponent. This advantage is increased for older teams, less talented teams, and teams that are less familiar with their opponents. Unfamiliar opponents benefit from an additional day to prepare by about .38 points which accounts for approximately 2.6 points for a standard seven day bye week. Additionally, about 54.9 percent of teams defeat their opponents when coming off of a bye week by an average margin of victory of about 12.3 points compared to the approximately 8.7 points that non-bye week teams defeated bye week teams by on average.
Details
-
File Typepdf
-
Upload Time-
-
Content LanguagesEnglish
-
Upload UserAnonymous/Not logged-in
-
File Pages134 Page
-
File Size-