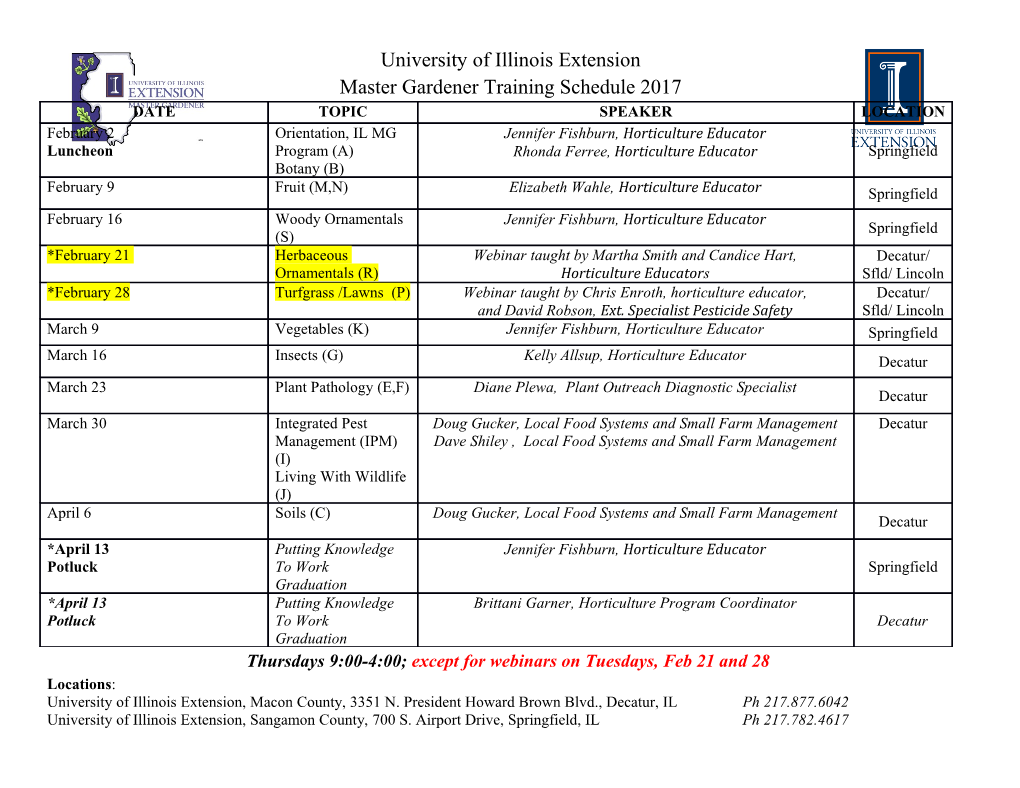
A Statistical View of Deep Learning Part 2 Jennifer Hoeting, Colorado State University A Statistical View of Deep Learning in Ecology Part 1: Introduction I Introduction to machine learning I Introduction to deep learning Part 2: Going deeper I Neural networks from 3 viewpoints I Mathematics of deep learning I Model fitting I Types of deep learning models Part 3: Deep learning in practice I Ethics in deep learning I Deep learning in ecology A Statistical View of Deep Learning Part 2 | Jennifer Hoeting, Colorado State University 202 / 276 Neural networks from three viewpoints Neural network: algorithm which allows a computer to learn from data Deep learning: multi-layer neural network A Statistical View of Deep Learning Part 2 | Jennifer Hoeting, Colorado State University 203 / 276 Neural networks: An Introduction from multiple viewpoints A neural network I as a Black Box I as a Statistical Model I in Deep Learning A Statistical View of Deep Learning Part 2 | Jennifer Hoeting, Colorado State University 204 / 276 Neural Network as a black box algorithm Image source: www.learnopencv.com A Statistical View of Deep Learning Part 2 | Jennifer Hoeting, Colorado State University 205 / 276 Neural Network as a statistical model Goal: extract linear combinations of the inputs as derived features, and then model the target as a nonlinear function of these features Translated to statistics: I fit a nonlinear model to the response and predictors I predictors are transformed using multivariate techniques A neural network is a nonlinear model A Statistical View of Deep Learning Part 2 | Jennifer Hoeting, Colorado State University 206 / 276 Neural Network as a statistical model A Statistical View of Deep Learning Part 2 | Jennifer Hoeting, Colorado State University 207 / 276 Image source Statistics versus neural network terminology Statistics Neural Networks model network, graph fitting learning, training coefficient, parameter weight predictor, variable input, feature predicted response output observation exemplar parameter estimation training or learning steepest descent back-propagation intercept bias term derived predictor hidden node penalty function weight decay A Statistical View of Deep Learning Part 2 | Jennifer Hoeting, Colorado State University 208 / 276 Neural networks in deep learning A Statistical View of Deep Learning Part 2 | Jennifer Hoeting, Colorado State University 209 / 276 Some mathematics of neural networks A Statistical View of Deep Learning Part 2 | Jennifer Hoeting, Colorado State University 210 / 276 Opening the black box of deep learning A Statistical View of Deep Learning Part 2 | Jennifer Hoeting, Colorado State University 211 / 276 Overview Key idea: Neural networks are merely regression models with transformed predictors Consider the following progression of models: I Regression model I Nonparametric regression model I Neural network model I Deep learning model A Statistical View of Deep Learning Part 2 | Jennifer Hoeting, Colorado State University 212 / 276 Regression model 6 5 4 y 3 2 1 0.00 0.25 0.50 0.75 1.00 x A Statistical View of Deep Learning Part 2 | Jennifer Hoeting, Colorado State University 213 / 276 Regression model Model 2 y = β0 + β1x + where ∼ N(0, σ ) = f (x) Fitted model yb = βb0 + βb1x = fb(x) Loss function n X 2 (yi − ybi ) i=1 A Statistical View of Deep Learning Part 2 | Jennifer Hoeting, Colorado State University 214 / 276 Regression model 1.0 0.5 y 0.0 −0.5 −1.0 0.25 0.50 0.75 1.00 x A Statistical View of Deep Learning Part 2 | Jennifer Hoeting, Colorado State University 215 / 276 Nonparametric regression K X yb = fb(x) = βb0 + βbk σbk (x) k=1 | {z } function of x Examples 1. Polynomial regression k 2 K σk (x) = x so fb(x) = βb0 + βb1x + βb2x + ··· + βbK x 2. Regression splines I A spline is a function that is constructed piece-wise from polynomial functions. I Apply a family of transformations to x, σ1(x), σ2(x), . , σK (x) A Statistical View of Deep Learning Part 2 | Jennifer Hoeting, Colorado State University 216 / 276 Nonparametric regression A spline of degree K is a function formed by connecting polynomial segments of degree K so that: I the function is continuous, I the function has K − 1 continuous derivatives, and I the Kth derivative is constant between knots. A Statistical View of Deep Learning Part 2 | Jennifer Hoeting, Colorado State University 217 / 276 Nonparametric regression Simple spline regression Moving window: yb is the average of the y’s for nearby x values or a weighted average (kernel smoothing) 1.0 0.5 y 0.0 −0.5 moving window −1.0 0.25 0.50 0.75 1.00 x A Statistical View of Deep Learning Part 2 | Jennifer Hoeting, Colorado State University 218 / 276 Nonparametric regression Basis functions: every continuous function can be represented as a linear combination of basis functions. More advanced spline functions: basis splines B−Splines Basis functions 0.6 0.5 0.4 0.3 0.2 0.1 0.0 0.2 0.4 0.6 0.8 1.0 x A Statistical View of Deep Learning Part 2 | Jennifer Hoeting, Colorado State University 219 / 276 Nonparametric regression 1.0 0.5 y 0.0 −0.5 −1.0 0.25 0.50 0.75 1.00 x key df = 100 df = 3 A Statistical View of Deep Learning Part 2 | Jennifer Hoeting, Colorado State University 220 / 276 Nonparametric regression 1.0 0.5 y 0.0 −0.5 −1.0 0.25 0.50 0.75 1.00 x A Statistical View of Deep Learning Part 2 | Jennifer Hoeting, Colorado State University 221 / 276 Nonparametric regression The weaknesses of this approach are: I The basis is fixed and independent of the data I If there are many predictors, this approach doesn’t work well I If the basis doesn’t ‘agree’ with true f , then K will have to be large to capture the structure I What if parts of f have substantially different structure? Alternative approach: the data tell us what kind of basis to use A Statistical View of Deep Learning Part 2 | Jennifer Hoeting, Colorado State University 222 / 276 Neural network model Key idea: think of a neural network as a multiple regression model with transformed predictors Example: One-layer neural network model with K hidden nodes σ1(x), σ2(x), . , σK (x) and 3 predictors (x1, x2, x3) y =β0 + β1 σ1(α10 + α11x1 + α12x2 + α13x3) + β2 σ2(α20 + α21x1 + α22x2 + α23x3) + ··· + βK σK (αK0 + αK1x1 + αK2x2 + αK3x3) | {z } function of x A Statistical View of Deep Learning Part 2 | Jennifer Hoeting, Colorado State University 223 / 276 Deep Learning Model Neural network model K X y = β0 + βk σk (αk0 + αk1x1 + αk2x2 + αk3x3) k=1 Two-layer “deep” learning model K X yb = fb(x) = βb0 + βbk σk (αk0+αk1x1 + αk2x2 + αk3x3)) k=1 ↑ ↑ ↑ Replace each of these predictors with another neural network model A Statistical View of Deep Learning Part 2 | Jennifer Hoeting, Colorado State University 224 / 276 Illustration of a deep learning model Image: Deep Learning, Fig 1.2 A Statistical View of Deep Learning Part 2 | Jennifer Hoeting, Colorado State University 225 / 276 Image source: Fig 1.2, Deep Learning A Statistical View of Deep Learning in Ecology Part 1: Introduction I Introduction to machine learning I Introduction to deep learning Part 2: Going deeper I Neural networks from 3 viewpoints I Mathematics of deep learning I Model fitting I Types of deep learning models Part 3: Deep learning in practice I Ethics in deep learning I Deep learning in ecology A Statistical View of Deep Learning Part 2 | Jennifer Hoeting, Colorado State University 226 / 276 Deep Learning: Model fitting A Statistical View of Deep Learning Part 2 | Jennifer Hoeting, Colorado State University 227 / 276 Deep Learning Software I Python • Most popular language for deep learning • Object-oriented, high-level programming language • Main packages: TensorFlow, PyTorch, Keras, . • Do you need to learn Python? Maybe I Keras • Open-source neural-network library written in Python. • Keras package is an API. • API (application programming interface) allows multiple software packages to interact. • Keras can interact with: TensorFlow, Microsoft Cognitive Toolkit, R, Theano, PlaidML A Statistical View of Deep Learning Part 2 | Jennifer Hoeting, Colorado State University 228 / 276 Deep learning in R Neural network models in the Caret package 1. Neural network models: nnet, mxnet, mxnetAdam, neuralnet, and more 2. Stacked AutoEncoder Deep Neural Network 3. Many other options R packages: keras and kerasR I Interface to the Python deep learning package Keras I Rstudio’s keras pages I Can be buggy when any of the packages it accesses are updated A Statistical View of Deep Learning Part 2 | Jennifer Hoeting, Colorado State University 229 / 276 Deep Learning: Model fitting Steps in model fitting 1. Define network structure 2. Select loss function 3. Select optimizer A Statistical View of Deep Learning Part 2 | Jennifer Hoeting, Colorado State University 230 / 276 Network structure I A layer consists of a set of nodes I In a fully-connected model, each node on one layer connects to all nodes in the next layer Image source: towardsdatascience A Statistical View of Deep Learning Part 2 | Jennifer Hoeting, Colorado State University 231 / 276 Network structure Defining the network model in the R interface to Keras model <- keras_model_sequential() model %>% layer_dense(units = 256, activation = 'relu') %>% layer_dense(units = 128, activation = 'relu') %>% layer_dense(units = 10, activation = 'softmax') keras.rstudio.com A Statistical View of Deep Learning Part 2 | Jennifer Hoeting, Colorado State University 232 / 276 Network structure Recall the basic neural network model (one layer, p predictors) K X y = β0+ βk σk (α0k + α1k x1 + α2k x2 + ··· + α3pxp) x k=1 Activation
Details
-
File Typepdf
-
Upload Time-
-
Content LanguagesEnglish
-
Upload UserAnonymous/Not logged-in
-
File Pages76 Page
-
File Size-