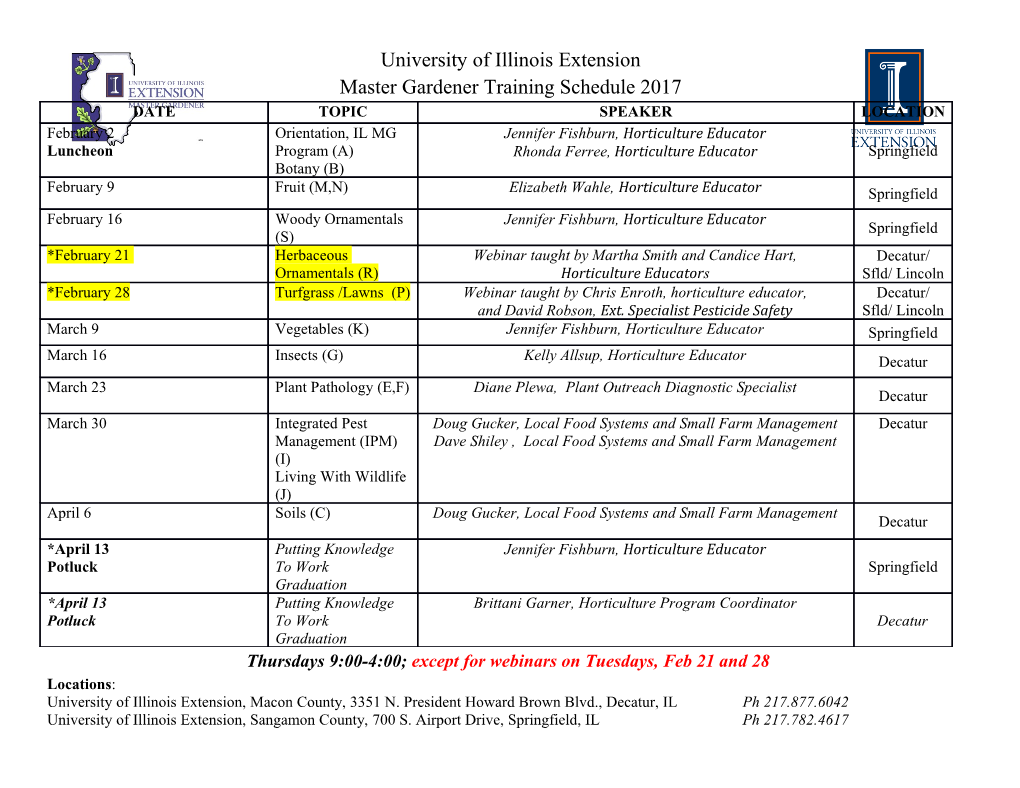
Statistics 910, #8 1 Introduction to ARMA Models Overview 1. Modeling paradigm 2. Review stationary linear processes 3. ARMA processes 4. Stationarity of ARMA processes 5. Identifiability of ARMA processes 6. Invertibility of ARMA processes 7. ARIMA processes Modeling paradigm Modeling objective A common measure used to assess many statistical models is their ability to reduce the input data to random noise. For example, we often say that a regression model “fits well" if its residuals ideally resemble iid random noise. We often settle for uncorrelated processes with data. Filters and noise Model the observed time series as the output of an un- known process (or model) M \driven by" an input sequence composed iid 2 of independent random errors ftg ∼ Dist(0; σ ) (not necessarily nor- mal), t ! Process M ! Xt From observing the output, say X1;:::;Xn, the modeling task is to characterize the process (and often predict its course). This \signal processing" may be more appealing in the context of, say, underwater acoustics rather than macroeconomic processes. Prediction rationale If a model reduces the data to iid noise, then the model captures all of the relevant structure, at least in the sense that Statistics 910, #8 2 we obtain the decomposition ^ Xt = E (XtjXt−1; :::) + t = Xt + t : Causal, one-sided Our notions of time and causation imply that the cur- rent value of the process cannot depend upon the future (nonantici- pating), allowing us to express the process M as Xt = M(t; t−1;:::) : Volterra expansion is a general (too general?) expansion (like the infinite Taylor series expansion of a function) that expresses the process in terms of prior values of the driving input noise. Differentiating M with respect to each of its arguments, we obtain the one-sided expansion (Wiener 1958), 1 X X X Xt − µ = jt−j + jkt−jt−k + jkmt−jt−kt−m + ··· ; j=0 j;k j;k;m @2M where, for example, jk = evaluated at zero. The first @t−j @t−k summand on the right gives the linear expansion. Linearity The resulting process is linear if Xt is a linear combination (weighed sum) of the inputs, 1 X Xt = jt−j (1) j=0 Other processes are nonlinear. The process is also said to be causal (Defn 3.7) if there exists an white noise sequence t and an absolutely summable sequence (or sometimes an `2 sequence) f jg such that (1) holds. The key notion of causality is that the current observation is a function of current and past white noise terms (analogous to a random variable that is adapted to a filtration). Invertibility The linear representation (1) suggests a big problem for iden- tifying and then estimating the process: it resembles a regression in which all of the explanatory variables are functions of the unobserved Statistics 910, #8 3 errors. The invertibility condition implies that we can also express the errors as weighted sum of current and prior observations, 1 X t = πjXt−j: j=0 Thinking toward prediction, we will want to have an equivalence of the form (for any r.v. Y ) E [Y jXt;Xt−1;:::] = E [Y jt; t−1;:::] This equivalence implies that information in the current and past er- rors is equivalent to information in the current and past data (i.e., the two sigma fields generated by the sequences are equivalent). Notice that the conditioning here relies on the infinite collection of prior val- ues, not a finite collection back to some fixed point in time, such as t = 1. Implication and questions The initial goal of time series modeling using the class of ARMA models to be defined next amounts to finding a par- simonious, linear model which can reduce fXtg to iid noise. Questions remain about how to do this: 1. Do such infinite sums of random variables exist, and how are they to be manipulated? 2. What types of stationary processes can this approach capture (i.e., which covariance functions)? 3. Can one express these models using few parameters? Review: Stationary Linear Processes Notation of S&S uses fwtg as the canonical mean zero, finite variance white-noise process (which is not necessarily normally distributed), 2 wt ∼ WN(0; σ ) P Convergence. For the linear process defined as Xt = j jwt−j to exist, we need assumptions on the weights j. An infinite sum is a limit, 1 n X X jwt−j = lim jwt−j; n j=0 j=0 Statistics 910, #8 4 and limits require a notion of convergence (how else do you decide if you are close)? Modes of convergence for r.v.s include: • Almost sure, almost everywhere, with probability one, w.p. 1, a:s: Xn ! X, P f! : lim Xn = Xg = 1: P • In probability, Xn ! X, lim P f! : jXn − Xj > g = 0 n • In mean square or `2, the variance goes to zero: 2 E (Xn − X) ! 0: `2 convergence of linear process requires that X 2 j < 1 or f jg 2 `2: j Consequently X X 2 j j+k ≤ j < 1: j In general, if f jg 2 `2 and fXtg is stationary, then the linear filter 1 X Yt = jXt−j j=0 defines a stationary process with covariance function X Cov(Yt+h;Yt) = γY (h) = j kγX (h − j + k): j;k P P 2 Informally, Var(Yt) = j;k j kγ(k − j) ≤ γ(0) j j . Covariances When the \input" is white noise, then the covariances are infinite sums of the coefficients of the white noise, 1 2 X γY (h) = σX j j+h: (2) j=0 Statistics 910, #8 5 P Absolutely summable S&S often assume that j jj < 1 (absolutely summable). This is a stronger assumption that simplifies proofs of 1 a.s. convergence. For example, j is not absolutely summable, but is square summable. We will not be too concerned with a.s. convergence and will focus on mean-square convergence. (The issue is moot for ARMA processes.) Question Does the collection of linear processes as given define a vector space that allows operations like addition? The answer is yes, using the concept of a Hilbert space of random variables. Statistics 910, #8 6 ARMA Processes Conflicting goals Obtain models that possess a wide range of covariance functions (2) and that characterize j as functions of a few parameters that are reasonably easy to estimate. We have seen several of these parsimonious models previously, e.g., • Finite moving averages: j = 0; j > q > 0. j • First-order autoregression: j = φ ; jφj < 1. ARMA processes also arise when sampling a continuous time solution to a stochastic differential equation. (The sampled solution to a pth degree SDE is an ARMA(p,p − 1) process.) Definition 3.5 The process fXtg is an ARMA(p,q) process if 1. It is stationary. 2. It (or the deviations Xt − EXt) satisfies the linear difference equation written in \regression form" (as in S&S, with negative signs attached to the φs) as Xt − φ1Xt−1 − · · · − φpXt−p = wt + θ1wt−1 + ··· + θqwt−q (3) 2 where wt ∼ WN(0; σ ). Backshift operator Abbreviate the equation (3) using the so-called back- k shift operator defined as B Xt = Xt−k. Using B, write (3) as φ(B)Xt = θ(B)wt where the polynomials are (note the differences in signs) p φ(z) = 1 − φ1z − · · · − φpz and q θ(z) = 1 + θ1z + ··· + θqz Closure The backshift operator shifts the stochastic process in time. Be- cause the process is stationary (having a distribution that is invariant of the time indices), this transformation maps a stationary process Statistics 910, #8 7 into another stationary process. Similarly, scalar multiplication and finite summation preserve stationarity; that is, the vector space of sta- tionary processes is closed in the sense that if Xt is stationary, then P 2 so too is Θ(B) Xt so long as j θj < 1. Aside: Shifts elsewhere in math The notion of shifting a stationary process f:::;Xt;Xt+1;::: to f:::;Xt−1;Xt;::: has parallels. For ex- ample, suppose that p(x) is a polynomial. Define the operator S on the space of polynomials defined as S p(x) = p(x − 1). If the space of polynomials is finite dimensional (up to degree m), then we can write 3 m S = I + D + D2=2 + D =3! + ··· D =m! where I is the identity (I p = p) and D is the differntiation operator. (The proof is a direct application of Taylor's theorem.) Value of backshift notation 1. Compact way to write difference equations and avoid backsub- stitution, Backsubstitution becomes the conversion of 1=(1 − x) into a geometric series; If we manipulate B algebraically in the conversion of the AR(1) to moving average form, we obtain the same geometric representation without explicitly doing the te- dious backsubstitution: w φ(B)X = w ) X = t t t t 1 − φB 2 2 = (1 + φB + φ B + :::)wt 2 = wt + φwt−1 + φ wt−2 + :::: (4) Which clearly requires (as in a geometric series) that jφj < 1. 2. Expression of constraints that assure stationarity and identifia- bility. 3. Express effects of operations on a process: • Adding uncorrelated observation random noise to an AR pro- cess produces an ARMA process. • A weighted mixture of lags of an AR(p) model is ARMA. Statistics 910, #8 8 Consider the claim that an average of several lags of an autoregression forms an ARMA process. Backshift polynomials make it trival to show this claim holds: θ(B) φ(B)X = w ) θ(B)X = w ; t t t φ(B) t which has the rational form of an ARMA process.
Details
-
File Typepdf
-
Upload Time-
-
Content LanguagesEnglish
-
Upload UserAnonymous/Not logged-in
-
File Pages15 Page
-
File Size-