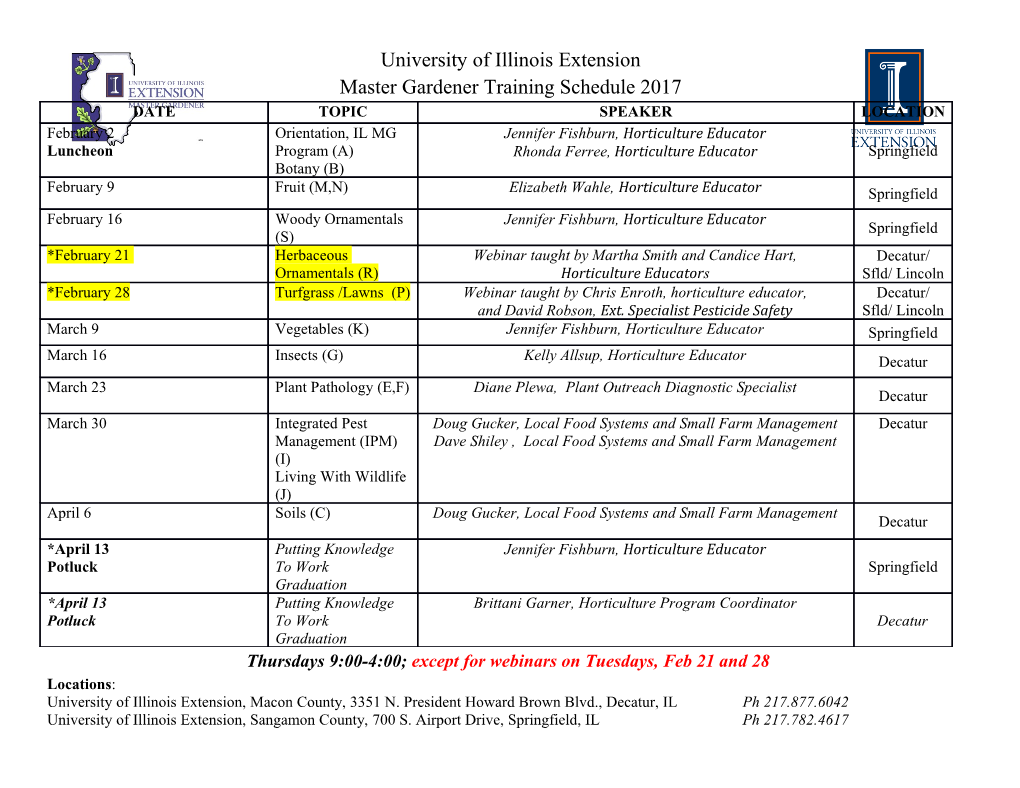
Benchmarking and Accelerating TensorFlow-based Deep Learning on Modern HPC Systems A Thesis Presented in Partial Fulfillment of the Requirements for the Degree Master of Science in the Graduate School of The Ohio State University By Rajarshi Biswas, Graduate Program in Department of Computer Science and Engineering The Ohio State University 2018 Master’s Examination Committee: Dr. Dhabaleswar K. (DK) Panda, Advisor Dr. Christopher Charles Stewart Dr. Xiaoyi Lu c Copyright by Rajarshi Biswas 2018 Abstract Google’s TensorFlow is one of the most popular Deep Learning (DL) frameworks avail- able in the community. gRPC, a Remote Procedure Call (RPC) framework also developed by Google, is the main communication engine for distributed TensorFlow. TensorFlow pri- marily uses gRPC for exchanging tensors and communicating administrative tasks among different processes across the nodes. Tensor updates during the training phase are commu- nication intensive and thus TensorFlow’s performance is heavily dependent on the under- lying network and the efficacy of the communication engine. Apart from the default gRPC channel, TensorFlow supports various high-performance channels to efficiently transfer tensors such as gRPC+Verbs and gRPC+MPI. However, at present, the community lacks a thorough characterization of these available distributed TensorFlow communication chan- nels. This is critical to understand because high-performance Deep Learning with Tensor- Flow on modern HPC systems needs an efficient communication runtime. In this work, we first conduct a meticulous analysis of the communication character- istics of distributed TensorFlow over all available channels. Based on these characteris- tics we propose TF-gRPC-Bench micro-benchmark suite that enables system researches to quickly understand the impact of the underlying network and communication runtime on DL workloads. We propose three micro-benchmarks that take account TensorFlow DL workload characteristics over gRPC. Furthermore, our characterization shows that none of the existing channels in TensorFlow can support adaptive and efficient communication for ii DL workloads with different message sizes. Moreover, the community needs to maintain these different channels while the users are also expected to tune these channels to get the desired performance. Therefore, this work proposes a unified approach to have a single gRPC runtime (i.e., AR-gRPC) in TensorFlow with Adaptive and efficient RDMA proto- cols. In AR-gRPC, we propose designs such as hybrid communication protocols, message pipelining and coalescing, zero-copy transmission etc. to make our runtime be adaptive to different message sizes for DL workloads. Our evaluations show that AR-gRPC can signif- icantly speedup gRPC performance by up to 4.1x and 2.3x compared to the default gRPC design on IPoIB and another RDMA-based gRPC design in the community. By integrating our AR-gRPC with TensorFlow, we can achieve up to 3x distributed training performance improvement over default gRPC-IPoIB based TensorFlow. iii To my family, friends, and mentors. iv Acknowledgments My deepest gratitude is to my advisor Dr. D. K. Panda for the guidance and support he has given me throughout the thesis work. I am grateful to him for giving me this important opportunity to be part of HiBD research group. His work-ethic, commitment, principles are big inspirations for me and I would always want to follow in this direction of right path. I also want to thank Dr. Christopher Charles Stewart for agreeing to be a committee member for my thesis defense exam, and make it work despite of his tight schedule and commitments. I would like to give special thanks to Dr. Xiaoyi Lu, who has been my mentor and team lead. His technical guidance and encouragement throughtout my tenure in the lab has been invaluable to me. His insightful and thought-provoking technical comments has helped me grow. His willingness to support me even in trying circumstances kept me moving forward. He brings a lot of positivity to the lab and his commitment to work is exceptional. I’ve learnt a lot working closely with him. From my family, I want to thank my mother Mrs. Minati Biswas and my father Mr. Ranajit Kumar Biswas for their continuous support and love. They have done a lot of sacrifices for me and I am grateful to have parents like them. I also want to thank my cousin sister Sampurna Biswas who has been a great support throughout my graduate studies in the US. v Finally, I thank my lab colleagues and friends Shashank, Haseeb, Moniba, Sourav, Haiyang, Dipti and others for the interactions we had. I would also like to thank all my friends back at home, particularly Arka and Chandan for encouraging me in my pursuit. vi Vita 2011 . B.E., Information Technology, Jadavpur University, India. 2011 - 2015 . Software Development Engineer, Citrix Research and Development, India 2015 - 2016 . Senior Software Development Engineer, Citrix Research and Development, India 2016 - Present . M.S., Computer Science and Engineer- ing, The Ohio State University, USA 2017 - Present . Graduate Research Associate, The Ohio State University, USA Publications X. Lu, H. Shi, R. Biswas, M. H. Javed, and D. K. Panda, DLoBD: A Comprehensive Study of Deep Learning over Big Data Stacks on HPC Clusters, In The Journal of IEEE Transactions on Multi-Scale Computing Systems, [June 2018]. R. Biswas, X. Lu, and D. K. Panda, Designing a MicroBenchmark Suite to Evaluate gRPC for TensorFlow: Early Experiences, In 9th Workshop on Big data benchmarks, Perfor- mance Optimization, and Emerging hardware (BPOE - 9), in conjunction with ASPLOS, [March 2018]. X. Lu, H. Shi, M. H. Javed, R. Biswas, and D. K. Panda, Characterizing Deep Learning over Big Data (DLoBD) Stacks on RDMA-Capable Networks, In 25th Annual Symposium on High-Performance Interconnects (HOTI ’17), [August 2017]. Fields of Study Major Field: Computer Science and Engineering vii Table of Contents Page Abstract . ii Dedication . iv Acknowledgments . .v Vita ........................................... vii List of Tables . .x List of Figures . xi 1. Introduction . .1 1.1 Motivation . .2 1.2 Organization of Thesis . .5 2. Background . .6 2.1 Overview of TensorFlow . .6 2.2 Overview of gRPC . .8 2.3 Overview of InfiniBand and RDMA . .9 3. Characterization of Distributed TensorFlow . 11 3.1 Distributed Execution of TensorFlow . 11 3.2 Methodology for Characterization . 12 3.3 Characterization for the gRPC Channel . 13 3.4 Characterization for the gRPC+Verbs Channel . 15 3.5 Characterization for the gRPC+MPI Channel . 15 3.6 Characteristics of TensorFlow Workload over gRPC Channel . 17 3.7 Summary . 18 viii 4. Designing a Micro-Benchmark Suite to Evaluate gRPC for TensorFlow . 21 4.1 Introduction . 21 4.2 TensorFlow Deep Learning Micro-benchmarks for gRPC . 22 4.2.1 Design Considerations . 22 4.2.2 Design of TF-gRPC-Bench Micro-benchmark Suite . 25 4.3 Performance Evaluation . 29 4.3.1 Experimental Setup . 29 4.3.2 TF-gRPC-P2P-Latency (Serialized Mode) . 31 4.3.3 TF-gRPC-P2P-Latency (Non-serialized Mode) . 32 4.3.4 TF-gRPC-P2P-Bandwidth (Non-serialized Mode) . 33 4.3.5 TF-gRPC-PS-Throughput (Non-serialized Mode) . 34 4.4 Related Work . 35 4.5 Summary . 36 5. Accelerating TensorFlow with Adaptive RDMA-based gRPC (AR-gRPC)... 38 5.1 Introduction . 38 5.2 Proposed Design of AR-gRPC . 39 5.2.1 Architecture Overview of AR-gRPC . 39 5.2.2 Adaptive RDMA-based Communication . 41 5.3 Performance Evaluation . 44 5.3.1 Experimental Setup . 45 5.3.2 Evaluation of gRPC . 45 5.3.3 Evaluation of AR-gRPC Enhanced TensorFlow . 52 5.4 Related Work . 59 5.5 Summary . 61 6. Conclusion and Future Work . 62 Bibliography . 63 ix List of Tables Table Page 1.1 Comparison with Related Work . .4 3.1 TensorFlow Performance for Resnet50 . 13 4.1 iovec Buffer Size Category . 28 4.2 Configurable Parameters for TF-gRPC-Bench Micro-benchmark Suite . 30 x List of Figures Figure Page 1.1 Contrast Between Current and Proposed Deep Learning Benchmarks . .3 2.1 Overview of TensorFlow . .7 2.2 Overview of gRPC Deployment . .9 3.1 Communication Pattern Between TensorFlow Parameter Servers and Workers 12 3.2 TensorFlow Payload Distribution and Communication Flow over gRPC channel . 14 3.3 TensorFlow Payload Distribution and Communication Flow over gRPC+Verbs channel . 16 3.4 TensorFlow Payload Distribution and Communication Flow over gRPC+MPI channel . 17 3.5 iovec Buffer Distribution Observed for TensorFlow training over gRPC . 18 4.1 Design Considerations for TF-gRPC-Bench Micro-benchmark . 23 4.2 TF-gRPC-Bench Micro-benchmark Deign . 26 4.3 TF-gRPC-P2P-Latency (Serialized Mode) Evaluation on Cluster A with 64KBytes Payload . 31 4.4 TF-gRPC-P2P-Latency (Non-serialized Mode) . 32 4.5 TF-gRPC-P2P-Latency (Non-serialized Mode) Evaluation on Cluster A for Different iovec Counts . 33 xi 4.6 TF-gRPC-P2P-Bandwidth (Non-serialized Mode) . 34 4.7 TF-gRPC-PS-Throughput (Non-serialized Mode) . 35 5.1 Overview of AR-gRPC and the Corresponding Communication in Tensor- Flow...................................... 40 5.2 gRPC Point-to-Point Latency Evaluation on Cluster A . 46 5.3 gRPC Point-to-Point Latency Evaluation on Cluster B . 47 5.4 Analysis of Various gRPC Designs on Cluster A . 49 5.5 gRPC Single Server, Multiple Clients Throughput Evaluation on Cluster A . 50 5.6 Performance Comparison in Fully-Connected Architecture of gRPC . 51 5.7 Inception4 Evaluation on Cluster A (Higher Better); TotalBatchSize = (BatchSize=GPU) × NUMo f GPUs ..................... 54 5.8 Resnet152 Evaluation on Cluster A (Higher Better); TotalBatchSize = (BatchSize=GPU) × NUMo f GPUs ..................... 55 5.9 Inception3 Evaluation on Cluster A (Higher Better); TotalBatchSize = (BatchSize=GPU) × NUMo f GPUs ..................... 56 5.10 Resnet50 Evaluation on Cluster A (Higher is Better); TotalBatchSize = (BatchSize=GPU) × NUMo f GPUs ....................
Details
-
File Typepdf
-
Upload Time-
-
Content LanguagesEnglish
-
Upload UserAnonymous/Not logged-in
-
File Pages84 Page
-
File Size-