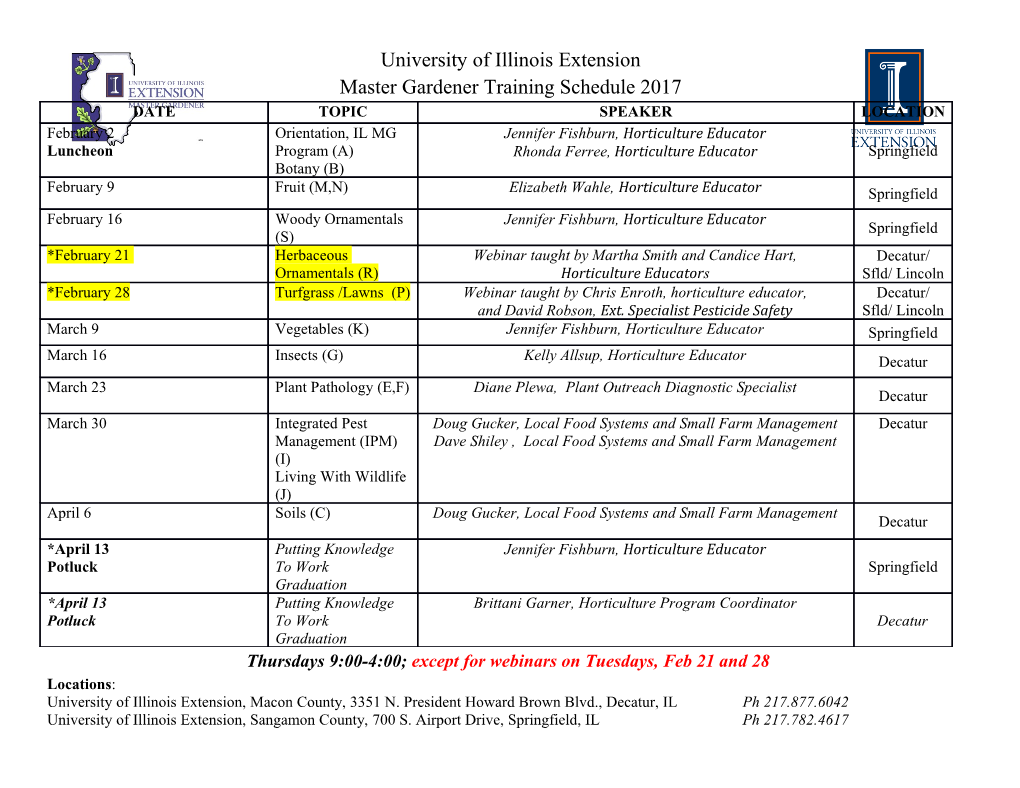
Nonparametric Bayesian Methods Tamara Broderick Michael I. Jordan ITT Career Development Assistant Professor Pehong Chen Distinguished Professor EECS EECS, Statistics MIT UC Berkeley Nonparametric Bayesian Methods: Part I Tamara Broderick ITT Career Development Assistant Professor EECS MIT Nonparametric Bayes 1 Nonparametric Bayes • Bayesian 1 Nonparametric Bayes • Bayesian P(parameters data) P(data parameters)P(parameters) | / | 1 Nonparametric Bayes • Bayesian P!(parameters data) P(data parameters)P(parameters) | / | • Not parametric (i.e. not finite parameter, unbounded/ growing/infinite number of parameters) 1 Nonparametric Bayes • Bayesian P!(parameters data) P(data parameters)P(parameters) | / | • Not parametric (i.e. not finite parameter, unbounded/ growing/infinite number of parameters) [wikipedia.org] 1 Nonparametric Bayes • Bayesian P!(parameters data) P(data parameters)P(parameters) | / | • Not parametric (i.e. not finite parameter, unbounded/ growing/infinite number of parameters) “Wikipedia phenomenon” [wikipedia.org] 1 Nonparametric Bayes • Bayesian P!(parameters data) P(data parameters)P(parameters) | / | • Not parametric (i.e. not finite parameter, unbounded/ growing/infinite number of parameters) [wikipedia.org] 1 Nonparametric Bayes • Bayesian P!(parameters data) P(data parameters)P(parameters) | / | • Not parametric (i.e. not finite parameter, unbounded/ growing/infinite number of parameters) [Ed Bowlby, NOAA] [wikipedia.org] 1 Nonparametric Bayes • Bayesian P!(parameters data) P(data parameters)P(parameters) | / | • Not parametric (i.e. not finite parameter, unbounded/ growing/infinite number of parameters) [Ed Bowlby, NOAA] [Fox et al 2014] [wikipedia.org] 1 Nonparametric Bayes • Bayesian P!(parameters data) P(data parameters)P(parameters) | / | • Not parametric (i.e. not finite parameter, unbounded/ growing/infinite number of parameters) [Lloyd et al 2012; Miller et al 2010] [Ed Bowlby, NOAA] [Fox et al 2014] [wikipedia.org] 1 Nonparametric Bayes • Bayesian P!(parameters data) P(data parameters)P(parameters) | / | • Not parametric (i.e. not finite parameter, unbounded/ growing/infinite number of parameters) [Lloyd et al 2012; Miller et al 2010] [Ed Bowlby, NOAA] [Fox et al 2014] [wikipedia.org] [Sudderth, Jordan 2009] 1 Nonparametric Bayes • Bayesian P!(parameters data) P(data parameters)P(parameters) | / | • Not parametric (i.e. not finite parameter, unbounded/ growing/infinite number of parameters) [Lloyd et al 2012; Miller et al 2010] [Ed Bowlby, NOAA] [Fox et al 2014] [Ewens [wikipedia.org] 1972; Hartl, Clark 2003] [Sudderth, Jordan 2009] 1 Nonparametric Bayes • Bayesian P!(parameters data) P(data parameters)P(parameters) | / | • Not parametric (i.e. not finite parameter, unbounded/ growing/infinite number of parameters) [Lloyd et al 2012; Miller et al 2010] [Ed Bowlby, NOAA] [Fox et al 2014] [Ewens [wikipedia.org] 1972; Hartl, Clark 2003] [Saria [Sudderth, et al Jordan 2009] 1 2010] Nonparametric Bayes • Bayesian P!(parameters data) P(data parameters)P(parameters) | / | • Not parametric (i.e. not finite parameter, unbounded/ growing/infinite number of parameters) [Lloyd et al 2012; Miller et al 2010] [Ed Bowlby, NOAA] [Fox et al 2014] [Arjas, Gasbarra [Ewens [wikipedia.org] 1994] 1972; Hartl, Clark 2003] [Saria [Sudderth, et al Jordan 2009] 1 2010] Nonparametric Bayes • Bayesian P!(parameters data) P(data parameters)P(parameters) | / | • Not parametric (i.e. not finite parameter, unbounded/ growing/infinite number of parameters) [Lloyd et al 2012; Miller et al 2010] [Ed Bowlby, NOAA] [Fox et al 2014] [Arjas, Gasbarra [Ewens [wikipedia.org] 1994] 1972; Hartl, [Escobar, Clark West 1995; 2003] [Saria Ghosal [Sudderth, et al et al 1999] Jordan 2009] 1 2010] Roadmap • Example problem: clustering • Example NPBayes model: Dirichlet process • Big questions • Why NPBayes? • What does a growing/infinite number of parameters really mean (in NPBayes)? • Why is NPBayes challenging but practical? 2 Clustering 3 Clustering 4 Clustering 4 Clustering P(parameters data) P(data parameters)P(parameters) | / | 4 Generative model P(parameters data) P(data parameters)P(parameters) | / | 4 Generative model P(parameters data) P(data parameters)P(parameters) | / | • Finite Gaussian mixture model (K=2 clusters) 4 Generative model P(parameters data) P(data parameters)P(parameters) | / | • Finite Gaussian mixture model (K=2 clusters) µ iid (µ , ⌃ ) k ⇠ N 0 0 4 Generative model P(parameters data) P(data parameters)P(parameters) | / | • Finite Gaussian mixture model (K=2 clusters) µ iid (µ , ⌃ ) k ⇠ N 0 0 z iid Categorical(⇢ , ⇢ ) n ⇠ 1 2 4 Generative model P(parameters data) P(data parameters)P(parameters) | / | • Finite Gaussian mixture model (K=2 clusters) µ iid (µ , ⌃ ) k ⇠ N 0 0 iid zn Categorical(⇢1, ⇢2) ⇠indep x (µ , ⌃) n ⇠ N zn 4 Generative model P(parameters data) P(data parameters)P(parameters) | / | • Finite Gaussian mixture model (K=2 clusters) µ iid (µ , ⌃ ) k ⇠ N 0 0 iid zn Categorical(⇢1, ⇢2) ⇠indep x (µ , ⌃) n ⇠ N zn 4 Generative model P(parameters data) P(data parameters)P(parameters) | / | • Finite Gaussian mixture model (K=2 clusters) µ iid (µ , ⌃ ) k ⇠ N 0 0 ⇢ Beta(a ,a ) 1 ⇠ 1 2 ⇢2 =1 ⇢1 iid − zn Categorical(⇢1, ⇢2) ⇠indep x (µ , ⌃) n ⇠ N zn ⇢1 ⇢2 4 Generative model P(parameters data) P(data parameters)P(parameters) | / | • Finite Gaussian mixture model (K=2 clusters) µ iid (µ , ⌃ ) k ⇠ N 0 0 ⇢ Beta(a ,a ) 1 ⇠ 1 2 ⇢2 =1 ⇢1 iid − zn Categorical(⇢1, ⇢2) ⇠indep x (µ , ⌃) n ⇠ N zn ⇢1 ⇢2 4 Generative model P(parameters data) P(data parameters)P(parameters) | / | • Finite Gaussian mixture model (K=2 clusters) µ iid (µ , ⌃ ) k ⇠ N 0 0 ⇢ Beta(a ,a ) 1 ⇠ 1 2 ⇢2 =1 ⇢1 iid − zn Categorical(⇢1, ⇢2) ⇠indep x (µ , ⌃) n ⇠ N zn ⇢1 ⇢2 4 Beta distribution review ⇢1 (0, 1) Γ(a1 + a2) a1 1 a2 1 2 Beta(⇢1 a1,a2)= ⇢1 − (1 ⇢1) − a1,a2 > 0 | Γ(a1)Γ(a2) − 5 Beta distribution review ⇢1 (0, 1) Γ(a1 + a2) a1 1 a2 1 2 Beta(⇢1 a1,a2)= ⇢1 − (1 ⇢1) − a1,a2 > 0 | Γ(a1)Γ(a2) − density ρ1 5 Beta distribution review ⇢1 (0, 1) Γ(a1 + a2) a1 1 a2 1 2 Beta(⇢1 a1,a2)= ⇢1 − (1 ⇢1) − a1,a2 > 0 | Γ(a1)Γ(a2) − • What happens? density ρ1 5 Beta distribution review ⇢1 (0, 1) Γ(a1 + a2) a1 1 a2 1 2 Beta(⇢1 a1,a2)= ⇢1 − (1 ⇢1) − a1,a2 > 0 | Γ(a1)Γ(a2) − • What happens? a = a1 = a2 0 density a = a = a ! 1 2 !1 a1 >a2 ρ1 5 Beta distribution review ⇢1 (0, 1) Γ(a1 + a2) a1 1 a2 1 2 Beta(⇢1 a1,a2)= ⇢1 − (1 ⇢1) − a1,a2 > 0 | Γ(a1)Γ(a2) − • What happens? a = a1 = a2 0 density a = a = a ! 1 2 !1 a1 >a2 [demo] ρ1 5 Generative model P(parameters data) P(data parameters)P(parameters) | / | • Finite Gaussian mixture model (K clusters) 6 Generative model P(parameters data) P(data parameters)P(parameters) | / | • Finite Gaussian mixture model (K clusters) 6 Generative model P(parameters data) P(data parameters)P(parameters) | / | • Finite Gaussian mixture model (K clusters) µ iid (µ , ⌃ ) k ⇠ N 0 0 6 Generative model P(parameters data) P(data parameters)P(parameters) | / | • Finite Gaussian mixture model (K clusters) µ iid (µ , ⌃ ) k ⇠ N 0 0 ⇢ Dirichlet(a ) 1:K ⇠ 1:K ⇢1 ⇢2 ⇢3 6 Generative model P(parameters data) P(data parameters)P(parameters) | / | • Finite Gaussian mixture model (K clusters) µ iid (µ , ⌃ ) k ⇠ N 0 0 ⇢ Dirichlet(a ) 1:K ⇠ 1:K z iid Categorical(⇢ ) n ⇠ 1:K ⇢1 ⇢2 ⇢3 6 Generative model P(parameters data) P(data parameters)P(parameters) | / | • Finite Gaussian mixture model (K clusters) µ iid (µ , ⌃ ) k ⇠ N 0 0 ⇢ Dirichlet(a ) 1:K ⇠ 1:K z iid Categorical(⇢ ) n ⇠ 1:K indep x (µ , ⌃) n ⇠ N zn ⇢1 ⇢2 ⇢3 6 Dirichlet distribution review K K Γ( k=1 ak) ak 1 Dirichlet(⇢1:K a1:K )= ⇢ − a > 0 | K k k k=1 Γ(ak) P kY=1 Q a = ak =1 a = ak 0 a = a ! k !1 7 Dirichlet distribution review K K Γ( k=1 ak) ak 1 Dirichlet(⇢1:K a1:K )= ⇢ − a > 0 | K k k kP=1 Γ(ak) k=1 Y ⇢k (0, 1) 2 Q ⇢k =1 Xk a = ak =1 a = ak 0 a = a ! k !1 7 Dirichlet distribution review K K Γ( k=1 ak) ak 1 Dirichlet(⇢1:K a1:K )= ⇢ − a > 0 | K k k kP=1 Γ(ak) k=1 Y ⇢k (0, 1) 2 Q ⇢k =1 k a = (0.5,0.5,0.5) a = (5,5,5) a = (40,10,10)X density ρ2 ρ1 7 Dirichlet distribution review K K Γ( k=1 ak) ak 1 Dirichlet(⇢1:K a1:K )= ⇢ − a > 0 | K k k kP=1 Γ(ak) k=1 Y ⇢k (0, 1) 2 Q ⇢k =1 k a = (0.5,0.5,0.5) a = (5,5,5) a = (40,10,10)X density ρ2 ρ1 • What happens? a = ak =1 a = ak 0 a = ak ! [demo]!1 7 So far K << N. What if not? • e.g. species sampling, topic modeling, groups on a social network, etc. … ⇢1 ⇢2 ⇢3 ⇢1000 • Components: number of latent groups • Clusters: number of components represented in the data • Number of clusters for N data points is < K and random • Number of clusters grows with N 8 So far K << N. What if not? … ⇢1 ⇢2 ⇢3 ⇢1000 • Components: number of latent groups • Clusters: number of components represented in the data • Number of clusters for N data points is < K and random • Number of clusters grows with N 8 So far K << N. What if not? • e.g. species sampling, topic modeling, groups on a social network, etc. … ⇢1 ⇢2 ⇢3 ⇢1000 • Components: number of latent groups • Clusters: number of components represented in the data • Number of clusters for N data points is < K and random • Number of clusters grows with N 8 So far K << N.
Details
-
File Typepdf
-
Upload Time-
-
Content LanguagesEnglish
-
Upload UserAnonymous/Not logged-in
-
File Pages114 Page
-
File Size-