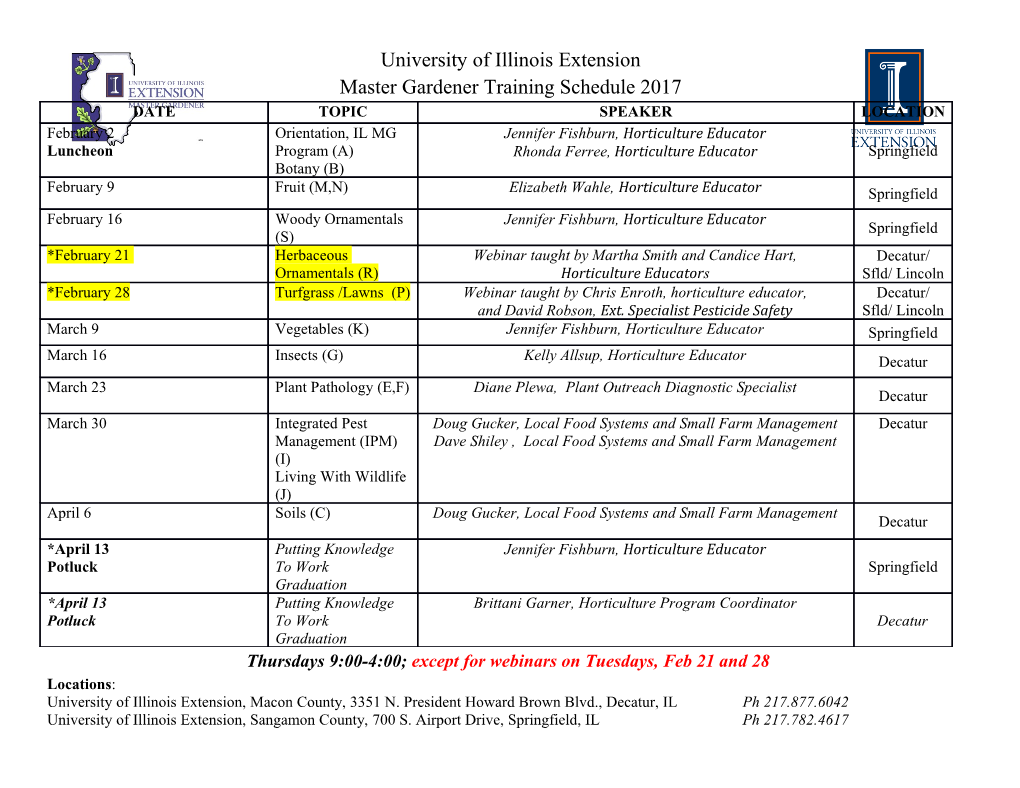
Internationl School for Advanced Studies (SISSA/Trieste) Attractors, memory and perception by Athena Akrami Supervisor Alessandro Treves A thesis submitted in partial fulfillment for the degree of Doctor of Philosophy in the Cognitive Neuroscience Sector Scuola Internazionale Superiore di Studi Avanzati January 2010 Summary of the work In this Thesis, the first three introductory chapters are devoted to the review of literature on contextual perception, its neural basis and network modeling of memory. In chapter 4, the first two sections give the definition of our model; and the next two sections, 4.3 and 4.4, report the original work of mine on retrieval properties of different network structures and network dynamics underlying the response to ambiguous patterns, respectively. The reported work in chapter 5 has been done in collaboration with Prof Bharathi Jagadeesh in University of Washington, and is already published in the journal ”Cerebral Cortex”. In this collaboration, Yan Liu, from the group in Seattle, carried out the recording experiments and I did the data analysis and network simulations. Chapter 6, which represents a network model for ”priming” and ”adaptation aftereffect” is done by me. The works reported in 4.3, 4.5, and the whole chapter 6 are in preparation for publication. Acknowledgements I thank Alessandro who taught me how to face ambiguity in Limbo. I thank him again for his (failed) attempts to foster a minimalistic, concise and essential style in my way of thinking. I thank Bharathi and her family with whom I had memorable moments in Seattle, while exploring the experimental world of Neuroscience. I thank my family whose omnipresent memories blessed every moment of my life at migrate. I thank SISSA for ”considerate la vostra semenza: fatti non foste a viver come bruti, ma per seguir virtute e canoscenza”. I thank my unique friends, Arash, Eleonora, Valentina and Erika who often had to bear the brunt of my frustration, rages and recalcitrance against the world and offered me their open hearts, in lieu, to regain my equanimity. I thank the gang of my fellow friends with whom I practiced life and refreshed identity. I thank my homeland whose fervent resistance and bravery inspired me with determination and hope. and my special thanks to the one whose name may not be revealed explicitly, for the countless support in any sense of the word. v Contents Summary of the work iv Acknowledgements v 1 Introduction 1 1.1 General issues ....................................... 1 1.1.1 Adaptation .................................... 1 1.1.2 Ambiguous perception .............................. 2 1.1.3 Memory-based prediction ............................ 2 Linking memory and perception ..................... 3 1.1.4 Neocortex as an autoassociative memory .................... 4 1.2 This Thesis: specific questions ............................. 4 1.2.1 The storage capacity in different network architectures ............ 5 1.2.2 The response to morphed patterns in analog networks ............ 5 Can we see retrieval dynamics by probing a local cortical network? . 5 1.2.2.1 The response to morphed patterns in IT Cortex, relevance of at- tractor dynamics ............................ 6 Electrophysiological evidence for attractor dynamics? ......... 6 1.2.3 Experience-dependent dynamics of attractor networks in response to mor- phed patterns ................................... 7 Can local attractor dynamics help us understand the effect of recent experience? .......................... 7 2 Visual Adaptation 9 2.1 Repetition: perceptual consequences .......................... 9 2.1.1 Perceptual priming ................................ 10 2.1.1.1 Priming as a memory phenomenon .................. 11 2.1.2 Adaptation aftereffect .............................. 12 2.1.3 Stereotypical dynamic properties for different adaptation aftereffect . 13 2.1.4 Switch between adaptation aftereffect and priming .............. 14 2.2 Masking .......................................... 16 vii viii 2.3 Neural substrates for perceptual priming ........................ 18 2.3.1 Timecourse of information processing in visual cortex ............ 18 2.3.2 Repetition: average network effects ....................... 20 2.3.2.1 Repetition adaptation in single neurons ............... 20 2.3.2.2 Match enhancement .......................... 21 2.3.2.3 Repetition suppression in fMRI signals ............... 22 Repetition lag ............................... 23 2.3.3 Is repetition suppression related to behaviour? ................ 23 Semantic priming ............................. 25 2.4 Review of the current models .............................. 25 Is bistable perception a memory-less phenomena? ........... 31 2.4.1 Concluding remarks ............................... 31 3 Associative Memory Networks 33 3.1 Memory in the cortex .................................. 33 3.1.1 Synaptic plasticity and memory ......................... 35 3.2 Visual memory and object representation in cortex .................. 36 Exponentially high coding capacity ................... 37 Ease with which the code can be read by receiving neurons ..... 37 Generalization, completion, graceful degradation, and higher resis- tance to noise ........................ 37 Speed of readout of the information .................. 37 Invariance in the neuronal representation of stimuli .......... 38 3.2.1 Role of IT ..................................... 38 3.2.1.1 Representation of familiar and novel objects in IT ......... 39 3.2.1.2 Delay period and contribution of IT, prefrontal cortex and prihinal coretx .................................. 40 3.2.1.3 Silent delay activity .......................... 41 3.3 Simple models of memory storage and memory retrieval ............... 42 3.3.1 A canonical microcircuit for neocortex ..................... 42 excitatory connections .......................... 43 Inhibitory interneurons and their connections ............. 43 Double banquet .............................. 44 Chandelier cells .............................. 44 Martinotti cells .............................. 44 3.3.2 Attractor neural networks: the Hopfield model and its variants ....... 44 3.3.2.1 The basic formalism of the Hopfield model ............. 45 3.3.2.2 Simple models meet psychology, not neurophysiology ....... 47 Towards a test of the attractor neural network paradigm ....... 48 3.4 Dynamics of large, more realistic associative networks ................ 49 3.4.1 Dynamics of sparsely connected spiking neurons ............... 49 Synapses .................................. 50 Mean-field analysis ............................ 51 ix Different time scales ........................... 52 Summary ................................. 54 3.4.1.1 Realistic time course of synaptic conductance ............ 54 3.4.2 Dynamics in rate-models ............................ 56 What about the transients about this solution? ............ 57 1 ...................................... 58 2 ...................................... 58 3.5 Spike frequency adaptation ............................... 58 3.5.1 Prediction through adaptation ......................... 59 3.5.2 Possible mechanisms underlying spike-rate adaptation ............ 60 3.6 Gain modulation ..................................... 60 Functional differentiation of GABAa and GABAb ........... 62 3.7 Concluding remarks ................................... 63 4 Theoretical basis of the model 65 4.1 Structure of the model .................................. 66 4.1.1 General parameters and analytical approach .................. 66 The input to each unit. ......................... 66 Stored memory patterns ......................... 67 Synaptic weights. ............................. 67 Temporal evolution of the firing rates. ................. 67 Overlap .................................. 68 The noise term (crosstalk effect) .................... 69 Firing rate probability, as a function of the signal. .......... 70 4.1.2 Modeling the adaptation current ........................ 71 Spiking neuron .............................. 72 Rate model ................................ 73 4.1.3 Mean activity, sparseness, and setting threshold and gain .......... 74 Mean activity ............................... 74 Sparseness ................................. 74 4.2 Simulation setting .................................... 76 4.2.1 General characteristics of the simulated network ............... 76 Dynamics ................................. 78 Input patterns .............................. 78 Weights .................................. 78 Recurrent collaterals do not add signal during the storage ...... 79 Active process to regulate threshold and gain (fixed level of sparseness 80 Implementation of adaptation as firing rate decay ........... 81 4.3 Result: Retrieval in different network structures .................... 82 Background ................................ 82 Dilution in connectivity and storage capacity ............. 83 Noise Reverberations ........................... 83 Three comparisons ............................ 84 x 4.3.1 General points: sparseness and cross correlation ............... 85 Input patterns .............................. 85 Parameters of the quenched patterns .................. 86 Different distributions with the same sparseness. ........... 87 Result: sparseness sets correlation ................... 87 4.3.2 Three comparisons: binary vs. continuous, quenched assigned vs. self organized, and 1-layer vs. 2-layer. ....................... 87 Introducing dilution in our model .................... 88 Introducing
Details
-
File Typepdf
-
Upload Time-
-
Content LanguagesEnglish
-
Upload UserAnonymous/Not logged-in
-
File Pages222 Page
-
File Size-