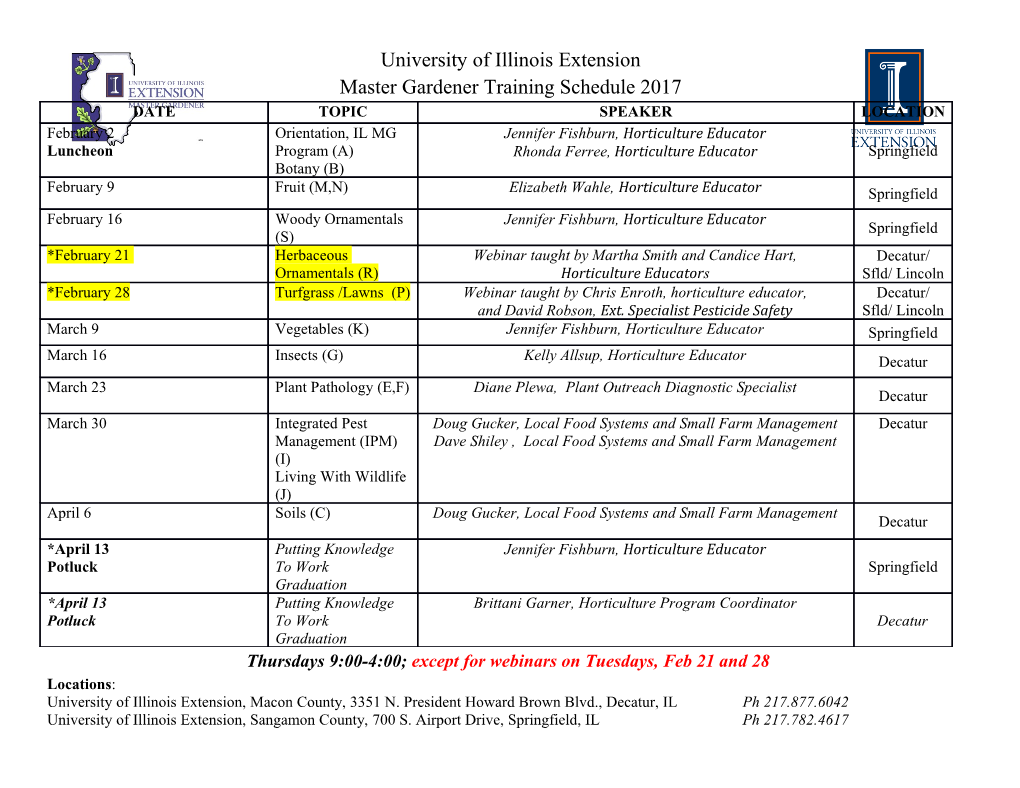
DCT/IDCT Filter Design for Ultrasound Image Filtering Barmak Honarvar Shakibaei1,2,*, Jan Flusser1, Yifan Zhao2, John Ahmet Erkoyuncu2 and Rajkumar Roy3 1Czech Academy of Sciences, Institute of Information 2Through-Life Engineering Services Centre, Theory and Automation, Pod vodarenskou´ veˇzˇ´ı 4, School of Aerospace, Transport and Manufacturing, 18208 Praha 8, Czech Republic Cranfield University, Bedfordshire MK43 0AL, UK Email: honarvar,flusser @utia.cas.cz Email: barmak,yifan.zhao,j.a.erkoyuncu @cranfield.ac.uk { } { } 3School of Mathematics, Computer Science and Engineering City, University of London, Northampton Square, London, EC1V 0HB, UK Email: [email protected] Abstract—In this paper, a new recursive structure based on the convolution model of discrete cosine transform (DCT) is correspondingly often called simply “the inverse DCT” or for designing of a finite impulse response (FIR) digital filter “the IDCT”. The N-point DCT-II of a discrete signal, x(n) is is proposed. In our derivation, we start with the convolution given by model of DCT-II to use its Z-transform for the proposed filter structure perspective. Moreover, using the same algorithm, a N−1 filter base implementation of the inverse DCT (IDCT) for image π 1 X = c(k) x(n) cos n + k , (1) reconstruction is developed. The computational time experiments k N 2 n=0 of the proposed DCT/IDCT filter(s) demonstrate that the pro- X posed filters achieve faster elapsed CPU time compared to the for k = 0, 1,...,N 1, where others. The image filtering and reconstruction performance of − 1 the proposed approach on ultrasound images are presented to , k = 0 validate the theoretical framework. √N c (k)= 2 I. INTRODUCTION , otherwise. N DCT has found wide applications in signal and image r The above scale factor can be rewritten in processing in general, and in data compression, filtering and terms of the unit impulse and step functions as feature extraction in particular. The DCT has been proved √ √ . The inverse 1-D successful at decorrelating and correlating the energy of image c(k) = δ(k)+ 2u(k 1) / N discrete cosine transform (IDCT)-II− can be defined as data. After decorrelation, each DCT coefficient can be en- N−1 coded independently without lossing compression efficiency π 1 x(n)= c(k)X cos n + k , (2) since it has a strong ‘energy compation’ property in typical k N 2 k=0 applications [1]. In comparison to discrete Fourier transform X (DFT), DCT is a real transform that transforms a sequence of for n = 0, 1,...,N 1. real data points into its real spectrum and therefore avoids the Medical ultrasound− images are usually corrupted by noise problem of redundancy. Also, as DCT is derived from DFT, in its acquisition and transmission. Hand-held ultrasound scan- all the desirable properties of DFT (such as the fast algorithm) ners are increasingly being employed at the point of care and are preserved. To reduce DCT computational complexities, the used in telemedicine to serve rural population limited access development of fast and efficient algorithms for computing 2- to hospitals [3]. However, image quality of these portable D DCT/IDCT becomes increasingly important. Various fast al- systems are in general poorer than those of standard scanners. gorithms for computing 2-D DCT were proposed to minimize They are also often used in scans by physicians rather than the computational complexity [2]. However, there are a variety by expert sonographers. Thus, the poor image quality is one of DCT of which four are common (DCT-I, DCT-II, DCT-III, of major drawbacks of the ultrasound image due to speckle and DCT-IV). Each differs by only a bit, and each has its noise. In general, ultrasound images have two main noise own usage in particular field. For image reconstruction, DCT components - electronic noise, modeled as an additive white II is used to decompose and DCT III is used to reconstruct. Gaussian noise, and speckle noise. In raw RF data, speckle Each DCT has its cosine basis kernel which is orthogonal. The noise is multiplicative but in the B-mode image we consider most common variant of discrete cosine transform is the type- it as an additive noise due to the log transform. Speckle noise II DCT, which is often called simply “the DCT”. Its inverse is correlated with the signal and is not Gaussian [4]. However, the proposed denoising suppresses all additive components regardless of their probability distribution. On the other hand, multiplicative speckle noise is generally more difficult to remove than additive noise, because the intensity of the noise varies with the image intensity [5]. Image noise is usually Fig. 1: A simple FIR filter structure with impulse response random, but ultrasound speckle is not random and results hk(n) for generating DCT-II coefficients from a flipped input from some patterns of constructive and destructive interference signal. shown as bright and dark dots in the image. Sometimes speckle f(n) of length N, over a kernel function of g(n,k) can be helps to identify the boundaries better in ultrasound images derived by the discrete convolution of the kernel and the than without speckle. In addition to speckle, there is thermal flipped signal which is evaluated at N 1. noise in ultrasound images arising due to electronics. In this Proof. The discrete transform for a− 1-D signal f(n) of research, the proposed method deals with the additive noise length N with any kernel function of g(n,k), can be written which is pertinent to addressing the image quality of low cost as: scanners in which noise performance of amplifiers may be low compared to high end scanners. The proposed method N−1 also allows for reconstruction after compression which may Fk = f(n)g(n,k). (3) n=0 be necessary in telemedicine (when images will need to be X transmitted over limited band widths). The presence of speckle By changing n to N 1 n, the above equation can be written − − noise affects difficulties on features extraction and quantitative as: measurement of ultrasound images. There are some algorithms N−1 to suppress the speckle noise while attempting to preserve Fk = f(N 1 n)g(N 1 n,k) the image content using combination of Gaussian filter and − − − − n=0 DCT approach [6]. Furthermore, the main challenge in image NX−1 denoising techniques is to remove such noises while preserving = f F (n)g(N 1 n,k) (4) − − the important features and details. Filtering techniques can n=0 be classified as single scale spatial filtering (linear, nonlinear, X adaptive methods, etc.) and multiscale filtering (anisotropic = f F (n) g(n,k) , ∗ diffusion-based methods, DCT, Wiener, wavelet, curvelet, n=N−1 contourlet, etc.). Mean filtering and Gaussian filtering are where f F (n) is the flipped version of the input signal. Us- the examples of linear methods which blur the sharp edges, ing the definition of 1-D discrete convolution for the above destroy lines and suppress the details [7], [8]. The authors in −1 equation, we end up with N f(n)g(n,k) = f F (n) [8] also showed that filtering efficiency depends considerably n=0 ∗ g(n,k) , which completes the proof of Theorem 1. on DCT coefficient statistics. n=N−1 P In this paper, our approach toward deriving an FIR filter A. FIR filter implementation for 1-D DCT-II structure is based on a convolution equation to simplify 1- D DCT in terms of the flipped input signal. We then obtain By applying Theorem 1 to DCT-II definition in (1) and the transfer function of the FIR filter in Z-domain to find a considering the kernel function as a cosine signal, g(n,k) = π 1 simple filter structure of DCT coefficients generation [9], [10]. cos N n + 2 k , we get: Finally, using the orthogonality property of cosine function, we N−1 π 1 derive the IDCT-II FIR filter structure to recover the original X = c(k) x(n) cos n + k k N 2 signal based on its limited DCT-II coefficients by applying n=0 the same method of transfer function design. The main con- X (5) F tribution of this study is in developing a new computational = c(k) x (n) hk (n) , ( ∗ ) algorithm for denoising of ultrasound images to have a better n=N−1 image quality performance. Moreover, the proposed FIR filters 1 where h (n) = cos π n + k . The function h (n) is make an automatic system to accelerate the generated DCT k N 2 k called the digital filter impulse response which is the same coefficients to apply it for the proposed DCT-based ultrasound as kernel function g(n,k). Such a system is shown in Fig. 1. image filtering. The system feeds by a flipped signal and generates the DCT-II II. DERIVATION OF A RECURSIVE ALGORITHM FOR 1-D coefficients which are sampled at N 1. − DCT AND IDCT To find the FIR filter structure of the above system, it is easy to obtain the transfer function of the system in Z-domain Before deriving a recursive algorithm for 1-D DCT based (H (z)). We start to expand the cosine function of h (n) as on FIR digital filter structure, we show how to get a 1-D signal k k follow: transform based on any kernel function using a simple discrete convolution in the following Theorem. πnk πnk hk(n)= αk cos βk sin , (6) Theorem 1. A discrete transformation of a discrete signal, N − N 2 Fig.
Details
-
File Typepdf
-
Upload Time-
-
Content LanguagesEnglish
-
Upload UserAnonymous/Not logged-in
-
File Pages8 Page
-
File Size-