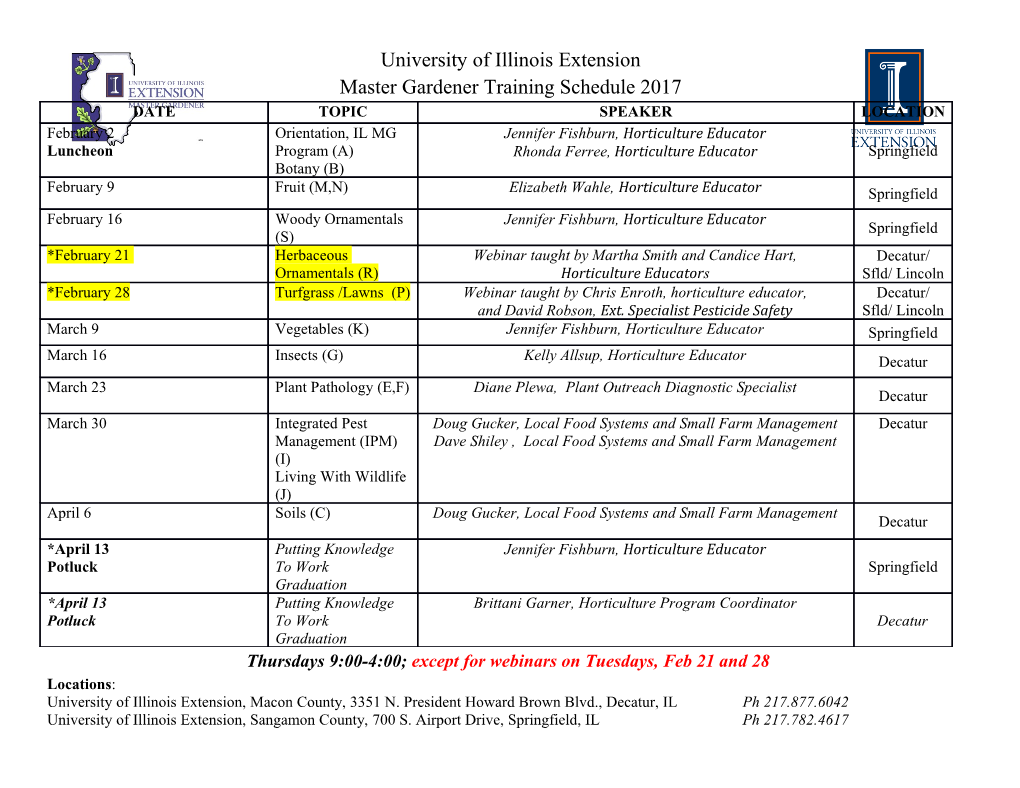
UNIVERSITY OF CALIFORNIA SANTA CRUZ ACCURATE, FAIR, AND EXPLAINABLE: BUILDING HUMAN-CENTERED AI A dissertation submitted in partial satisfaction of the requirements for the degree of DOCTOR OF PHILOSOPHY in COMPUTER SCIENCE by Aaron Springer June 2019 The Dissertation of Aaron Springer is approved: Dr. Steve Whittaker, Chair Dr. Marilyn Walker Dr. Peter Pirolli Lori Kletzer Vice Provost and Dean of Graduate Studies Copyright c by Aaron Springer 2019 Table of Contents List of Figures vii List of Tables viii Abstract ix Acknowledgments x 1 Introduction 1 1.1 Contributions . 5 1.1.1 Designing Accurate Personal Informatics Systems: . 5 1.1.2 Auditing and Removing Algorithmic Bias: . 6 1.1.3 When Do Users Want Transparency: . 6 1.1.4 How Do Users Want to Interact with Transparency: . 8 1.1.5 AI and Explanations in the Wild: . 8 2 Literature Review 9 2.1 New Aspects of User Experience in Intelligent Systems . 11 2.1.1 Accuracy . 11 2.1.2 Transparency . 14 2.1.3 Algorithmic Bias and Machine Learning Fairness . 20 2.2 Domains . 25 2.2.1 Emotional Analytics . 26 2.2.2 Learning Analytics . 32 2.2.3 Voice Interfaces . 33 3 Improving Accuracy in Intelligent Systems 37 3.1 Introduction: Why we Need Accurate Mood Models to Promote Mental Wellbeing 38 3.2 Methods . 43 3.2.1 EmotiCal System Overview . 43 3.2.2 Users . 51 3.2.3 Procedure . 51 iii 3.3 Results . 52 3.3.1 Modeling the Impact of Activities on Daily Mood . 52 3.3.2 Activities are Critical for Explaining Mood: Health and Social are Im- portant . 53 3.3.3 User Explanations Improve Mood Models . 56 3.4 Discussion . 64 4 Removing Bias in Intelligent Systems 70 4.1 Introduction . 71 4.2 Methods . 74 4.2.1 Prototype and Infrastructure . 75 4.2.2 Identifying Underserved Content . 76 4.3 Identifying Content Results . 79 4.3.1 Voice Interfaces May Underserve Specific Genres . 79 4.3.2 Typology of Underserved Content . 82 4.4 Correcting Underserved Content . 86 4.5 Correcting Content Results . 91 4.5.1 Aliases Improve Content Accessibility . 92 4.6 Discussion . 94 4.6.1 Limitations and Trade-Offs for Practitioners . 96 4.6.2 Bugs or Biases . 97 4.6.3 Creative Intricacies . 98 4.6.4 Conclusion . 98 5 When Do Users Want Transparency 99 5.1 Introduction . 100 5.1.1 Contribution . 102 5.2 Why Emotional Analytics? . 103 5.3 Research System: E-meter . 104 5.3.1 Machine Learning Model . 105 5.3.2 Version 1: Document-level: . 106 5.3.3 Version 2: Word-level . 106 5.4 Study 1 . 108 5.4.1 Method . 108 5.4.2 Study 1 Results . 111 5.4.3 Study 1 Summary . 113 5.5 Study 2 . 114 5.5.1 Method . 114 5.5.2 Users . 115 5.5.3 Measures . 115 5.5.4 Study 2 Results . 115 5.5.5 Study 2 Discussion . 122 5.6 Study 3 . 122 iv 5.6.1 Method . 122 5.6.2 Results . 123 5.6.3 Discussion . 127 5.7 Overall Discussion . 127 5.7.1 Limitations . 128 5.7.2 Synthesizing Contradictory Results . 129 5.7.3 Social Communication Theories to Support Transparency . 130 5.7.4 Design Implications for Intelligent Systems . 131 5.7.5 Presenting Errors in Intelligent Systems . 132 6 How do users want to interact with transparency? 134 6.1 Introduction . 134 6.1.1 Contribution . 137 6.2 Research System: E-meter . 137 6.2.1 Machine Learning Model . 138 6.2.2 System Versions . 140 6.3 Study 1 . 141 6.3.1 Method . 142 6.3.2 Results . 143 6.3.3 Discussion . 148 6.4 Study 2 . 149 6.4.1 Method . 149 6.4.2 Results . 151 6.4.3 Discussion . 154 6.5 Discussion and Conclusions . 155 6.5.1 Meeting the Competing Needs of Transparency Through Progressive Disclosure . 156 6.5.2 Impact for Future Transparency Research . 160 6.5.3 Limitations . 160 6.5.4 Conclusion . 161 7 AI and Explanations in the Wild 162 7.1 Introduction . 162 7.2 Methods . 163 7.2.1 Predictive Analytics System . 164 7.2.2 Participants . 171 7.2.3 Materials . 172 7.2.4 Interview Questions . 173 7.3 Results . 175 7.3.1 Advisors Initially Evaluate System By Carefully Examining Students with Different Characteristics . 177 7.3.2 Transparency Can Polarize System Perceptions . 178 7.3.3 Explanations Undermine a System Prediction . 179 v 7.3.4 Progressive Disclosure Principles: Explaining Features . 180 7.3.5 Advisors Have Difficulty Understanding Engineered Features in Expla- nations . 181 7.3.6 Discrepancies Between Advisor and System Models of Student Perfor- mance . 182 7.3.7 Institutional Constraints the System Does Not Know . 183 7.3.8 Conflicting Explanation Goals . 184 7.3.9 Ethical Concerns About Using Predictive Analytics . 184 7.4 Discussion . 185 7.4.1 Error and Expectation Violation . 186 7.4.2 Systems Should Deliberately Walkthrough Users Initially . 186 7.4.3 Explanation Should Be Interactive . 187 7.4.4 Resolving Conflicts in Explanation Goals . 189 7.4.5 Conclusion . 190 8 Discussion 192 8.1 Summary . 192 8.2 Open Problems . ..
Details
-
File Typepdf
-
Upload Time-
-
Content LanguagesEnglish
-
Upload UserAnonymous/Not logged-in
-
File Pages228 Page
-
File Size-