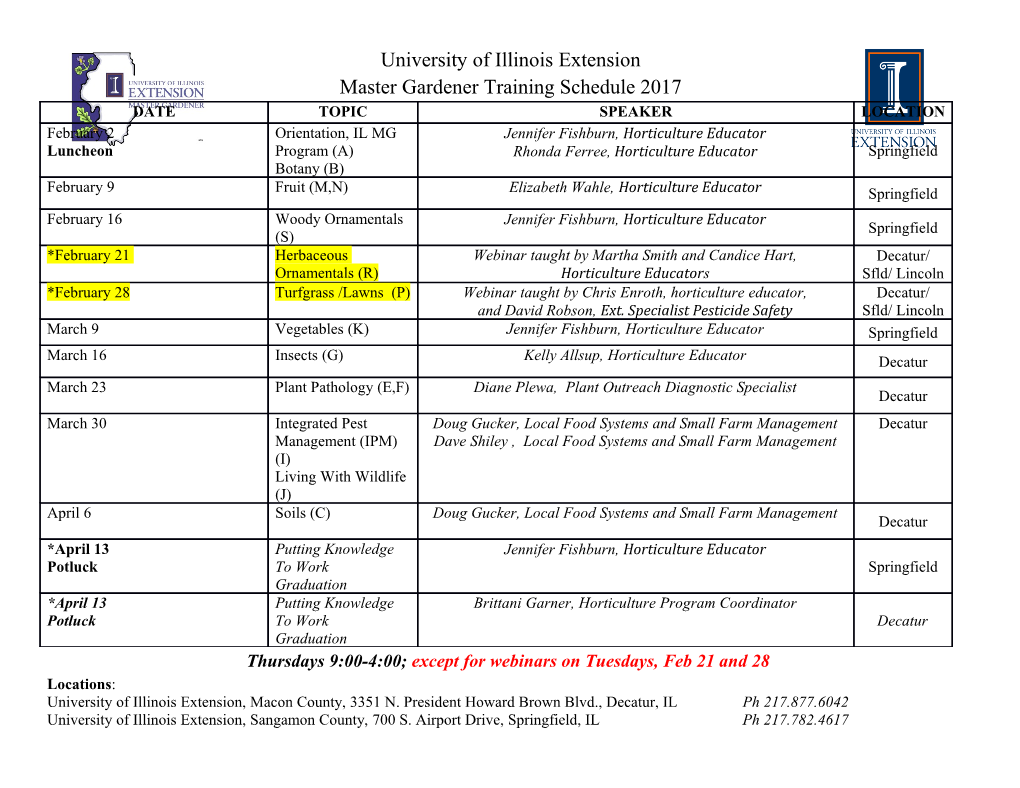
A FUZZY FRAMEWORK FOR ROBUST ARCHITECTURE IDENTIFICATION IN CONCEPT SELECTION A Thesis Presented to The Academic Faculty by Frank H. Patterson In Partial Fulfillment of the Requirements for the Degree Doctor of Philosophy in the School of Aerospace Engineering Georgia Institute of Technology December 2015 Copyright c 2015 by Frank H. Patterson A FUZZY FRAMEWORK FOR ROBUST ARCHITECTURE IDENTIFICATION IN CONCEPT SELECTION Approved by: Professor Daniel Schrage, Advisor Professor George Vachtsevanos School of Aerospace Engineering School of Electrical and Computer Georgia Institute of Technology Engineering Georgia Institute of Technology Professor Dimitri Mavris Dr. Sylvester Ashok School of Aerospace Engineering School of Aerospace Engineering Georgia Institute of Technology Georgia Institute of Technology Professor Brian German Date Approved: November 9, 2015 School of Aerospace Engineering Georgia Institute of Technology To my growing family, Love does multiply. iii ACKNOWLEDGEMENTS First and foremost I would like to thank Dr. Daniel Schrage for giving me the oppor- tunity to return to school for my graduate degrees. He gave me a chance when no one else would, and provided an opportunity that changed not just my career, but my entire life. Thank you for your continued support and encouragement. And many thanks to Bill McCandless for vouching for me yet again. I would also like to thank the rest of my committee for their time, help and guidance throughout this process. I owe so much to my amazing family for all they have provided me, and for their love, support and faith throughout my entire career (academic and otherwise). My deepest gratitude to my amazing wife, Martha, and my mother, without whose selfless sacrifice I would have never been able to even begin a PhD. And thank you to my son, Everett, who lights up my every day, no matter how long. In my seemingly short time in graduate school at Tech, I have been privileged to work with some of the best colleagues I've ever had. Thanks to the graduate students and research engineers (Rich, Nate, Sylvester, Mike M., Mike R., Kyle, and many, many more), who provided indispensable advice and insight on making it through this. I would also like to thank all of my labmates in my time with the IPLE, who have made my time there so enjoyable. iv TABLE OF CONTENTS DEDICATION .................................. iii ACKNOWLEDGEMENTS .......................... iv LIST OF TABLES ............................... viii LIST OF FIGURES .............................. x SUMMARY .................................... xvii I INTRODUCTION ............................. 1 1.1 Concept Selection . .6 1.1.1 Decision Matrices, Multi-Criteria Decision Making, and AHP9 1.1.2 Concept Selection Methods Accounting for Uncertainty . 11 1.1.3 Multi-Objective Decision Making/Optimization . 15 1.1.4 Other Related Methods . 17 1.2 Research Objectives . 19 1.3 Research Questions and Hypotheses . 25 1.3.1 Capturing Uncertainty in Value . 26 1.3.2 Generating and Evaluating Alternatives . 27 1.3.3 Making a Decision . 32 1.4 Research Methods . 35 II TOOLS .................................... 38 2.1 Fuzzy Set Theory . 38 2.1.1 Types of Fuzzy Sets . 39 2.1.2 Operations on Fuzzy Sets . 42 2.2 Fuzzy Systems . 48 2.2.1 Mamdani Fuzzy Model . 49 2.2.2 Larsen Fuzzy Models . 53 2.2.3 Other Fuzzy Models . 54 2.2.4 Neuro-Fuzzy Systems . 54 v 2.3 Multi-Criteria Decision Making . 56 2.3.1 Traditional AHP and TOPSIS . 58 2.3.2 Probabilistic Approaches to Traditional MADM . 62 2.3.3 Fuzzy Multi-Criteria Decision Making . 63 2.4 Computational Tools . 69 III RESEARCH ................................. 71 3.1 Benchmarking: Application of MADM . 72 3.1.1 Setting up the Problem . 72 3.1.2 Generating Benchmark Alternatives . 76 3.1.3 Evaluating the Alternatives . 79 3.1.4 Making a Decision . 83 3.1.5 Benchmarking Discussion . 93 3.2 Data Elicitation . 95 3.2.1 Gathering Architecture Input Data . 98 3.2.2 Gathering Architecture Performance Data . 100 3.2.3 Data Aggregation . 103 3.2.4 Physics-Based Data Generation . 106 3.2.5 Discussion . 116 3.3 Fuzzy System Models . 118 3.3.1 Fuzzy Rule Based System (FRBS) . 119 3.3.2 Fuzzy Error Functions . 124 3.3.3 Building a Simple FRBS . 126 3.3.4 Creation and Training of FRBSs from Expert Elicited Data . 129 3.3.5 Creation and Training of FRBSs from Physics-Based Data . 143 3.3.6 Neuro-Fuzzy Systems (NFS) . 145 3.3.7 Development of a DFES System for Physics-Based Data . 152 3.3.8 Development of a DFES System for Expert Elicited Data . 155 3.3.9 Additional Framework Fuzzy Models . 158 vi 3.3.10 Discussion . 161 3.4 Modeling Systems and Framework Implementation . 169 3.4.1 Identification of Pareto Optimal Architectures . 171 3.4.2 Fuzzy Possibility of Success (FPOS) Metric . 181 3.4.3 Decision Making and Implications . 190 3.4.4 Discussion . 197 3.5 Conclusions . 199 APPENDIX A | INPUT DATA ELICITATION SURVEY .... 204 APPENDIX B | SELECTED HISTORICAL DATA ........ 212 APPENDIX C | FUZZY SYSTEM DATA .............. 215 REFERENCES .................................. 250 vii LIST OF TABLES 1 Example Morphological Matrix for Notional Helicopter . .7 2 Fundamental Properties of Fuzzy Set Operations . 43 3 Example Fuzzy Arithmetic . 46 4 Customer Requirements (WHATs) . 75 5 Impact Relationship Values . 75 6 Engineering Characteristics (Criteria): Weights . 77 7 Morphological Matrix . 78 8 Alternatives 1-5 . 80 9 Alternatives 6-10 . 81 10 Ranking Results for AHP Methods with Crisp Measure (α = max(µ) Rank)................................... 84 11 Ranking Results for TOPSIS Methods with Crisp Measure (α = max(µ) Rank)................................... 85 12 Architectural Dependent (Morphological) Input Data . 101 13 Parameter Ranges: Figure of Merit Data . 109 14 RF Baseline Mission . 113 15 Parameter Ranges: RF Method Data . 114 16 Final Inputs for Empty Weight FRBS System . 134 17 Training MSE for Various System and Data Fuzzy Membership Functions135 18 Comparing Data Modeling Function Types for DFES Figure of Merit Systems . 153 19 Training Results for DFES Figure of Merit Systems . 154 20 Fit Statistics for DFES RF Systems . 160 21 NSGA-II Algorithm (from [32]) . 174 22 Optimization Parameters (NSGA-II) . 175 23 FPoS Optimization Parameters (NSGA-II) . 185 24 Identified Architecture Families . 193 25 New Alternatives 11-15 . 195 viii 26 Comparing Benchmarked Alternatives to Newly Identified Alternatives 196 ix LIST OF FIGURES 1 Phases of Design [74] . .2 2 Georgia Tech's Generic IPPD Process [97] . .3 3 Robust Design Simulation [97] . .4 4 Continuous Product Improvement [97] . .5 5 Interactive Reconfigurable Matrix of Alternatives (IRMA) [40] . .8 6 Analytic Hierarchy Process . 11 7 Concept Selection using JPDM [64] . 12 8 Development of an s-Pareto Frontier [71] . 13 9 Domain, Mapping, and Spaces in Axiomatic Design [114] . 18 10 Gaps in Current Concept Identification Methods . 20 11 Charting the Capabilities of Existing Concept Selection Methods (adapted from [82]) . 23 12 Generating Input Parameters for Expert Systems/Fuzzy Models) . 25 13 Examples of Fuzzification . 27 14 Example Database for an Inference System . 29 15 Notional Example of Mapping Historical First Order Design Parame- ters to Criteria (adapted from [31] . 32 16 Framework: Combining Fuzzy System with Decision Making Methods 33 17 Research Progression . 36 18 Types of Fuzzy Numbers . 42 19 Dominance in Fuzzy Sets . 47 20 Mamdani Fuzzy Inference (Single Input) . 51 21 Mamdani Fuzzy Inference (Multiple Inputs) . 52 22 Larsen Fuzzy Inference (Multiple Inputs) . 53 23 Simple Notional Neuro-Fuzzy System [81] . 56 24 Analytic Hierarchy Process . 59 25 Probabilistic AHP and TOPSIS Methodology . 63 x 26 QFD Relationship Matrix . 76 27 Weighting Methods Representing Uncertainty . 77 28 Combined Fuzzy Triangular and Trapezoidal Scores as Generated from Expert Elicitation . 82 29 Comparison of Uncertainy in AHP Results (Triangular Inputs) . 87 30 Comparison of Uncertainy in TOPSIS Results (Triangular Inputs) . 88 31 AHP Results (Triangular Inputs): Probability of Ranking in the Top N Alternatives . 89 32 TOPSIS Results (Triangular Inputs): Probability of Ranking in the Top N Alternatives . 89 33 AHP Ranking Possibility Matrix (Triangular Inputs) . 91 34 TOPSIS Ranking Possibility Matrix (Triangular Inputs) . 92 35 Probability of Alternative Being in the Top 4 with N Alternatives Re- moved for Probabilistic AHP(a) and TOPSIS(b) . 92 36 Possibility of Ranking as Top Alternative with N Alternatives Removed for Fuzzy AHP(a) and TOPSIS(b) . 93 37 Online Concept Evaluation Tool . 102 38 Selected Examples of Expert Evaluation (Empty Weight Ratio) with Pseudo-Random Experts and Data Combination Examples . 104 39 Means of Fuzzifying Expert Evaluations for 3 Example Evaluations . 106 40 Expanded RF Core Algorithm . 110 41 RF Method Validation . 111 42 Output Membership Functions for Installed Power Training Data from RF Algorithm . 114 43 Generated Data from RF Algorithm . 115 44 Accuracy vs. Complexity in a FRBS (from [28]) . 122 45 Examples of Fuzzy Error Methods for Various Membership Functions 125 46 L/D Expert System Architecture . 127 47 Inference Mechanics of the Example L/D FRBS for a Specific Example (Alternative 1: Variable RPM Tilt-Rotor) . 128 48 Performance of L/D System for Baseline Alternatives (α-cut at max(µ)), with % Overlap Shown. 129 xi 49 Effects of Training Data Set Size on Baseline FRBS for φ (Min, Mean, Max) . ..
Details
-
File Typepdf
-
Upload Time-
-
Content LanguagesEnglish
-
Upload UserAnonymous/Not logged-in
-
File Pages279 Page
-
File Size-