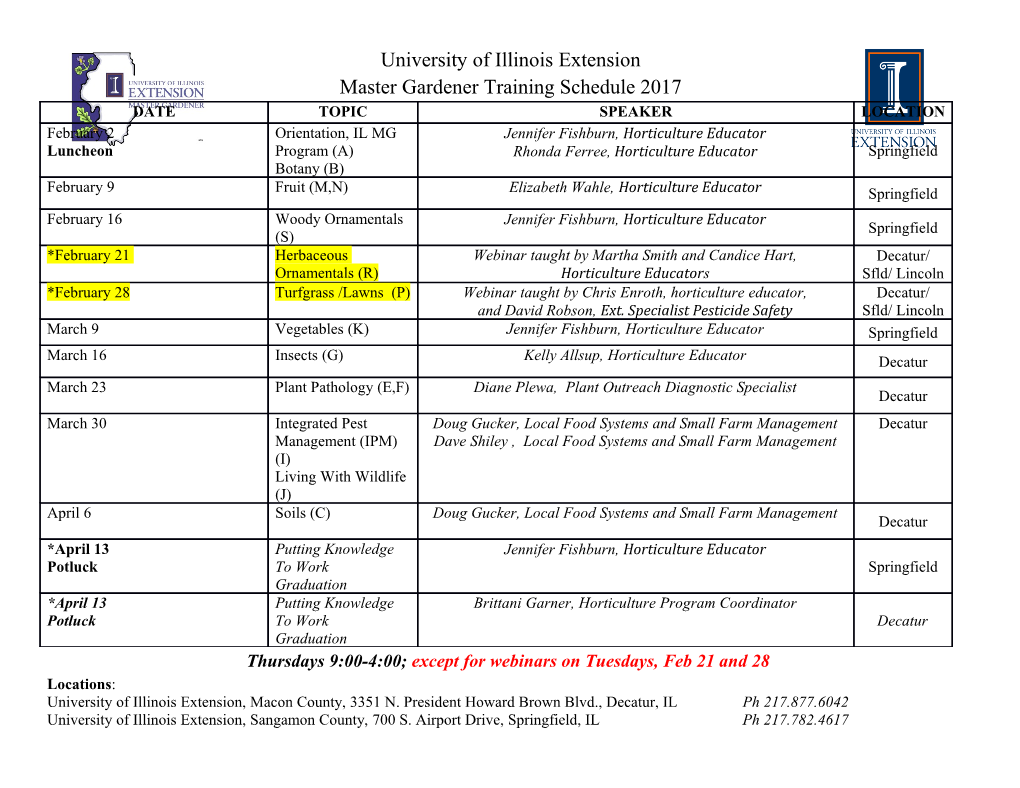
University of Vermont ScholarWorks @ UVM Graduate College Dissertations and Theses Dissertations and Theses 2018 Fluvial Processes in Motion: Measuring Bank Erosion and Suspended Sediment Flux using Advanced Geomatic Methods and Machine Learning Scott ouD glas Hamshaw University of Vermont Follow this and additional works at: https://scholarworks.uvm.edu/graddis Part of the Computer Sciences Commons, Environmental Engineering Commons, and the Hydrology Commons Recommended Citation Hamshaw, Scott ouD glas, "Fluvial Processes in Motion: Measuring Bank Erosion and Suspended Sediment Flux using Advanced Geomatic Methods and Machine Learning" (2018). Graduate College Dissertations and Theses. 827. https://scholarworks.uvm.edu/graddis/827 This Dissertation is brought to you for free and open access by the Dissertations and Theses at ScholarWorks @ UVM. It has been accepted for inclusion in Graduate College Dissertations and Theses by an authorized administrator of ScholarWorks @ UVM. For more information, please contact [email protected]. FLUVIAL PROCESSES IN MOTION: MEASURING BANK EROSION AND SUSPENDED SEDIMENT FLUX USING ADVANCED GEOMATIC METHODS AND MACHINE LEARNING A Dissertation Presented by Scott D. Hamshaw to The Faculty of the Graduate College of The University of Vermont In Partial Fulfilment of the Requirements For the Degree of Doctor of Philosophy Specializing in Civil and Environmental Engineering January, 2018 Defense Date: September 12, 2017 Dissertation Examination Committee: Donna M. Rizzo, Ph.D., Advisor Andrew W. Schroth, Ph.D., Chairperson Mandar M. Dewoolkar, Ph.D. Beverley C. Wemple, Ph.D. Arne Bomblies, Ph.D. Jarlath O’Neil-Dunne, M.S. Cynthia J. Forehand, Ph.D., Dean of the Graduate College ABSTRACT Excessive erosion and fine sediment delivery to river corridors and receiving waters degrade aquatic habitat, add to nutrient loading, and impact infrastructure. Understanding the sources and movement of sediment within watersheds is critical for assessing ecosystem health and developing management plans to protect natural and human systems. As our changing climate continues to cause shifts in hydrological regimes (e.g., increased precipitation and streamflow in the northeast U.S.), the development of tools to better understand sediment dynamics takes on even greater importance. In this research, advanced geomatics and machine learning are applied to improve the (1) monitoring of streambank erosion, (2) understanding of event sediment dynamics, and (3) prediction of sediment loading using meteorological data as inputs. Streambank movement is an integral part of geomorphic changes along river corridors and also a significant source of fine sediment to receiving waters. Advances in unmanned aircraft systems (UAS) and photogrammetry provide opportunities for rapid and economical quantification of streambank erosion and deposition at variable scales. We assess the performance of UAS-based photogrammetry to capture streambank topography and quantify bank movement. UAS data were compared to terrestrial laser scanner (TLS) and GPS surveying from Vermont streambank sites that featured a variety of bank conditions and vegetation. Cross-sectional analysis of UAS and TLS data revealed that the UAS reliably captured the bank surface and was able to quantify the net change in bank area where movement occurred. Although it was necessary to consider overhanging bank profiles and vegetation, UAS-based photogrammetry showed significant promise for capturing bank topography and movement at fine resolutions in a flexible and efficient manner. This study also used a new machine-learning tool to improve the analysis of sediment dynamics using three years of high-resolution suspended sediment data collected in the Mad River watershed. A restricted Boltzmann machine (RBM), a type of artificial neural network (ANN), was used to classify individual storm events based on the visual hysteresis patterns present in the suspended sediment-discharge data. The work expanded the classification scheme typically used for hysteresis analysis. The results provided insights into the connectivity and sources of sediment within the Mad River watershed and its tributaries. A recurrent counterpropagation network (rCPN) was also developed to predict suspended sediment discharge at ungauged locations using only local meteorological data as inputs. The rCPN captured the nonlinear relationships between meteorological data and suspended sediment discharge, and outperformed the traditional sediment rating curve approach. The combination of machine-learning tools for analyzing storm-event dynamics and estimating loading at ungauged locations in a river network provides a robust method for estimating sediment production from catchments that informs watershed management. CITATIONS Material from this dissertation has been published in the following form: Hamshaw, S. D., Bryce, T., Rizzo, D. M., O'Neil‐Dunne, J., Frolik, J., & Dewoolkar, M. M.. (2017). Quantifying streambank movement and topography using unmanned aircraft system photogrammetry with comparison to terrestrial laser scanning. River Research and Applications. doi: 10.1002/rra.3183 AND Material from this dissertation has been submitted for publication to Geomatics, Natural Hazards, and Risk on October 31, 2017 in the following form Hamshaw, S.D., Engel, T., Rizzo, D.M., O’Neil-Dunne, J., & Dewoolkar, M. M.. (2017). Application of unmanned aircraft system (UAS) for monitoring bank erosion along a river corridor. Geomatics, Natural Hazards and Risk. AND Material from this dissertation has been submitted for publication to Hydrological Processes on November 5, 2017 in the following form Hamshaw, S.D. and Rizzo, D.M.. (2017). Predicting river suspended sediment loadings using hydrometeorological variables and counterpropagation neural networks. Hydrological Processes. AND Material from this dissertation has been submitted for publication to Water Resources Research on November 13, 2017 in the following form Hamshaw, S.D., Dewoolkar, M.M., Schroth, A.W., Wemple, B.C., and Rizzo, D.M.. (2017). A new machine-learning approach for classifying hysteresis in suspended-sediment discharge relationships using high-frequency monitoring data. Water Resources Research. ii ACKNOWLEDGEMENTS This body of research would not have been possible nor successful without the assistance, guidance, and encouragement from many people. I would like to first acknowledge my committee for their guidance, encouragement, and interest during the development of this dissertation. Thank you to Andrew Schroth, Jarlath O’Neil-Dunne, Arne Bomblies, and Beverley Wemple –your research endeavors at UVM are the reason why UVM is an inspiring place to do research. Thanks to my unofficial co-advisor Mandar Dewoolkar, whose support and knowledge in my research was greatly appreciated and whose combination of positive attitude and efficient approach made finishing this dissertation possible. Finally, a special thank you to my advisor, Donna Rizzo, who was unwavering in offering guidance, encouragement, and who, no matter the seemingly impossible deadlines, was dedicated to giving valuable feedback. In the fifteen years I have known her at UVM, she has been continuously committed to supporting in achieving my personal and career goals, and it was no different with supporting my teaching and research during my PhD program. I was fortunate to be able to work with many terrific undergraduate interns and fellow graduate students in conducting this research and it would not have been possible without them. Thank you to interns Alex Morton, Hanna Anderson, Kira Kelley, Anna Waldron, Jordan Duffy, Nathalie Simoes, and Wimara Rubia Sa Gomes for their help in collecting field data. Sincere thanks to fellow graduate students Thomas Bryce, Jody Stryker, Justin Guilbert, John Hanley, Nikos Fytilis, and Ian Anderson for their collaboration and contributions in data collection and feedback on project design. A special thank you to Kristen Underwood whose collaboration was especially valued during project design, data collection and analysis phases. iii A number of programs and labs at UVM provided support for this project, in particular for field data collection. Thank you to EPSCoR RACC project staff Katie Chang, Janel Roberge, Saul Blocher, and Pat Clemins for their work on testing water quality samples and supporting data management. The UVM Spatial Analysis Lab (SAL), and in particular Tayler Engel and the UAS team, contributed significantly to data collection and processing. I would also acknowledge the contributions from Beverley Wemple, Jeff Frolik, Don Ross, and Breck Bowden’s labs and their many graduate students and interns that I collaborated with on fieldwork and data collection. I am especially grateful for the contributions of a number of people in the larger community that both helped guide this research and made it possible to undertake. A number of landowners in the Mad River Valley were remarkably accommodating of having myself and other students setting up monitoring stations on their property and helping to keep it safe. Thanks to the Friends of the Mad River for their support of all the UVM research undertaken in the Mad River Valley. This research would not have been possible without the funding and support provided by the National Science Foundation (NSF), Vermont EPSCoR, the Vermont Water Resources and Lake Studies center, and the Robert and Patricia Switzer Foundation. Finally, thank you to my friends and family who both supported
Details
-
File Typepdf
-
Upload Time-
-
Content LanguagesEnglish
-
Upload UserAnonymous/Not logged-in
-
File Pages307 Page
-
File Size-