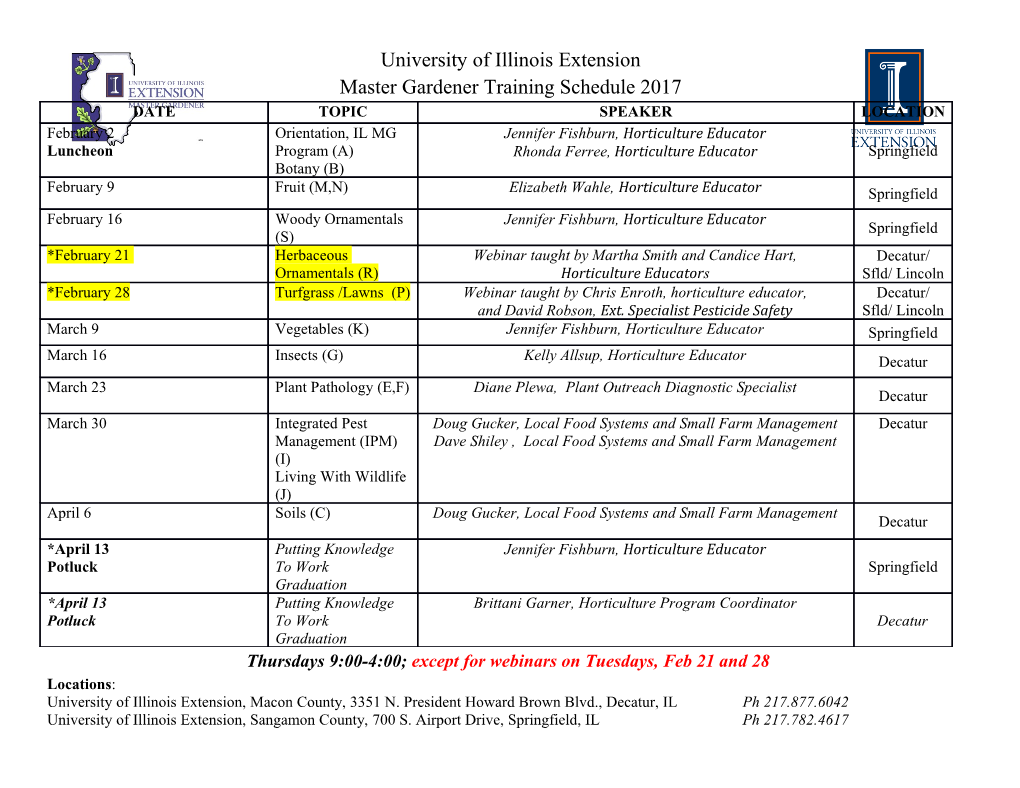
Top-down effect of apparent humanness on vocal alignment toward human and device interlocutors Georgia Zellou ([email protected]) Phonetics Lab, Department of Linguistics, UC Davis, 1 Shields Avenue Davis, CA 95616 USA Michelle Cohn ([email protected]) Phonetics Lab, Department of Linguistics, UC Davis, 1 Shields Avenue Davis, CA 95616 USA Abstract (Bentley et al., 2018). Current voice-AI technology has advanced dramatically in recent years; common systems can Humans are now regularly speaking to voice-activated artificially intelligent (voice-AI) assistants. Yet, our now generate highly naturalistic speech in a productive way understanding of the cognitive mechanisms at play during and respond to spontaneous verbal questions and commands speech interactions with a voice-AI, relative to a real human, by users. In some speech communities, voice-AI systems interlocutor is an understudied area of research. The present are omni-present and used on a daily basis to perform a study tests whether top-down guise of “apparent humanness” variety of tasks, such as send and read text messages, make affects vocal alignment patterns to human and text-to-speech phone calls, answer queries, set timers and reminders, and (TTS) voices. In a between-subjects design, participants heard either 4 naturally-produced or 4 TTS voices. Apparent control internet-enabled devices around the home (Hoy, humanness guise varied within-subject. Speaker guise was 2018). Despite the prevalence of voice-AI, our scientific manipulated via a top-down label with images, either of two understanding of the socio-cognitive mechanisms shaping pictures of voice-AI systems (Amazon Echos) or two human human-device interaction is still limited. Specifically, as talkers. Vocal alignment in vowel duration revealed top-down humans engage in dyadic speech behaviors with these non- effects of apparent humanness guise: participants showed human entities, how are our speech behaviors toward them greater alignment to TTS voices when presented with a device similar or dissimilar from how we talk with humans? In this guise (“authentic guise”), but lower alignment in the two inauthentic guises. Results suggest a dynamic interplay of paper, we examine the effect of apparent “humanness” bottom-up and top-down factors in human and voice-AI category — i.e., top-down information that the interlocutor interaction. is a device or human — on people’s vocal alignment toward text-to-speech (TTS) synthesized and naturally-produced Keywords: vocal alignment; apparent guise; voice-activated artificially intelligent (voice-AI) systems; human-computer voices. interaction Computer personification On the one hand, classic theoretical frameworks of Introduction technology personification, such as the “Computers are social actors” (CASA) theory (Nass et al., 1997), Humans use speech as a way of conveying our abstract hypothesize that our attitudes and behavior toward thoughts and intentions. Yet, speech is more than just a technological agents mirror those toward real human signal to emit words and phrases; our productions are interactors. This has been tested by examining whether shaped by intricate cognitive and social processes social patterns of behavior observed in human-human underlying and unfolding over the interaction. One large interaction apply when people interact with a computer. For mediator of these processes is who we are talking to: their instance, people’s responses to questions vary based on the social characteristics (e.g., gender in Babel, 2012), social characteristics of the interviewer; for example, biases humanness (e.g., computer or human in Burnham et al., in responses are observed based on the gender and ethnicity 2010), and even our attitudes toward our interlocutors (e.g., of the (human) interviewer (Athey et al., 1960; Hutchinson speech accommodation in Chakrani, 2015) can shape our & Wegge, 1991). This phenomenon has been described as a productions. Examining speech behavior can serve as a social desirability effect: people seek to align their window into cognitive-social dimensions of communication responses to the perceived preferences of their interviewer and is particularly relevant for examining different types of because to do otherwise would be impolite (Finkel et al., interlocutors. 1991). Nass and colleagues (1999) examined how people Recently, humans have begun interacting with voice- apply this politeness pattern to interactive machines. After activated artificially intelligent (voice-AI) assistants, such as performing a task (either text- or voice-based) with a Amazon’s Alexa and Apple’s Siri. For example, over the computer system, consisting of a tutoring session by the past several years, tens of millions of “smart speakers” have computer on facts about culture, and then subsequently been brought into people’s homes all over the world being tested on those facts, participants were asked 3490 ©2020 The Author(s). This work is licensed under a Creative Commons Attribution 4.0 International License (CC BY). questions about the performance of the computer. There humanness can speak to the impact of both bottom-up were two critical conditions: either the same computer that (acoustic) and top-down (guise) factors on speech behavior performed the tutoring asked for an evaluation of its toward humans and voice-AI: if it is driven by the performance as a tutor, or the evaluation of that computer characteristics of the speech (e.g., naturally produced vs. was solicited by a different computer located in another TTS) and/or by the extent to which we “believe” we are room. Nass and colleagues found that people provided more interacting with a human or a device voice. At the same positive evaluations in the same-computer condition, time, presenting an inauthentic top-down label while relative to the different-computer condition, indicating that presenting the original audio (e.g., “human” label with a the social desirability effect applies when we interact with a TTS voice, and vice versa) results in cue incongruency. In machine. From empirical observations such as this, the the present study, we test whether a match or mismatch in CASA framework argues that our interactions with voice (real human vs. TTS) and image (human vs. device) computers include a social component, positing that social shape speech behavior — and whether speakers are more responses to computers are automatic and unconscious sensitive to incongruous cues by guise: apparent human vs. especially when they display cues associated with being apparent device talker. human, in particular “the use of language” (Nass et al., While prior work has examined using moving computer 1999, p. 1105, emphasis ours). avatars (e.g., Burnham et al., 2010; Heyselaar et al., 2017), Yet, the extent to which individuals display differences in most modern voice-AI systems lack an avatar representation their socio-cognitive representations of real human versus (i.e., “Siri” and “Alexa” have no faces). As such, we ask computer interlocutors has resulted in mixed findings in the whether presenting a guise via images (either of a device or literature. For example, studies manipulating interlocutor a human face) can trigger differences in speech behavior guise – as human or computer – are one way to compare toward AI. Prior matched guise experiments have found that these interactions while holding features of the interaction, participants’ perception of a given voice shifts according to such as error rate and conversational context, constant. For minimal social information available. For example, seeing a example, Wizard-of-Oz studies, where a human photo depicting speakers of various ages and socio- experimenter is behind different interactions that are economic statuses impacts how listeners categorize the same apparently with either a “computer system” or a “real set of vowels (Hay et al., 2006). Thus, in the present study person”, demonstrate that people do produce different we predict that top-down influences (here, images of voice- speech patterns toward humans and technological systems. AI devices and human faces), will impact how participants In particular, Burnham and colleagues (2010) found respond to identical stimuli. In particular, we hypothesize increased vowel space hyperarticulation and slower that participants will display different speech patterns productions in speech toward an apparent computer avatar, toward the voices labeled as “devices” compared to relative to apparent human interlocutor; this suggests that “humans”. people have distinct representations for the two interlocutor types, computer versus human. Others have observed Vocal alignment greater overlap: Heyselaar and colleagues (2017) found In the current study, to test these questions, we examine similar priming effects for real humans and human-like vocal alignment, a subconscious, yet pervasive, speech avatars (presented in virtual reality, VR); yet, they behavior in human interactions, while varying top-down additionally found less priming for the computer avatar in guise (“human” or “device”). Vocal alignment is the general. Together, these results suggest that people’s phenomenon whereby an individual adopts the subtle cognitive representations for humans and computerized acoustic properties of their interlocutor’s speech during interlocutors appear to be different and, further, they may be verbal exchanges. Vocal alignment appears to be, to some gradiently, rather than categorically, distinct. extent, an automatic behavior, argued
Details
-
File Typepdf
-
Upload Time-
-
Content LanguagesEnglish
-
Upload UserAnonymous/Not logged-in
-
File Pages7 Page
-
File Size-