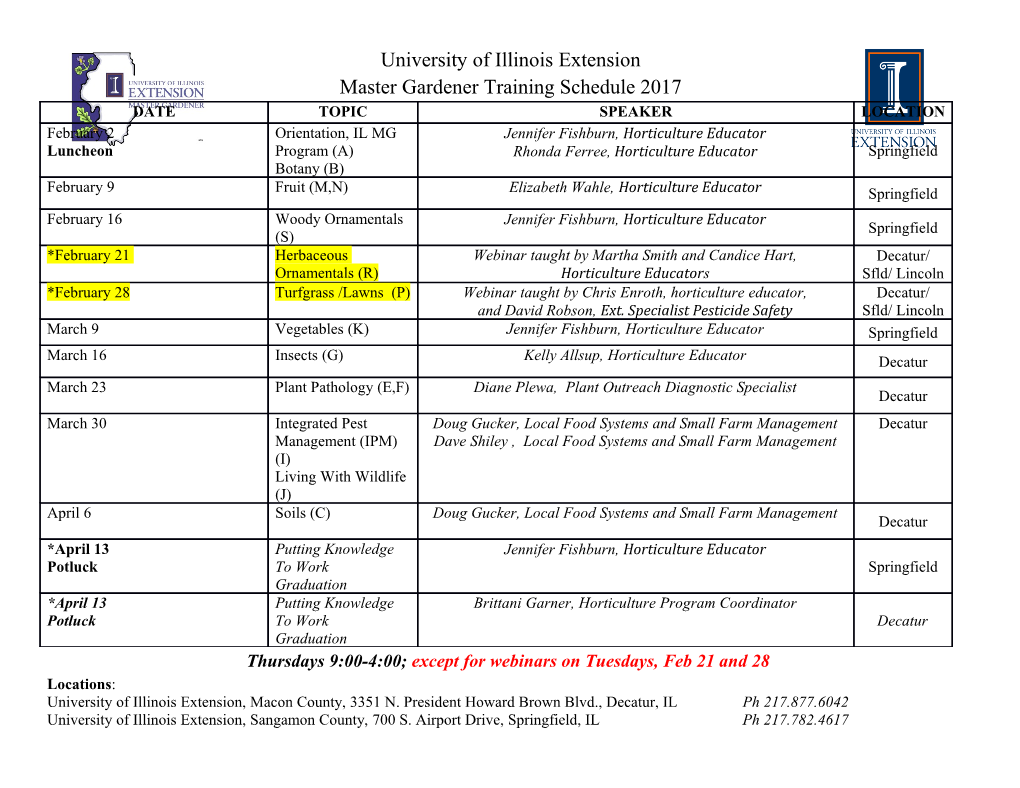
Epidemiol. Methods 2021; 10(s1): 20200047 Mauro Magnoni* The first diffusion of the Covid-19 outbreak in Northern Italy: an analysis based on a simplified version of the SIR model https://doi.org/10.1515/em-2020-0047 Received October 29, 2020; accepted March 10, 2021; published online March 25, 2021 Abstract: In this paper an analysis of the first diffusion of the Covid-19 outbreak occurred in late February 2020 in Northern Italy is presented. In order to study the time evolution of the epidemic it was decided to analyze in particular as the most relevant variable the number of hospitalized people, considered as the less biased proxy of the real number of infected people. An approximate solution of the infected equation was found from a simplified version of the SIR model. This solution was used as a tool for the calculation ofthe basic reproduction number R0 in the early phase of the epidemic for the most affected Northern Italian regions (Piedmont, Lombardy, Veneto and Emilia), giving values of R0 ranging from 2.2 to 3.1. Finally, a theoretical formulation of the infection rate is proposed, introducing a new parameter, the infection length, characteristic of the disease. Keywords: approximate solution; infectious lenght; SIR model. Introduction A sudden increase of cases of Covid-19 diseases originated by the new coronavirus SARS-CoV-2 struck Northern Italy and Lombardy in particular,inlate February 2020 (Distante et al. 2020; Ital- ian National Institute of Health (ISS); Italian Ministry of Health). The rapid growth of many severe illnesses leads to a dramatic pressure on the hospitals, particularly in the intensive care units. In a few weeks thousands of people were hospitalized and roughly an equal number of persons found positive to the tests and with only mild symptoms, were quarantined at home. However, this was just the tip of the iceberg: the estimated incubation period of about 5 days but with a quite large range (2–14 days) (Lauer et al. 2020) clearly promotes the diffusion of the outbreak and, as a consequence of that, it was very soon clear that also mild, asymptomatic and pre-symptomatic cases played a significant role in the rapid surge of the epidemicLi ( et al.). This fact wasconfirmedbymanyotherstudies(Gandhi et al. 2020) where also negative serial intervals SI1 have been reported (Du et al. 2020). Unfortunately, the lack of a quick availability of a large number of test kits prevented to know the real dimension of the outbreak diffusion. For that reason, the reliability of the official data given by the authorities was questioned: the number of people found positive was of course greatly influenced by the number of tests performed and, more importantly, the criteria followed to submit people to the tests, especially in the first weeks after the beginning of the outbreak, were not completely consistent in space and time. It is thus certain that the number of the infected people officially declared doesn’t reflect the real number of infected individuals in the population. Nevertheless, these limitations don’t prevent the possibility of using the official data for some analyses. The most interesting features of the dynamics of an outbreak can be investigated studying the variations of the relevant variables, irrespective of their precise and “real” values. 1 The serial interval SI is the time between a primary case-patient (infector) and a secondary case-patient (infectee). *Corresponding author: Mauro Magnoni, ARPA Piemonte, Department of Physical and Technological Risks, Via Jervis, 30, 10015 Ivrea (TO), Italy, E-mail: [email protected] 2 | Magnoni: Covid-19 in Northern Italy: a SIR model based analysis Figure 1: The Northern regions of Italy most affected by the Covid-19 outbreak: Piedmont, Lombardy, Emilia–Romagna and Veneto. The official data were given on a daily basis and for each Italian region bythe(Ital- ian Department of Civil Protection). In this study the following data were considered: the number of infected people, unfortunately greatly affected by the number of tests performed, the number of hospitalized people and the number of people admitted in the intensive care units. In order to study the time evolution of the epidemic we decided to use as relevant variable, besides the number of infected people, also the number of the hospitalized, considered as a less biased proxy of the real number of infected people, the latter being potentially influenced by the unknown number of undetected cases. The rationale of this choice is that the number of hospitalized patients depends essentially on the epidemic dynamics and should not be greatly affected by some “external factors” such as, for example, the varying availability of tests and the changing of policies of the health authorities during the emergency. In doing so, there are of course some very important underlying assumptions, the most important one is that the susceptibility to the disease within the affected population is assumed consistent in space and time. This condition is certainly not completely true, as it is well known that the susceptibility of a given population to this disease is largely influenced by its age structure and density. A correction for these effects are possible in principle, provided that detailed information on the age structure of the populations and their densities are taken into account. However, it was decided to not include these aspects in this preliminary work: the data were taken as they are and the results were discussed. Nevertheless, no significant biases are expected due to the lack of consideration of the population ages structure because their distributions are quite similar within the considered Italian regions. All data are interpreted in the light of the SIR model (Kermack and McKendrick 1927), i.e. the standard model describing the evolution of the outbreaks in term of susceptible people (S), the infected (I) and the recovered (R) ones, presented here in an approximate and simplified form. As the Covid-19 epidemic affects Italy very unevenly, with the Northern regions much more affected than the rest of the country, this work is mainly focused on the most affected ones, in particular: Lombardy, Piedmont, Emilia–Romagna and Veneto (Figure 1). Sicily was also considered for the sake of comparison, as a typical representative of the Southern regions. Magnoni: Covid-19 in Northern Italy: a SIR model based analysis | 3 Theoretical background The standard SIR model, first proposed in 1927 by Kermack and McKendric (Kermack and McKendrick 1927), is a model described by a system of three non linear differential equation connecting three compartments representing the stocks of the susceptible population (S), the infected (I) and the recovered (R) ones. The mathematical formulation of the model is shown in the following, in which x(t) represents the susceptible population, y(t)theinfected,z(t) the recovered, while the parameters and are respectively the infection rate and the recovery rate2: dx (t) =− ⋅ x (t) ⋅ y (t) (1a) dt N dy(t) = ⋅ x (t) ⋅ y (t) − ⋅ y (t) (1b) dt N dz (t) = ⋅ y (t) (1c) dt In these equations N is a constant, representing the total number of people that can be potentially involved (fixed population). The boundary conditions of the system are generically given by: x(0) = N1; y(0) = N2; z(0) = N3, with the following constraint, N = N1 + N2 + N3. The dynamics of the infection is governed by the ratio R0 = / , the so called basic reproduction number, the parameter usually utilized for the description of the diffusion of an epidemic: the greater is R0, the greater and faster is the spread of the outbreak, while a < value of R0 1 leads to the extinction of the disease. However, while is a constant depending essentially on the medical characteristics of the disease and, for that reason, can’t be substantially affected by actions taken during an epidemic, the reduction ofthe R0 value can be achieved only by lowering the infection rate , usually done introducing measures such as social distancing. This kind of measures were actually taken by the Italian government, in particular from the 8th March (DPCM 2020): a strict lockdown was imposed all over Italy in the effort to reduce the spread of the disease in the whole country. Going back to the theoretical framework, it can be observed that the solutions of the equations system are usually performed numerically using different mathematical approaches (Biazar 2006; Rafei et al. 2007a, 2007b). An exact analytical solution of the SIR equations was quite recently (2014) proposed in a parametric form (Harko et al. 2012). However, all these methods are not easy to be handled and require some technicalities not always easily available among many researchers working in the medical field. For those reasons in this work we will derive an approximate analytical solution of the system, giving very simple formulas much easier to be used and applied to real conditions. The basic assumption we made was substituting at the right side of the first Eq. (1a),thetimevarying function y(t), representing the infected population with its average value ȳ,calculatedinthetimerange during which the outbreak develops, namely: Δ 1 ⋅ y = ∫ y (t) dt Δ 0 The first Eq. (1a) can thus be rewritten in the following form: dx (t) =− ⋅ x (t) ⋅ y dt N that is straightforward integrated giving the following expression: − ⋅y⋅t ⋅ N x (t) = x0 e 2 It is worth to note that in this model the parameter in the equation represents more precisely the rate of removal from the infected compartment rather than a real recovery rate. Actually, a complete recovery in a clinical sense may take more time and does not coincide with the time lag during which the infected patient is capable to spread the disease.
Details
-
File Typepdf
-
Upload Time-
-
Content LanguagesEnglish
-
Upload UserAnonymous/Not logged-in
-
File Pages13 Page
-
File Size-