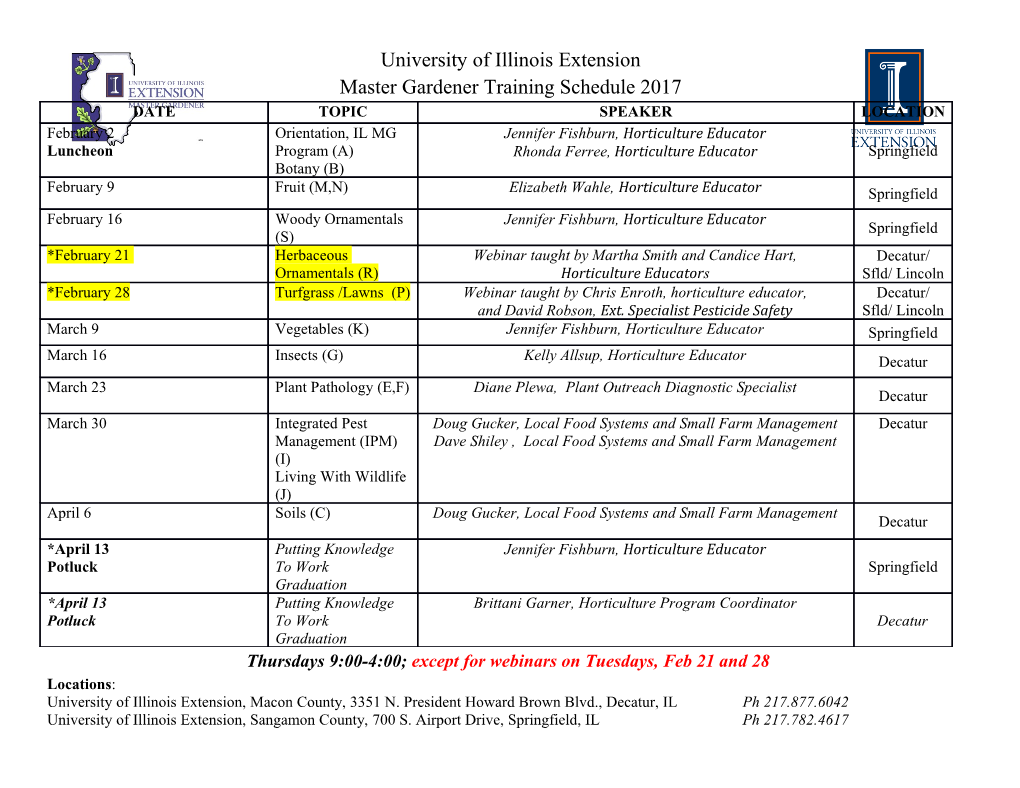
Bayesian SAE using Complex Survey Data Lecture 5A: Survey Sampling Jon Wakefield Departments of Statistics and Biostatistics University of Washington 1 / 119 Outline Overview Design-Based Inference Simple Random Sampling Stratified Simple Random Sampling Cluster Sampling Multistage Sampling Discussion Technical Appendix: Simple Random Sampling Technical Appendix: Stratified SRS Technical Appendix: Cluster Sampling Technical Appendix: Lonely PSUs 2 / 119 Overview 3 / 119 Outline Many national surveys employ stratified cluster sampling, also known as multistage sampling, so that’s where we’d like to get to. In this lecture we will discuss: I Simple Random Sampling (SRS). I Stratified SRS. I Cluster sampling. I Multistage sampling. 4 / 119 Main texts I Lohr, S.L. (2010). Sampling Design and Analysis, Second Edition. Brooks/Cole Cengage Learning. Very well-written and clear mix of theory and practice. I Lumley, T. (2010). Complex Surveys: A Guide to Analysis Using R, Wiley. Written around the R survey package. Great if you already know a lot about survey sampling. I Korn, E.L. and Graubard, B.I. (1999). Analysis of Health Surveys. Wiley. Well written but not as comprehensive as Lohr. I Sarndal,¨ Swensson and Wretman (1992). Model Assisted Survey Sampling. Springer. Excellent on the theory though steep learning curve and hard to dip into if not familiar with the notation. Also, anti- model-based approaches. 5 / 119 The problem We have a question concerning variables in a well-defined finite population (e.g., 18+ population in Washington State). What is required of a sample plan? We want: I Accurate answer to the question (estimate). I Good estimate of the uncertainty of the estimate (e.g., variance). I Reasonable cost for the study (logistics). We may be interested in this particular finite population only, or in generalizing to other populations/situations, i.e., the process. If the former, then if we sample the complete population, we are done! No statistics needed... 6 / 119 The problem A simple random sample (SRS) is almost always better than a non-random sample, because the former allows more allows an assessment of uncertainty. We will focus on design-based inference: in this approach the population values of the variable of interest, y1;:::; yN are viewed as fixed, what is random is the indices of the individuals who are sampled. This approach is frequentist, so that properties are based on hypothetical replications of the data collection process; hence, we require a formal description of the replication process. A complex random sample may be: I better than a SRS in the sense of obtaining the same precision at lower cost, I but may be worse in the sense of precision, but be required logistically. 7 / 119 Domains Often estimation is required for sub-populations of interests, these are known as domains. Often the decision to study the domain occurs after the design, and so the sample sizes in the domains are random, and may be small. If the domains are defined geographically, then inference for these domains is known as small area estimation (SAE). Domains may also be defined as socio-demographic groups and trying to obtain sufficient samples in some domains (e.g., based on race), may lead to small sample sizes in others (e.g., states). 8 / 119 Probability samples Notation for random sampling, in a single population (and not distinguishing areas): I N, population size. I n sample size. I πk , sampling probability for a unit (which will often correspond to a person) k, k = 1;:::; N. Random does not mean “equal chance”, but means that the choice does not depend on variables/characteristics (either measured or unmeasured), except as explicitly stated1 via known sampling probabilities. In a simple random sample, the sampling probabilities are all equal, n π = : k N 1For example, in stratified random sampling, certain groups may have fixed numbers sampled. 9 / 119 Probability samples For design-based inference, which we shall discuss in detail: I To obtain an unbiased estimator every individual k in the population to have a non-zero probability of being sampled; this probability will be defined as πk , for k = 1;:::; N. I To carry out inference, this probability πk must be known for every individual in the sample (so not needed for the unsampled individuals). I To obtain a form for the variance of an estimator: for every pair of individuals, k and l, in the sample, there must a non-zero probability of being sampled together, call this probability, πkl , for k = 1;:::; N, l = 1;:::; N, k 6= l. 2 I The probability πkl must be known for every pair in the sample . Lower case values will denote population values, y1;:::; yN . 2in practice, these are often approximated, or the variance is calculated via a resampling technique such as the jackknife 10 / 119 Probability samples The label probability sample is often used instead of random sample. Non-probability sampling approaches include I convenience (accidental, haphazard) sampling (e.g., asking for volunteers); I purposive (also known as judgmental) sampling in which a researcher users their subject knowledge to select participants (e.g, selecting an “average” looking individual). I Quota sampling in which quotas in different groups are satisfied (but unlike stratified sampling, probability sampling is not carried out). Non-probability samples cannot be analyzed with design-based approaches, because there is no πk or πkl . 11 / 119 Representative samples Surveys are broadly of two types: questionnaire and interview. A fundamental concept in sampling is whether the sample is representative. There is no perfect, “scaled down” version of the population for whom we would like to make inference is available. Lohr (2010, p. 3) says, “...a good sample will be representative in the sense that characteristics of interest in the population can be estimated from the sample with a known degree of accuracy”. Post-stratification and raking are techniques for making a sample more representative. 12 / 119 When does having a representative sample matter? Inference for population quantities such as means, totals, medians, etc., are not reliable except with random samples. Estimation of relationships between variables, for example, whether a diet high in salt increases blood pressure, can often be estimated from non-random samples, with careful modeling — called model-based analysis. 13 / 119 Definitions: Based on Lohr (2010, Section 1.2). Definitions to allow the idea of a good sample to be make precise: I Observation unit: An object on which a measurement is taken, sometimes called an element. In human populations, observation units are individuals. I Target population: The complete collection of observations we want to study. I Sample: A subset of a population. I Sampled population: The collection of all possible observation units that might have been chosen in a sample; the population from which the sample was chosen. The sampled population will often not correspond to the target population; it may be a more accessible version for example. 14 / 119 Definitions I Sampling unit: A unit that can be selected for a sample. Although we might want to study individuals, we may not have a list of individuals in the target population. For example, households may serve as the sampling units, with the individuals in the household being the observation units. I Sampling frame: A list, map or other specification of sampling units in the population from which a sample may be selected. i.e.. it allows access to the sampling units. For a multistage survey, a sampling frame should exist for each stage of sampling. Examples: I In BRFSS, the sampling frame is a list of telephone numbers (actually 2 lists, landline and cell). I for the DHS, the sampling frame is often derived from the census and corresponds to a list of enumeration areas (EAs); within each EA, there should be a list of households; I In NHANES the sampling frame is counties. 15 / 119 Selection bias I Selection bias occurs when some population units are sampled at a different rate than intended by the investigator. I Undercoverage can lead to selection bias, e.g., BRFSS is a telephone survey; started in 1984 at which time many households did not have landline telephones, and so such people are not a random sample of the target population (over 18 years of age). I Overcoverage includes population units in the sampling frame that are not in the target population, e.g., desire over-18 year olds by phone, but younger individuals are included. I Multiplicity in lists, e.g., households with more than one phone have a greater probability of being selected. I Non-response frequently leads to selection bias since non-responders often differ from responders. It is better to have a small survey with a high response rate, than a large survey with a low response rate. I Surveys in which the participants volunteer (e.g., internet polls) are fraught with selection bias. 16 / 119 Common sampling designs I Simple random sampling: Select each individual with probability πk = n=N. I Stratified random sampling: Use information on each individual in the population to define strata h, and then sample nh units independently within each stratum. I Probability-proportional-to-size sampling: Given a variable related to the size of the sampling unit, Zk , on each unit in the population, sample with probabilities πk / Zk . I Cluster sampling: All units in the population are aggregated into larger units called clusters, known as primary sampling units (PSUs), and clusters are sampled initially, with units within clusters then being sampled. I Multistage sampling: Stratified cluster sampling, with multiple levels of clustering. 17 / 119 Measurement error Measurement error reflects inaccurate responses. Multitude of reasons; people: I lie, I do not understand the question, I forget, I respond how they think the interviewer would like them to respond.
Details
-
File Typepdf
-
Upload Time-
-
Content LanguagesEnglish
-
Upload UserAnonymous/Not logged-in
-
File Pages121 Page
-
File Size-