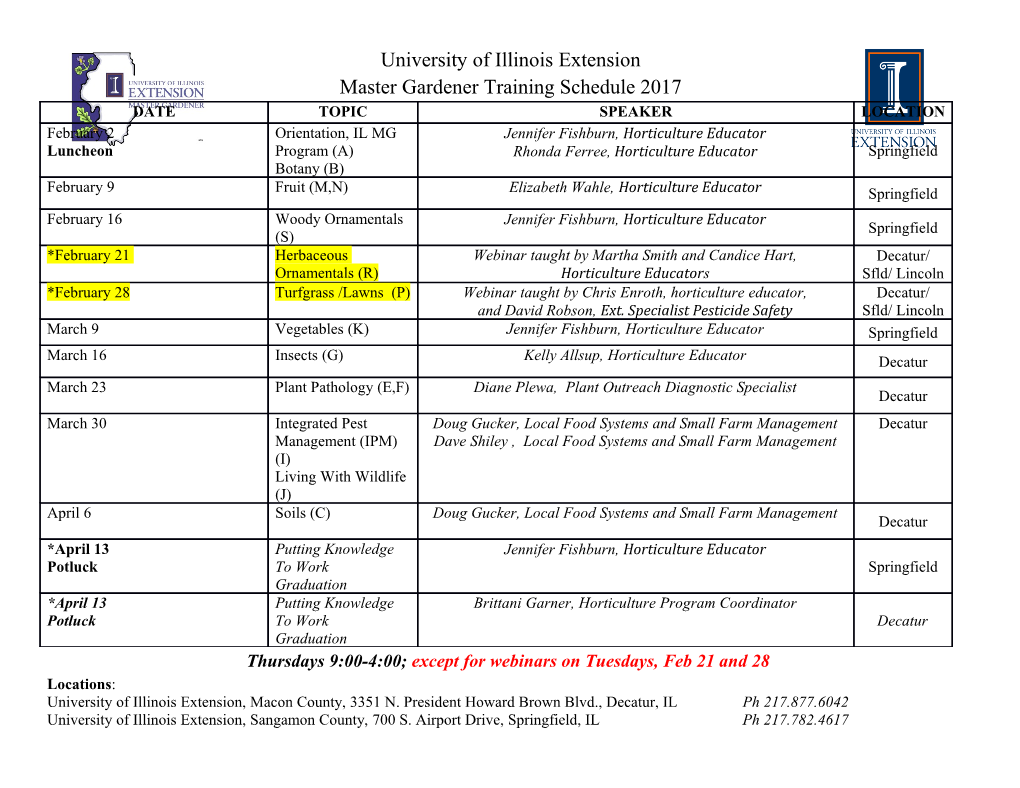
投稿類別:英文寫作 篇名: Data Science and Analysis are Changing the Baseball 作者: 林俊沂。私立僑泰高中。高二 1 班 指導老師: 胡雯俐老師 Data Science and Analysis are Changing the Baseball I. Introduction 1. Motivation Whoever relishes baseball would spend valuable time paying highly constant attention to it. I am no exception. Reading articles or watching programs like MLB almost occupy my free time. I notice that understanding the statistics about baseball is essential because statistics is the most objective ways to define player’s capability. Although baseball statistics sometimes takes a lot of time to grasp, it is actually fun to strengthen the baseball knowledge and to acquaint the influence on players with data science. Therefore, I decide to learn more about baseball statistics and try to show its phenomena and effects through this research. 2. Purpose This research aims at the revolution of the baseball statistics with its origin and the use in the few years. I also sort out some opposite of baseball statistics to distinguish two different views of the topic to annotate it deeper. Through two-side arguments, the research aims to present the influence of the baseball statistics and explain it. II. Body 1. Sabermetrics Sabermetrics is a baseball statistics that can make objective analysis of baseball activities, as for the interpretation and evaluation of baseball statistics during baseball games. The term coined by Bill James, is derived from the acronym SABR which stands for the Society for America Baseball Research and is rooted with metrics. 1.1. The early history of Sabermetrics The first baseball statistics way to describe the baseball activity called box scores, developed by Henry Chadwick in 1858. Box scores offer some basic summary statistics for the players and team. Sabermetrics had not been noticed and dismissed by most of the baseball teams and professionals then, because they thought the statistics wouldn’t relate to the overall team of the standings and player’s ability. However, there are some persons still dedicated to showing their research on Sabermetrics like Earnshaw Cook, Bill James, and even players like Davey Johnson especially when he was playing in Baltimore Orioles in 1970s. These statisticians tried to provide some favorite stats like batting average in different views and opinions. Sabermetrics is a new concept in that era by telling everyone that a good measure determined by how well the players help 1 Data Science and Analysis are Changing the Baseball their team get or save more runs. 1.2. The measurements of Sabermetrics Bill James and his crew found traditional measurements had had some flaws. For example, batting average ignores other ways like walks, hit-by-pitches. Furthermore, some statisticians, like Tom Tango, created a statistic based on linear weights. This type of stats can measure the more accurate player’s overall in per plate appearance. Take Mike Trout’s performance in 2020 as an example to explain the new measurements of Sabermetrics. Mike Trout had 199 at bats, 56 hits, 35 based on balls (4 intentional bases on balls), 3 hit by pitch, 4 sacrifice flies in 2020. His 1B was 28, 2B was 9, 3B was 2, HR was 17 in 2020. OBP The total number of hits + bases on balls + hit by pitch are divided by at bats (On-Base (AB) + bases on balls (BB) + hit by pitch (HBP) + sacrifice flies.(SF) Percentage) Ex: His OBP was 56+35+3/199+35+3+4≈ 0.390. SLG The total number of bases in all hits is divided by the total numbers of time at (Slugging bat. Ex: Mike Trout had 120 total number of bases in 2020. His SLG was Percentage) 120/199≈ 0.603. OPS OBP+SLG (On-base Plus Ex: Mike Trout OPS was 0.390+0.693=0.993 in 2020. Slugging) WOBA Formula 2020: ((0.699 x NIBB) + (0.728 x HBP) + (0.883 x 1B) + (1.238 x (Weighted on 2B) + (1.558 x 3B) + (1.979x HR) )/ AB+BB-IBB+SF+HBP Base Average) NIBB means Non-intentional bases on balls. Ex: Mike Trout IBB was 4 in 2020, so his NIBB was 31. His wOBA was ((0.699 x 31) + (0.728 x 3) + (0.883 x 28) + (1.238 x 9) + (1.558 x 2) + (1.979x 17)) / 199+35-4+4+3≈0.407 in 2020. Ps. Weighted factors will change annually due to every situation in games. Picture source: FanGraphs wOBA Sabermetrics Library Table 1: the explanation of some of measurements in Sabermetrics Table made by myself; information from FanGraphs Baseball 2 Data Science and Analysis are Changing the Baseball 1.3. The recent use on Sabermetrics Now attached is Sabermetrics with higher mathematics like related rates and quantitative analysis to examine information, stats and strategy for organization and the front office. It not only can define the market value and role of players but also give a function to analyze players whether to release or sign by data with correlation. Prediction is also part of Sabermetrics. Building machine models with code like R code or SQL takes on more calculation and precision when applied to large number of events. These predictions can help teams summarize their variables like opponents’ run scored and estimate their winning rate. Sabermetrics has inspired a lot of people who love baseball and statistics like Nate Silver’s PECOTA. It is a system that helps those who have great interest about Sabermetrics to learn and discuss. Some of the technology like PITCHf/x can record play-by-play data by video cameras. It was adopted by MLB and often used at the postseason for broadcasters to report in the beginning of the 2007 season. Private baseball cage is also a trend for some professional favorites to change and improve their swinging performance and strategy. With the latest electronic device, players can know their launch angle (LA), exit velocity (EV), angular velocity and other subjects in just a second. 2. Events Sabermetrics has a strong influence on modern era of baseball. Here are some events that make Sabermetrics become the main stream of the baseball. 2.1. Moneyball: The art of winning an unfair game Moneyball is a breakout of baseball statistics. It was a story about a team called Athletics (A’s) with sabermetrics to create their team in 2001~2003. Their all-star players like Jason Giambi, Johnny Damon and Jason Isringhausen just left and were signed respectively by Yankees, Red Sox and St. Louis Cardinals at all costs. Athletics was a team constrained budget. They only had a modest payroll of $50 million to recreate their team. Their General Manager Billy Beane then applied research with the revolutionary idea about analysis to choose players who did not have too much expectation in the baseball world. Besides, Billy Beane chose the players with high on-base-percentage (OBP) instead of batting average (BA). He thought on-base-percentage was a nice statistics because outs were the most precious things in his mind. According to his philosophy at that time, he could buy players that his ability about scoring as well as a high-value player with fewer money. Here is an example, about Scott Hatteberg, a catcher with an outstanding OBP in his whole career. I chose his stats 3 Data Science and Analysis are Changing the Baseball (2000~2001) before he joined Athletics (2002) with between a hall of fame player Mike Piazza and all hitters OBP (plate appearance above 250), Mike Piazza had the best stats at catcher at the time in 2000 and in 2001. Players Scott Hatteberg Mike Piazza All hitters average Year (s) (plate appearance above 250) 2000 0.367 0.398 0.345 2001 0.332 0.384 0.332 Table 2: the statistics between the players OBP Table made by myself; information from Baseball Reference As we see, Scott Hatteberg’s OBP was above league average. What’s more, he is only a substitute catcher for Red Sox. Billy Beane only spent 9 thousand dollars signing him. The average salary number in 2002 was about 2 million dollars. Scott Hatteberg handed in a 121 wRC+ record in 2002, which means his scoring runs ability and creativity were 21 percent above league average above. It is crazy because he is a bench-salary player or even lower than any other bench player in the rich team. This motion was not to build or produce the best team. A’s just tried to do their best and to enhance their competitivity. The team wasn’t strong enough to compete with the contenders in the market, so they kept mining players and drafting potential players they had selected based on their novel system and stimulated the players capability, outperformed their value and sold them. This situation related the draft style and ploys the team had decided. Billy Beane preferred mature players more than the young boys, thereby college players over high school players. But A’s shortcoming in the postseason was their dark side and was pointed out by the critical fans and traditional baseball players. Those people disagreed A’s purports and said Moneyball was largely misunderstood and disrespected about baseball. As Billy Beane (2009) said in ESPN, “It’s all about evaluating skills and putting a price tag on them,” Beane told ESPN. “They can choose a fund manager who manages their retirement by gut instinct, or one who chooses by research and analysis. I know which I’d choose.” Moneyball absolutely boosts the sports analytics a lot. We all agree that most of the team will use the analytics eventually, but Moneyball just speeds up the process.
Details
-
File Typepdf
-
Upload Time-
-
Content LanguagesEnglish
-
Upload UserAnonymous/Not logged-in
-
File Pages11 Page
-
File Size-