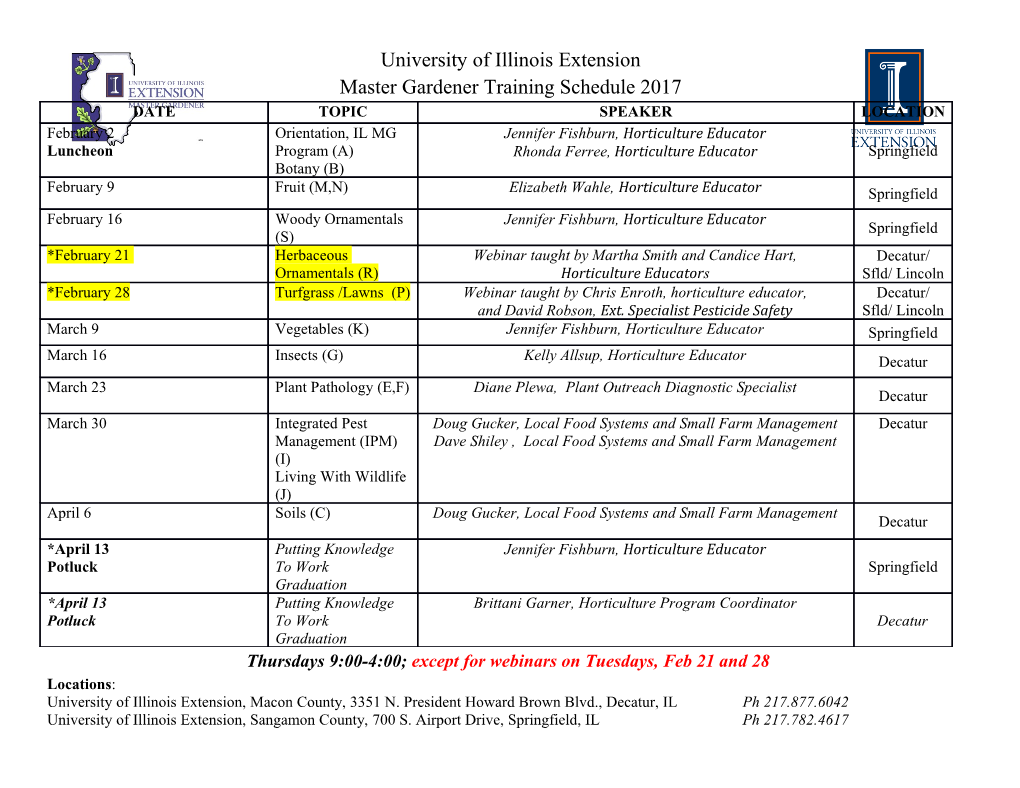
Update on International HPC Activities (mostly Asia) Input from: Erich Strohmaier, Patrick Naullieu (LBNL) Satoshi Matsuoka (TiTech) Haohuan Fu (Wuxi) And many conversations in Singapore John Shalf Lawrence Berkeley National Laboratory ASCAC, April 18, 2017 Performance of Countries 100,000 US /s] 10,000 EU Tflop Japan 1,000 China 100 10 1 Total P erformanc e [ 0 2000 2002 2004 2006 2008 2010 2012 2014 2016 Share of Top500 Entries Per Country Historical Share Current Share (averaged over liftetime of list) (November 2016 list) Korea, Poland Italy Others Italy Poland Italy China South United United 1% 1% 11% 1% 1% 1% Canada 1% others KingdomKingdom Others United States 1% 12% 3% France 3%France 11% ChinaChina Germany China 4% France 4% Japan 3434%% Japan 5% United 4% Japan United 6% States 6% France Kingdom Germany 6% 52% Germany United Kingdom 6% United Germany 6% United StatesStates Poland 8% Japan 34%34% Italy 10% Producers of HPC Equipment 500 India 450 400 Taiwan 350 Australia 300 250 Russia 200 China 150 100 Europe 50 0 Japan 1993 1995 1997 1999 2001 2003 2005 2007 2009 2011 2013 2015 USA Vendors / Performance Share 2007 Now HPE, 66, 10% HPE SGI, 40, 6% SGI Others, Lenovo 136, 20% Lenovo, 64, 10% Cray Inc. NUDT, 39, 6% Sugon FuJitsu, 38, 6% Cray Inc., Dell, 16, 2% 143, 21% IBM Inspur, Bull, Atos 9, 1% Huawei, 9, 1% Bull, Atos, 24, 4% Huawei IBM, 63, 9% Sugon, 25, 4% Sum of Pflop/s, % of whole list by vendor NSA-DOE Technical Meeting on High Performance Computing December 1, 2017 Top Level Conclusions 1. National security requires the best computing available, and loss of leadership in HPC will severely compromise our national security. 2. HPC leadership has important economic benefits because of HPC’s role as an enabling technology 3. Leadership positions, once lost, are expensive to regain Meeting participants expressed significant concern that – absent aggressive action by the U.S. – the U.S. will lose leadership and not control its own future in HPC v It is critical to lead the exploration and development of innovative computing architectures that will unleash the creativity of the HPC community v Workforce development is a major concern in HPC and a priority for supporting NSCI Objectives #4 and #5 v NSCI leadership develop more efficient contracting regulations to improve the public-private partnership in HPC science and technology development. PERFORMANCE AND ALGORITHMS RESEARCH GROUP China Update Aggressive Growth of China Chip Fabs v Current 28nm domestic capability in Shenzhen, Nanjing and other regions v Broke ground on 14nm fab for 2018 near Shanghai § Annual spending on fab equipment in China above $10B by 2018 § Feb 2017: China is expected to be the top spending region for fab equipment spending by 2019, overtaking South Korea and Taiwan. v Foxconn offered 3T Yen ($30B) bid for Toshiba fabs § Amazon & Google + SK Hynix & Western Digital consortium bidding § Apple bidding to own 20% stake in Fujitsu fab § TSMC withdrew its bid § Selection by June 8 Fab Construction in China Source Semiconductor Equipment and Materials International (SEMI) 9 Fab Construction in China Source Semiconductor Equipment and Materials International (SEMI) 10 Fab Construction in China Source Semiconductor Equipment and Materials International (SEMI) 11 Fab Construction in China Source Semiconductor Equipment and Materials International (SEMI) 12 China 2017 Prototype System Bake-off v China plans to have three prototypes for candidate exascale systems delivered in 2017 [Xinhua: Jan 19, 2017] v Scale-up winner(s) to exascale in 2020 (my guesses below) v Other: Longsoon (unlikely), Silicon Cube (no), Thatic AMD (Tianjin/Sugon?) Wuxi/Sunway NSC/Phytium NUDT/Tianhe2a? v Heterogeneous v Homogeneous v Attached Accelerator manycore/accel Manycore v ARMv8 PCIe attached v 4*8x8 CPEs (light) v 64-core ARMv8 self- accelerator (ISC16) + 4 MPE (heavy) hosted v <strategy may change> Core ... Core Core ... Core Core Core ... Acc. Memory ... ... ... Network-on-Chip Network-on-Chip Network-on-Chip ... ... ... Memory Memory Memory Core Core Acc. ... Core ... Core Core ... Core Core Core Memory 13 152 J. Comput. Sci. & Technol., Jan. 2015, Vol.30, No.1 4 Implementation and Performance Evalua- The prototype includes 256 CPEs, four MPEs and four tion MCs, as shown in Fig.7. The FPGA prototype system uses a total of 352 To validate DFMC, we implemented a full chip Altera EP3C120, 21 Xilinx 5VLX330 and one Xilinx RTL design and built a prototype system with FPGA. 5VLXT220. The frequency of the prototype system is The performance of cooperative computing techniques 2.6 MHz. Table 5 lists the components and functions. in the prototype system was evaluated. Furthermore, Although there are many cross-board signals, we several typical applications were mapped to the DFMC balance all of the stages related to cross-board and en- architecture for performance analysis. sure the FPGA prototype system is equal to the RTL design at the cycle level. Then, the foremost reason 4.1 Full Chip RTL that the simulation is inaccurate is the main memory frequency. Compared with the target RTL design, the The RTL of DFMC is designed in-house; thus we ratio of CPE frequency to MC frequency in the proto- can easily optimize the microarchitecture, extend the type is quite different, which results in simulation de- functionality, and balance the performance and the viation. To ensure accuracy, the prototype system uses power-usage. Clock gate and fault tolerance technology the performance calibration techniques. FPGA proto- are also used in this design. For the future test chip, types have many performance adjusters and counters, we finished the physical design intended for fabrication and we have an FPGA adjustment benchmark that in- in 40 nm technology. cludes more than one hundred short programs espe- The parameters of DFMC are compared with those cially for memory systems. We define the deviation ra- of an Intel Xeon CPU and an NVIDIA GPU as shown in tio as the ratio of a program’s execution time on RTL Table 4. These processors are different in architectures, to its execution time on FPGA. Then, we can adjust but under the similar CMOS technology process. Be- the latency, bandwidth, and scheduling in the FPGA cause of the balance design of power and performance in prototype to find the minimum average deviation ratio CPEs, DFMC achieves the best peak performance and for the benchmark. The performance counters can in- the ratio of computation to power consumption. How- dicate which adjustment is more important. The test ever, the ratio of memory bandwidth to computation of shows that the performance accuracy of the prototype DFMC is the worst. In this paper, DFMC combines a system is up to 95% in the benchmark thanks to the series of cooperative computing techniques to solve this calibration. problem. 4.3 Software Layer 4.2 Prototype System In this paper, the programs running on DFMC use The applications and tests run slowly in a software the accelerated model. We designed a library-based environment, thereby we implemented a full chip pro- programming approach to ease the task of utilizing totype system with FPGA for acceleration. DFMC. The library supports programming interfaces The FPGA prototype system adopts a modular for thread management, data stream transfer, register structure, which consists of MPE cards, CPE cards, level communication and synchronization. Program- Sunwaya Node PCIe card, Architecture an MC card, an NoC card, and so on. mers can use these interfaces to explicitly control the (refresher course) Table 4. Parameters of DFMC/Xeon/GPGPU SW26010: Sunway 260-CoreDFMC Processor (40 nm) Intel! Xeon! 5680 (32 nm) NVIDIA Fermi M2090 (40 nm) Architecture Memory 4 CPE clustersMemory (256 CPEs) 6 cores 512 CUDA cores 4 MPEs, 4 MCs iMC Core Group 0 Core Group 1 iMC Memory Level 8*8NoC CPE Mesh Mesh Ring topology – PPU PPU Computing Row On-chip memory 32 KB in each CPE 256=8 MB 12 MB cache 1 024 KB share memory/L1 cache Core Communication × 8*8 CPE 8*8 CPE Bus MPE MPE 768 KB L2 cache Mesh Frequency 1GHz Mesh 3.33 GHz 1.3 GHz LDM Level Registers Computing ability 1000 GFLOPS DP 80 GFLOPS DP 665.6 GFLOPS DP NoC Data Transfer Memory bandwidth 102.4 GB/s DDR3 32 GB/s DDR3 177.6 GB/s GDDR5 LDM Network Chip area 400 mm2@40 nm 240 mm2 @32 nm 520 mm2@40 nm 8*8 CPE 8*8 CPE MPE ∼ MPE Power Mesh 200 W Mesh Register Level 130 W 250 W Transfer Agent (TA) ∼ PPU PPU Control Column iMC iMC Network Communication Bus Core Group 2 Core Group 3 Computing Level That is 64k per CPEMemory LDM@28nmMemory (not 64k for the entire CPE mesh) Fang Zheng (Wuxi) 212 instructions Alpha-like ISA Jan 2015 240mm^2 chip area @ 28nm (cacti) J. Comp. & Sci. Tech 14 Phytium Mars Architecture Panel Architecture Xiaomi Xiaomi L2cache } Eight Xiaomi Cores Xiaomi Xiaomi } Compatible design with ARMv8 arch license DCU } Both AArch32 and AArch64 modes } EL0~EL3 supported Routing Cell } ASIMD-128 supported DCU } Adv. hybrid Branch Prediction Xiaomi Xiaomi } 4 fetch/4 decode/4 dispatch Out-of-Order L2cache Xiaomi Xiaomi superscalar pipeline 6000μm } Cache Hierarchy } Separated L1 ICache and L1 Dcache } Shared L2 cache, totally 4MB } Directory-based cache coherency 10600μm maintenance } Directory Control Unit (DCU) } Routing Cell 7 Phytium Technology Co., Ltd 15 Phytium Mars Architecture Cache & Memory Chip } L3 cache Mars Interface } 16MB Data Array } 2MB Data ECC L3 L3 } DDR bandwidth Bank0 Bank1 } 2 x DDR3-800:25.6GB/s D D Mem Mem D D } Proprietary interface between Mars & Ctrl0 Ctrl1 CMC R R } Parallel interface
Details
-
File Typepdf
-
Upload Time-
-
Content LanguagesEnglish
-
Upload UserAnonymous/Not logged-in
-
File Pages41 Page
-
File Size-